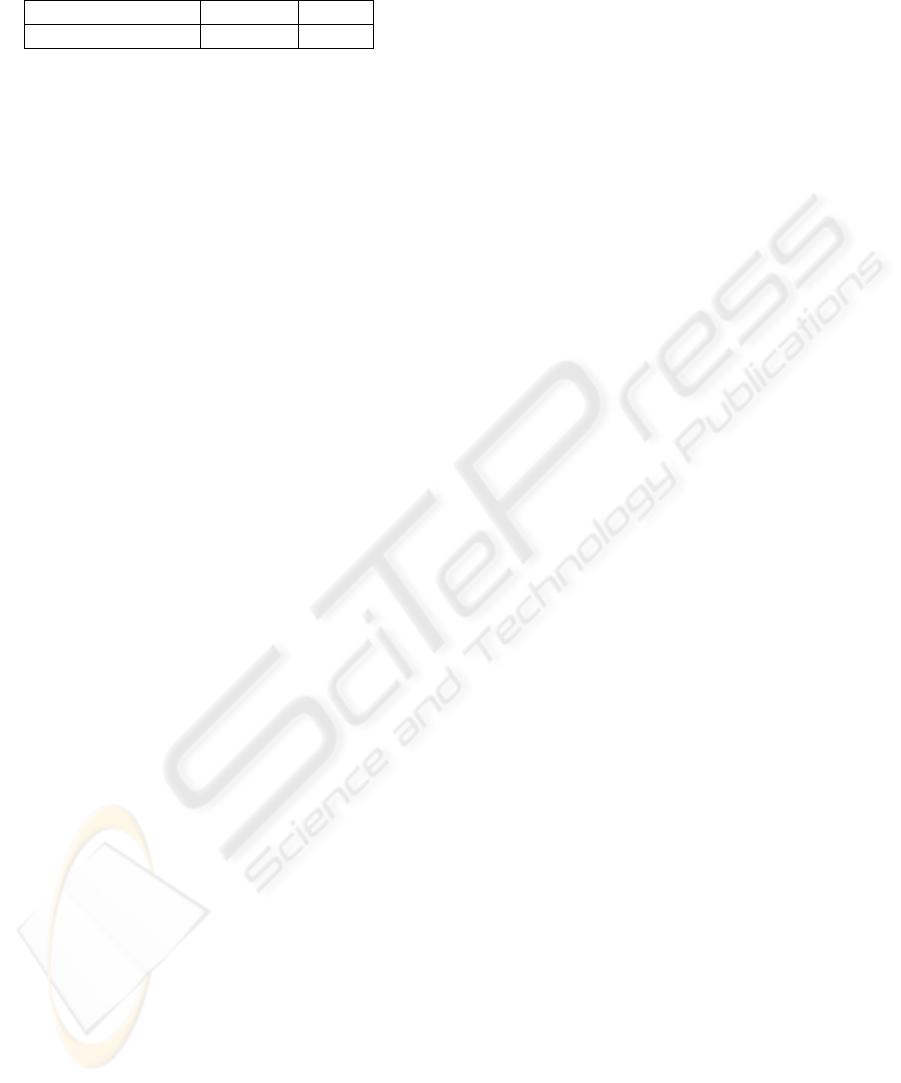
Table 2: The performance using multi-modality integration
under both Trecvid and medical dataset.
Precision Recall
489,655 keyframes 94.6% 96.4%
CT images. The images are all grayscale, 512*512
pixels. The experiments showed that the accuracy of
this algorithm has been greatly improved (Precision
94.6% Recall 96.4%. (See Table 2).
6 CONCLUSIONS AND FUTURE
WORK
In this paper, a new approach for medical image and
video retrieval system is presented. A new method
based on keyframe matching and partial sequence
alignment is proposed. An extensive evaluation of
different methods for multi-modality automatic cate-
gorization of medical images is presented. A new fea-
ture space expression named artificial potential field
based feature extraction method is discussed. The
experimental results show that it is feasible and per-
forms well. The average performance and precision
is pretty promising. It is shown that the addressed ap-
proaches are promising to offer new possibilities for
content-based access to medical images as an accu-
racy of 94% within the thirty best matches is sufficient
for most applications. Content-based image retrieval
systems that are no longer limited to a special context
are becoming possible. Our future work will focus
on the dataset collection and the multi-modality data
mining.
ACKNOWLEDGEMENTS
This research was supported in part by the National
Natural Science Foundation of China under the grant
No. 60621062 and 60605003, and the National
Key Foundation R&D Projects under the grant No.
2003CB317007 and 2004CB318108 and China Post-
doctoral Science Foundation 20080430422.
REFERENCES
Bucci, G., S., C., and Domicinis, R. D. (1996). Integrat-
ing content-based retrieval in a medical image refer-
ence databasez. Computerized Medical Imaging and
Graphics, 20(4):231–241.
Cai, W., Feng, D., and Fulton, R. (2001). Content-based re-
trieval of dynamic pet functional images. IEEE Trans.
Inform. Tech. Biomed, 4(2):152–158.
Cord, M., Fournier, J., and Philipp-Foliguet, S. (2003). Ex-
ploration and search-by-similarity in cbir. In Proc. of
SIBGRAPI 03, Sao Carlos, Brsil.
Khatib, O. and Maitre, J. L. (1978). Dynamic control
of. manipulators operating in a complex environment.
Proceedings Third International CISM-IFToMM Sym-
posium,September 1978, pages 267–282.
Kim, C. and Vasudev, B. (2005). Spatiotemporal sequence
matching for efficient video copy detection. IEEE
Trans. on Circuits and Systems for Video Technology,
15(1):127–132.
Liu, Y., Collins, R., and Rothfus, W. (2001). Robust mid-
sagittal plane extraction from normal and pathologi-
cal 3d neuroradiology images. IEEE Transactions on
Medical Imaging, 20(3):175–192.
Muller, H., Michoux, N., Bandon, D., and Geissbuhler, A.
(2004). A review of content-based image retrieval sys-
tems in medical applications–clinical benefits and fu-
ture directions. International Journal of Medical In-
formatics, 73(1):1–23.
Orphanoudakis, S., Chornaki, C., and Kostomanolakis, S.
(1994). I2c-a system for the indexing, storage and re-
trieval of medical images by content. Med Informat-
ics, 19(2):109–122.
Poggio, T. and Bizzi, E. (2004). Generalization in vision
and motor control. NATURE, 296:768–774.
Smeulders, A. W. M., Worring, M., Santini, S., Gupta, A.,
and Jain, R. (2000). Contentbased image retrieval at
the end of the early years. IEEE Trans Pattern Anal
Machine Intell, 22(12):1349–1380.
Squire, D. M., Muller, A. W., Muller, H., and
Raki, J. (1999). Content-based query of im-
age databases-inspirations from text retrieval-inverted
files, frequency-based weights and relevance feed-
back. Proceeding Scandinavian Conference on Image
Analysis, Kangerlussuaq, Greenland, pages 143–149.
Tagare, H., Jaffe, C., and Duncan, J. (1997). Medical image
databases - a content-based retrieval approach. Jour-
nal of the American Medical Informatics Association,
4:184–198.
Taylor, D. M., Tillery, S. I. H., and Schwartz1, A. B. (2002).
Direct cortical control of 3d neuroprosthetic devices.
SCIENCE, 296:1829–1832.
IMAGAPP 2009 - International Conference on Imaging Theory and Applications
86