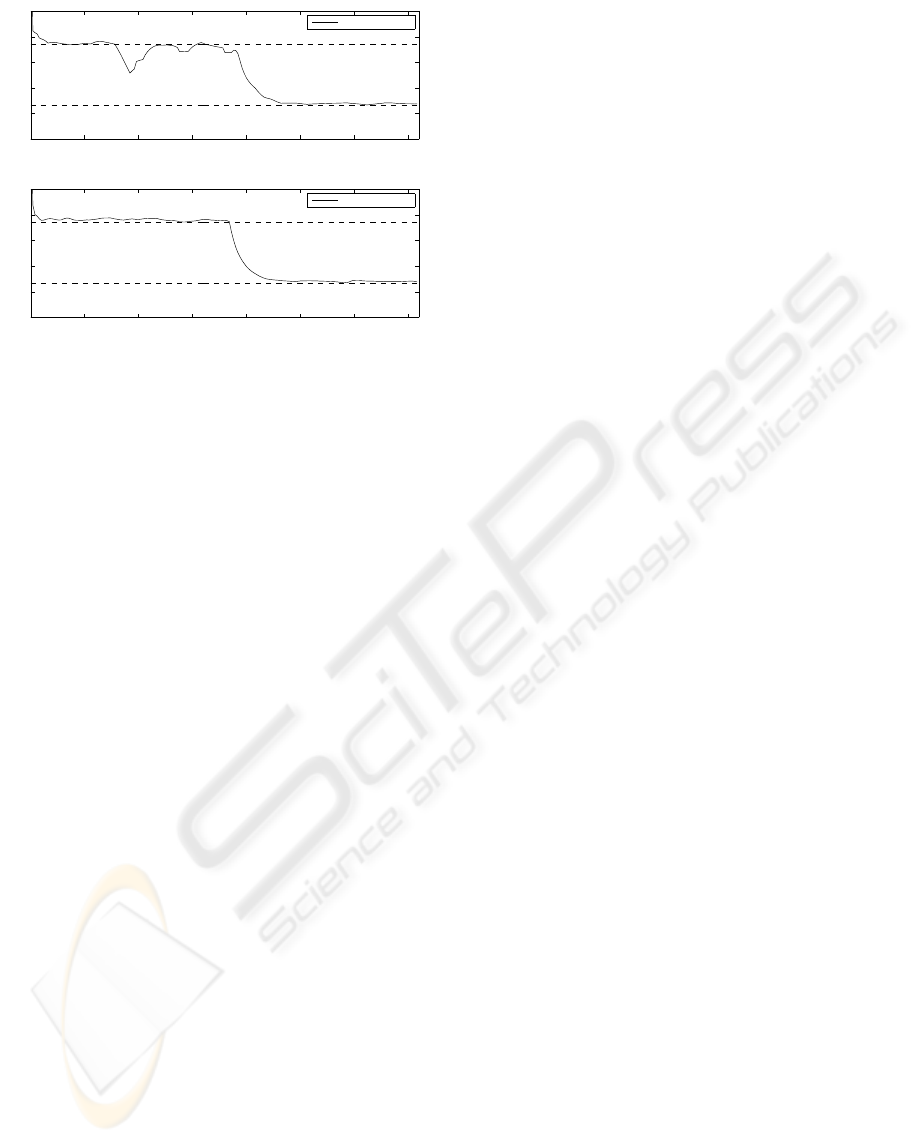
0 50 100 150 200 250 300 350
0.7
0.8
0.9
1
1.1
Time (sec)
Period T (sec)
Subject 1 (cycling)
0 50 100 150 200 250 300 350
0.7
0.8
0.9
1
1.1
Time (sec)
Period T (sec)
Subject 2 (cycling)
Figure 2: Estimation of cycling pedal rate using a triax-
ial accelerometer (at 2 exercise rates: 1.07 and 0.83 secs).
(Top) Subject 1, (Bottom) Subject 2.
4 CONCLUSIONS
An algorithm for the estimation of exercise rate from
triaxial accelerometer measurements was proposed in
this paper. The proposed algorithm is universal re-
gardless of the mode of exercise, and it has been ex-
perimentally verified in determining the exercise rates
of walking and cycling. The algorithm can readily be
applied to the monitoring of rehabilitation exercise for
the cardiac patients, and training exercise for the ath-
letics. Also, it will be useful in monitoring activities
of the elderly and the obese.
ACKNOWLEDGEMENTS
This work was supported by the Australian Research
Council.
REFERENCES
Asano, M., Tanabe, Y., Watanabe, K., Genno, H., Nemoto,
K., Nose, H., and Isawa, M. (2005). Development
of an exercise meter using triaxial acceleration data.
In Proceedings of Annual International Conference of
the IEEE Engineering in Medicine and Biology Soci-
ety, pages 3731–3734.
Chen, K. Y. and Sun, M. (1997). Improving energy ex-
penditure estimation by using a triaxial accelerometer.
Journal of Applied Physiology, 83:2112–2122.
de Cheveigne, A. and Kawahara, H. (2002). YIN, a fun-
damental frequency estimator for speech and mu-
sic. Journal of the Acoustical Society of America,
111(4):1917 – 30.
Hall, D. L. and Llinas, J. (1997). An introduction to multi-
sensor data fusion. Proceedings of the IEEE, 85(1):6–
23.
Hess, W. (1983). Pitch determination of speech signals.
Springer-Verlag, Berlin.
Karantonis, D. M., Narayanan, M. R., Mathie, M., Lovell,
N. H., and Celler, B. G. (2006). Implementa-
tion of a real-time human movement classifier us-
ing a triaxial accelerometer for ambulatory monitor-
ing. IEEE Transactions on Information Technology in
Biomedicine, 10(1):156–167.
Kim, D. and Kim, H. (2008). Estimation of activity en-
ergy expenditure based on activity classification using
multi-site triaxial accelerometry. Electronics Letters,
44(4):266– 267.
Parker, P. J. and Anderson, B. D. O. (1990). Frequency
tracking of nonsinusoidal periodic signals in noise.
Signal Processing, 20(2):127–152.
Rabiner, L., Cheng, M., Rosenberg, A., and McGonegal,
C. (1976). A comparative performance study of sev-
eral pitch detection algorithms. IEEE Transactions
on Acoustics, Speech and Signal Processing, ASSP-
24(5):399–418.
Rabiner, L. and Schafer, R. (1978). Digital processing of
speech signals. Prentice-Hall, Englewood Cliffs, NJ.
Ross, M. J., Shaffer, H. L., Cohen, A., Freudberg, R., and
Manley, H. J. (1974). Average magnitude difference
function pitch extractor. IEEE Transactions on Acous-
tics, Speech, and Signal Processing, ASSP-22(5):353
– 362.
Simon, D. (2006). Optimal State Estimation: Kalman, H
∞
,
and nonlinear approaches. John Wiley & Sons, New
Jersey.
Smyth, A. and Wu, M. (2007). Multi-rate Kalman filter-
ing for the data fusion of displacement and accelera-
tion response measurements in dynamic system mon-
itoring. Mechanical Systems and Signal Processing,
21(2):706–723.
Tan, H., Wilson, A. M., and Lowe, J. (2008). Measure-
ment of stride parameters using a wearable GPS and
inertial measurement unit. Journal of Biomechanics,
41(7):1398–1406.
Tanaka, C., Tanaka, S., Kawahara, J., and Midorikawa,
T. (2007). Triaxial accelerometry for assessment
of physical activity in young children. Obesity,
15(5):1233–1241.
BIOSIGNALS 2009 - International Conference on Bio-inspired Systems and Signal Processing
538