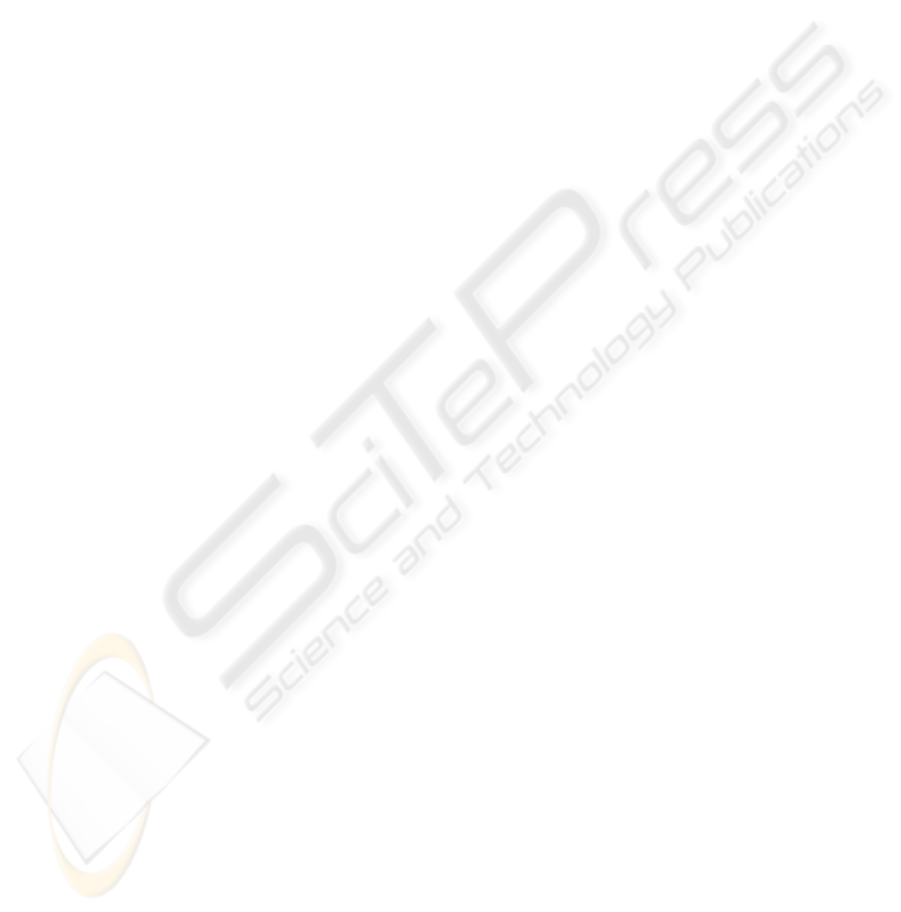
accurately measure the dynamics of pupillary
movements given various stimuli. This is commonly
considered one of the most important parameters to
make non-invasive diagnosis of many neurological
disorders.
This system has a very user friendly interface that
will allow doing clinical trials by people not
specialised in this specific technique.
The acquired results showed some error in the
contour detection. In order to surpass this problem
the detection algorithm must be optimized and a
different type of camera must be used. Since the tests
were made using a camera that works in the visible
wavelengths, there was some light reflex in the
subject’s eye. For better results an infrared camera
should have been used, but no such camera was
available at the time of this study.
The results also showed some pupil noise, that is
chiefly due to the sympathetic and parasympathetic
neural flow. The main algorithm has been improved
to a good ratio between statistical error and
processing speed.
It is relevant to say that the detection algorithm
does not use physical models and does not
approximate the pupil contour to any geometrical
figure. The speed of the algorithm is compromised
but the results are more precise.
This study sets out to design a medical
instrument that can be used by any technician or
physician to measure pupil dynamics. For example,
it could be attached to a hospital bed, to monitor the
pupil activity of patients. The interface is compiled
in a .exe file that can easily be installed in every
computer even if the computer does not have Matlab
installed.
6.1 Future Perspectives
To improve the existing system it is clear that it must
evolve into an optical device that works in infrared
light. With this feature the light reflexes in the eye
will not influence the acquired data and the system
will also work in the dark. Using a CCD camera and
an infrared light system, an image of the anterior
surface of the eye can be obtained, even when
external lighting is not present (without interference
from non-controlled stimuli).
We intend to process the acquired data using
Fourier and Wavelet analysis, to work in frequency
and time domains.
The main algorithm will adapt to every image in
such a way that the threshold value will be
calculated for each frame, so that the digital image
features may vary (brightness, contrast and gamma).
In the developed GUI all results are expressed in
pixels, but, to facilitate the physician’s work, an
algorithm must be created to convert pixels into
millimeters. However, it will not be easy, since the
system uses a zoom lens and so the pixel size is
dependent of the zoom setting.
We believe that, in the near future this
methodology can be of assistance to Ophthalmology
diagnosis by quantifying the sympathetic and
parasympathetic pupillary dilatation components.
ACKNOWLEDGEMENTS
We thank the Department of Physiology of the
Institute of Molecular Medicine for technical advice
and for sponsoring the project, and the colleagues of
Hangar 4 of FCT-UNL for laboratory assistance, and
the colleagues of the Institute of Biophysics and
Biomedical Engineering for technical advice and
helpful discussions.
REFERENCES
Enderle, John, Susan Blanchard and Joseph Bronzino.
Introduction to Biomedical Engeneering. 2
th
Edition.
San Diego: Academic Press, 2005.
Filipe, João A. Capão, F. Falcão Reis and J. Castro
Correia. “Assessement of autonomic function in high
level athletes by pupillometry”. Autonomic Neuro-
science: Basic and Clinical. Vol.104. 2003, pp. 66-72.
Gonzalez, Rafael C., Richard E. Woods and Steven
L.Eddins. Digital Image Processing using MATLAB.
New Jersey: Prentice Hall, 2004.
Hachol, A., et al. “Measurement of pupil reactivity using
fast pupilometry”. Physiol. Meas. Vol.28. 2007, pp. 61-72.
Howarth, Peter A., Gordon Heron and Louise Wittaker. “
The Measurement of Pupil cycling time”. Graete's
Arch Clin. Exp. Ophthalmol. Vol.238, 2000, pp. 826-832.
Iacoviello, Daniela. “Analysis of pupil fluctuations after a
light stimulus by image processing and neural
network”. Computers and Mathematics with
Applications. Vol.53. 2006.
Kardon, Randy. 2003. “Pupil”. In Adler's Physiology of
the Eye. Edited by Paul L. Kaufman and Albert Alm.
10th Edition. St Louis : Mosby, 713-743.
Kim, Jieun, Kyungmo Park and Gon Khan. 2004. “A
Method for Size Estimation of Amorphous Pupil in 3-
Dimensional Geometry”. Paper presented at the 26th
Annual International Conference of the IEEE EMBS,
in San Francisco.
Li, Dongheng and Derrik J. Parkhurst. “Starburst: A robust
algorithm for video-based eye tracking”. openEyes.
http://thirtysixthspan.com/openEyes/publications.html.
Rakshit, Soumyadip and Donald M. Monro. Pupil Shape
Description using Fourrier Series. 2007.
Smith, Shirley A. and S. E. Smith. “Pupil function: tests
and disorders”, in MATHIAS, Christopher J. and Sir
Roger Bannister, Autonomic Failiure: A Textbook of
Clinical Disorders of the Autonomic Nervous System.
4
th
Edition. Oxford : Oxford University Press, 2001.
DEVELOPING A PUPILLOMETER
319