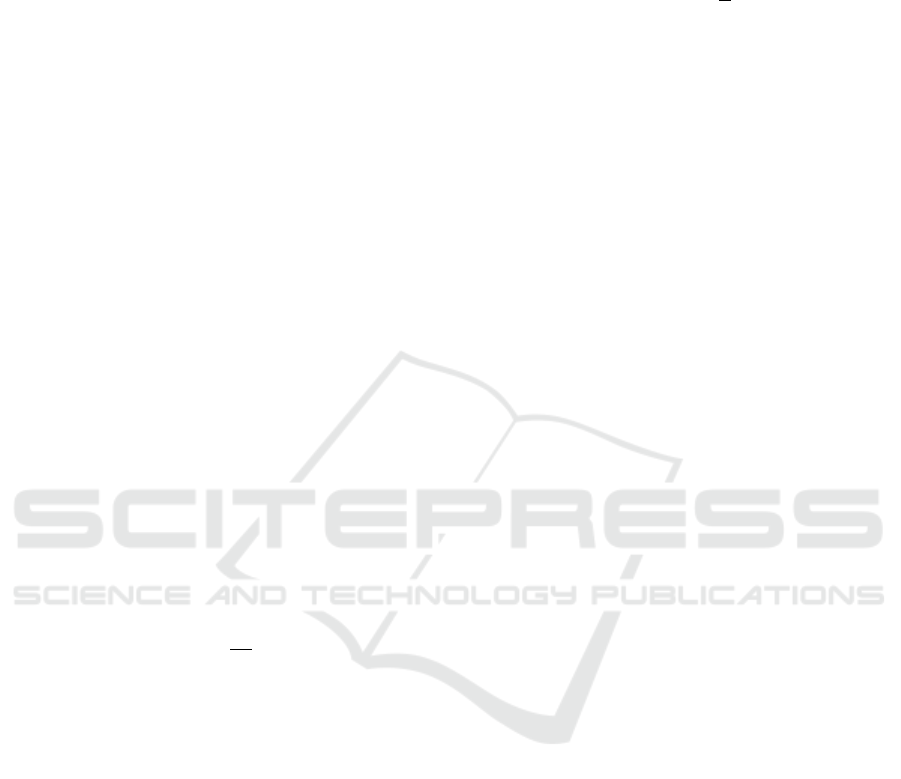
threshold value being determined based on the mea-
sure that best separate the histogram peaks. However,
not necessarily all features of interest form promi-
nent peaks. In a general way, global approaches yield
good results only when there is a good separation
between foreground and background, a limitation in
document images with degradation problems like in-
homogeneous backgrounds, smears and strains.
A well-known general purpose histogram-based
global thresholding approach is the Otsu’s algo-
rithm (Otsu, 1979). Briefly, it selects as an opti-
mal threshold the one which minimizes the ratio be-
tween the “between-class” and the total variance. The
between-class variance is defined as the deviation
of the mean values for each considered class (back-
ground and object) from the overall mean of the pix-
els.
On the other hand, local thresholding approaches
provide an adaptive solution where the threshold
value is determined pixelwise and depends on re-
gional image characteristics. Due to the computa-
tional cost, it is important to define efficient transfor-
mations to be applied locally. We have compared our
approach against some of these methods, described
below.
The moving averages method considers a thresh-
old based on the mean gray level of the last n pixels,
and it is designed for images containing text. The im-
age can be treated as a one-dimensional stream of pix-
els and the average can either be computed exactly or
estimated via (Parker, 1996):
M
i+1
= M
i
−
M
i
n
+ g
i+1
(1)
where M
i+1
is the estimate of the moving average for
pixel i + 1 having gray level g
i+1
and M
i
is the previ-
ous moving average (i.e. for pixel i). Any pixel less
than a fixed percentage of its moving average is set to
black; otherwise it is set to white.
The Niblack’s algorithm defines a local threshold
based on the mean and standard deviation values cal-
culated over a rectangular window around the pixel
according to the following formula (Niblack, 1986):
T = m + k ∗ s (2)
where m is the mean and s the standard deviation of
the pixels in the window. The variable k determines
how much of the object is retained, and assumes a
value between −1 and 1. As drawbacks, we have the
low thresholding speed, the sensitivity to the size of
the window and the occurrence of noise in the back-
ground.
In order to minimize the background noise in im-
ages with uneven illumination, Sauvola proposed an
extension to Niblack’s algorithm where the threshold
value is computed with the dynamic range of the stan-
dard deviation, R, according to the equation (Sauvola
and Pietikainen, 2000):
T = m ∗
1 + k
s
R
− 1
(3)
where, again, m and s are mean and standard devi-
ation of the window. Here, k takes a positive value
between 0 and 1. To properly determine the R value,
it is necessary to know the document contrast. The
influence of the window size and the threshold speed
still remain a problem.
Gatos et al. (Gatos et al., 2006) proposed a locally
adaptative binarization scheme that can deal with de-
graded document images. The method consists in five
basic steps, starting from a rough estimation of the
foreground, obtained using the Sauvola’s algorithm,
that is improved using local image analysis. More
complete reviews of image thresholding techniques
can be found in (Trier and Jain, 1995) (Gatos et al.,
2006) (Sahoo et al., 1988) (Sezgin and Sankur, 2004).
3 SCALE-SPACE TOGGLE
OPERATOR FOR IMAGE
SIMPLIFICATION
Multiscale approaches have been largely consid-
ered, playing an important role when designing auto-
matic methods to cope with real world measurements
where, in most of the cases, there is no prior informa-
tion about which would be the appropriate scale.
Here, we use an operator based on the scale-space
approach (Witkin, 1984), in which the inherent mul-
tiscale nature of real-world images is represented by
embedding the original signal into a family of simpli-
fied signals, created by successively removing image
structures across scales while preserving the essential
features. Since the representation of an interest sig-
nal feature describes a continuous path through the
scales, it is possible to relate information obtained in
different representation levels, a drawback in many
multiscale approaches.
Due to the problems inherent to the linear ap-
proaches (Witkin, 1984), non-linear scale-space oper-
ators based on mathematical morphology have been
frequently used (Bosworth and Acton, 2003). In
this context, scale-spaces are generated by filtering
gray-scale signals with specific combinations of the
scaled erosion and dilation operations, defined as fol-
lows (Jackway and Deriche, 1996).
Definition (Dilation). The dilation of the function
f (x) by the structuring function g
σ
(x), ( f ⊕ g
σ
)(x),
A MULTISCALE OPERATOR FOR DOCUMENT IMAGE BINARIZATION
35