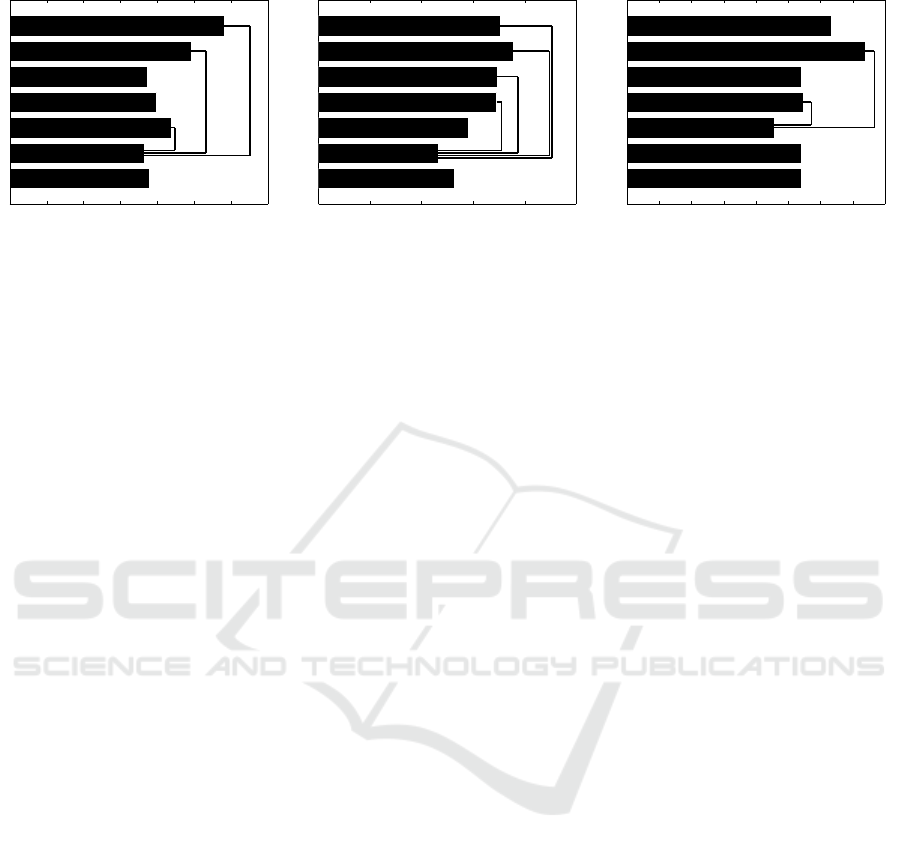
(a) raw pixel intensities (12×12).
8bit
7bit
6bit
5bit
4bit
3bit
2bit
median of test errors
0 0.05 0.1 0.15 0.2 0.25 0.3
0.35
(b) SGFs.
8bit
7bit
6bit
5bit
4bit
3bit
2bit
median of test errors
0 0.05 0.1 0.15 0.2
0.25
(c) SPECs.
8bit
7bit
6bit
5bit
4bit
3bit
2bit
median of test errors
0 0.02 0.04 0.06 0.08 0.1 0.12 0.14
0.16
Figure 9: Medians of test errors. Black lines indicate a significant difference (p < 0.05) between two methods. Only significant
differences of the best depth level to all others are considered in the plot.
of the novel SPEC features are comparable to those
obtained by manual inspection.
REFERENCES
Boser, B., Guyon, I., and Vapnik, V. (1992). A training al-
gorithm for optimal margin classifiers. In Haussler,
D., editor, Proc. of the 5th Annual ACM Workshop
on Computational Learning Theory, pages 144–152.
ACM Press.
Chen, Y. Q., Nixon, M. S., and Thomas, D. W. (1995). Sta-
tistical geometrical features for texture classification.
Pattern Recognition, 28(4):537–552.
Chiu, S. and Perng, M. (2007). Reflection-area-based fea-
ture descriptor for solder joint inspection. Machine
Vision and Applications, 18(2):95–106.
Cortes, C. and Vapnik, V. (1995). Support-vector networks.
Machine Learning, 20(3):273–297.
Driels, M. and Lee, C. (1988). Feature selection for auto-
matic visual inspection of solder joints. The Int. Jour-
nal of Advanced Manufacturing Technology, 3:3–32.
Fukunaga, K. (1972). Introduction to Statistical Pattern
Recognition. Academic Press.
Kim, J. and Cho, H. (1995). Neural network-based inspec-
tion of solder joints using a circular illumination. Im-
age and Vision Computing, 13(6):479–490.
Ko, K. and Cho, H. (2000). Solder joints inspection using
a neural network and fuzzy rule-based classification
method. IEEE Transactions on Electronics Packaging
Manufacturing, 23(2):93–103.
Labusch, K., Timm, F., and Martinetz, T. (2008). Sim-
ple incremental one-class support vector classifica-
tion. In Rigoll, G., editor, Proc. of the 30th German
Pattern Recognition Symposium DAGM, volume 5096
of Lecture Notes in Computer Science, pages 21–30.
Springer.
Ong, T., Samad, Z., and Ratnam, M. (2008). Solder joint
inspection with multi-angle imaging and an artificial
neural network. The Int. Journal of Advanced Manu-
facturing Technology, 38(5–6):455–462.
Poechmueller, W., Glesner, M., Listl, L., and Mengel, P.
(1991). Automatic classification of solder joint im-
ages. In Proc. of the Int. Joint Conf. on Neural Net-
works, volume 2, pages 933–940. IEEE Computer So-
ciety Press.
Russ, J. C. (2007). The Image Processing Handbook. CRC
Press.
Sch
¨
olkopf, B., Platt, J. C., Shawe-Taylor, J., Smola, A. J.,
and Williamson, R. C. (2001). Estimating the support
of a high-dimensional distribution. Neural Computa-
tion, 13(7):1443–1471.
Stone, M. (1974). Cross-validatory choice and assessment
of statistical predictions. Journal of the Royal Statis-
tics Society, 36:111–147.
Tax, D. M. J. and Duin, R. P. W. (2004). Support vector data
description. Machine Learning, 54(1):45–66.
Timm, F., Klement, S., and Martinetz, T. (2008). Fast model
selection for maxminover-based training of support
vector machines. In Proc. of the 19th Int. Conf. on
Pattern Recognition, Florida, USA. IEEE Computer
Society Press. to appear.
Vapnik, V. (1995). The Nature of Statistical Learning The-
ory. Springer Verlag, New York.
Walker, R. and Jackway, P. T. (1996). Statistical geometric
features: Extensions for cytological texture analysis.
In Proc. of the 13th Int. Conf. on Pattern Recognition,
pages 790–794. IEEE Computer Society Press.
WELDING INSPECTION USING NOVEL SPECULARITY FEATURES AND A ONE-CLASS SVM
153