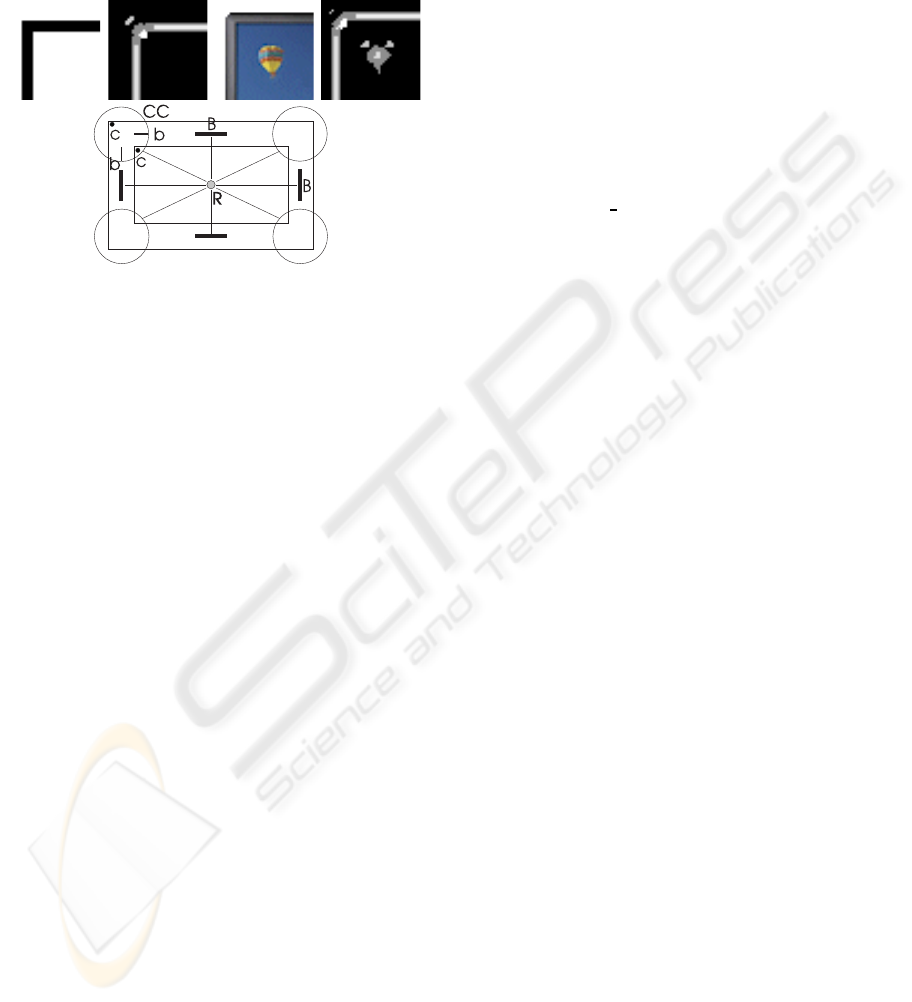
fast selection of possible object categories in memory
(Bar M., et al., 2006). This is a purely bottom-up and
data-parallel process for bootstrapping the serial ob-
ject categorization and recognition processes which
are controlled by top-down attention. Low-level ge-
Figure 8: Low-level geometry (top) and example of mid-
and high-level geometry groupings (bottom); see text for
details.
ometry is difficult to visualise, because it consists of a
large number of spatial maps, in this paper limited to
22 but there could be more, one for each spatial con-
figuration. Figure 8 (top), shows detail images with a
few configurations coded by different levels of gray,
i.e., corners, bars and blobs. The input was an ideal
rectangle with two sharp corners (left) and a com-
puter monitor with a sharp inner corner but rounded
outer one. Despite the outer corner being rounded,
evidence for a corner has been detected at two pixel
positions. These results were obtained on the basis of
colour conspicuity, but later texture and colour infor-
mation should be combined, and low-level geometry
should be used to construct mid- and high-levelgeom-
etry. The latter idea is illustrated in Fig. 8 (bottom):
at low level corners (c) and bars (b) are detected. At
mid level, these can be grouped into a complex corner
(CC), and at high level the CCs, together with linking
bars B, into a rectangle R. Such an R structure is typ-
ical for man-made objects, for example a computer
monitor or a photo frame. This example of high-level
geometry is perhaps the last level below semantic pro-
cessing: a computer monitor in combination with one
or more photo frames is an indication for global scene
gist: our office. In any case, the large number of fea-
tures at the lowest level (64 Gabor channels) is re-
duced to the number of spatial configurations at low-
level geometry, here 22. Groupings at mid level (e.g.,
complex corner CC) may lead to less configurations,
but at high level (e.g., rectangle R) the number of con-
figurations will increase again, because many elemen-
tary shapes must be represented. On the other hand,
the precise localisation of configurations which is re-
quired at low level is not necessary at higher levels;
for example, grouping cells for complex corners CC
may be located somewhere near the centres of the cir-
cles in Fig. 8 (bottom), as long as their dendritic fields
are big enough to receive input from two corner and
two bar cells. These aspects are subject to further re-
search.
ACKNOWLEDGEMENTS
Research supported by the Portuguese Foundation for
Science and Technology (FCT), through the pluri-
annual funding of the Inst. for Systems and Robotics
through the POS Conhecimento Program (includes
FEDER funds), and by the FCT project SmartVision:
active vision for the blind (PTDC/EIA/73633/2006).
REFERENCES
Bar M., et al. (2006). Top-down facilitation of visual recog-
nition. PNAS, 103(2):449–454.
du Buf, J. (2007). Improved grating and bar cell models in
cortical area V1 and texture coding. Image and Vision
Computing, 25(6):873–882.
Elazary, L. and Itti, L. (2008). Interesting objects are visu-
ally salient. Journal of Vision, 8(3):1–15.
Finlayson, G., Schiele, B., and Crowley, J. (1998). Compre-
hensive colour image normalization. Proc. 5th Europ.
Conf. Comp. Vision, I:475–490.
Hubel, D. (1995). Eye, brain and vision. Scientific Ameri-
can Library.
Itti, L. and Koch, C. (2001). Computational modeling
of visual attention. Nature Reviews: Neuroscience,
2(3):194–203.
Niebur, E. and Koch, C. (1996). Control of selective vi-
sual attention: Modeling the ‘where’ pathway. Neural
Information Processing Systems, 8:802–808.
Rensink, R. (2000). The dynamic representation of scenes.
Visual Cogn., 7(1-3):17–42.
Rodrigues, J. and du Buf, J. (2004). Visual cortex fron-
tend: integrating lines, edges, keypoints and disparity.
Proc. Int. Conf. Image Anal. Recogn., Springer LNCS
3211(1):664–671.
Rodrigues, J. and du Buf, J. (2006). Multi-scale keypoints
in V1 and beyond: object segregation, scale selection,
saliency maps and face detection. BioSystems, 2:75–
90.
Rodrigues, J. and du Buf, J. (2007). Invariant multi-
scale object categorisation and recognition. Proc.
3rd Iberian Conf. on Patt. Recogn. and Image Anal.,
Springer LNCS 4477:459–466.
van de Weijer, J., Gevers, T., and Bagdanov, A. D. (2006).
Boosting color saliency in image feature detection.
IEEE Tr. PAMI, 28(1):150–156.
VISAPP 2009 - International Conference on Computer Vision Theory and Applications
272