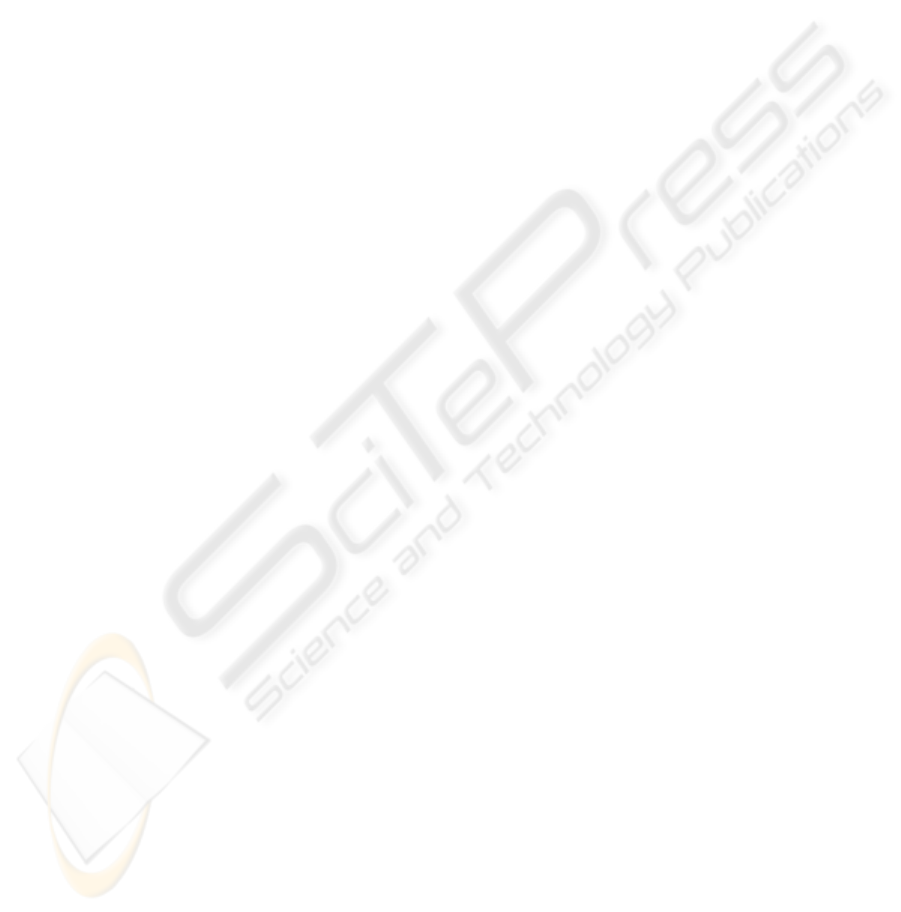
6 CONCLUSIONS
It has been shown that fusion of features has poten-
tial to improve detection accuracy of driver’s micro-
sleep. Features of two different extraction methods,
namely the Power Spectral Density (PSD) and the
Delay Vector Variance (DVV), were fused first, but
with a limited success. Fusion of different signals of
one signal type, such as all EEG signals, as well as
fusion of different signal types, namely EEG, EOG,
ETS, resulted in clear improvements. The best single
EEG signal (Cz) gained a mean error of 25 %. The
fusion of all 7 EEG signals reduced errors down to
16 %, and the fusion of all 15 signals available redu-
ces errors down to 9 %.
In high-dimensional spaces it is apparently intra-
ctable to search systematically and to approximate a
general, high-dimensional function accurately. This
is known as the so-called “curse of high dimensiona-
lity”. But, Support-Vector Machines and also other
modern methods of computational intelligence, but
not OLVQ1, impressively demonstrated that high di-
mensionality must not be a curse. OLVQ1 perfor-
mance decreased largely when the number of input
variables (features) was very high. Our results also
showed that fusion of features of all signals is most
beneficial.
Reduction is of limited advantage and was only
successful for highly correlating features, e.g. sum-
mation of PSD values in small spectral bands. There
is presumably no potential for further improvements
due to feature reduction. This was demonstrated by
computational expansive optimizations of the para-
meters of spectral bands utilizing Evolutionary Stra-
tegies. Note that these optimizations are capable to
search for different spectral bands for each subject,
if it would be advantageously.
Future work should reveal if a further diversifi-
cation of feature extraction may increase performan-
ce of discriminant analysis. Different types of featu-
res should then be fused which is likely to improve
accuracy and robustness of MSE detection.
On the one hand the detection of driver’s micro-
sleep is a relatively clear case illustration for the
problem of spontaneous behavioural events and their
detection. On the other hand, their detection in bio-
signals will be a necessary milestone for future on-
line driver monitoring technology. It explores the
extreme end of driver’s fatigue where it is essential
to avoid attention losses. The practical goal of such a
detection system is to establish a laboratory referen-
ce standard for detection of microsleep and extreme
hypovigilance. Contactless operating online driver
monitoring technology, which is currently under
development by car industry, must be validated uti-
lizing such a laboratory reference standard.
REFERENCES
Donoho, D. (2000). High-Dimensional Data Analysis: The
curses and blessing of dimensionality. Ann Conf Amer
Math Soc, Los Angeles. (http://www-stat.stan-
ford.edu/~donoho/Lectures)
Overview at http://www.microsleep.de
Schleicher, R., N. Galley, S. Briest, L. Galley (2007).
Blinks and Saccades as Indicators of Fatigue in
Sleepiness Warnings: Looking Tired? Ergonomics,
vol. 51, pp. 982-1010.
Summala, H., H. Häkkänen, T. Mikkola, J. Sinkkonen
(1999). Task effects on Fatigue Symptoms in Over-
night Driving. Ergonomics, vol. 42, pp. 798-806.
Ingre, M., T. Akerstedt, B. Peters, A. Anund, G. Kecklund
(2006). Subjective Sleepiness, Simulated Driving
Performance and Blink Duration. J Sleep Research,
vol. 15, pp. 47-53.
Santamaria, J., K.H. Chiappa (1987). The EEG of
Drowsiness in Normal Adults, J Clin Neurophysiol,
vol. 4, pp. 327-382.
Maulsby, R.L. et al (1968). The Normative Electro-
encephalographic Data Reference Library, Final
Report, Contract NASA 9-1200, National Aeronautics
and Space Administration.
Akerstedt, T., G. Kecklund, A. Knutsson (1991). Manifest
Sleepiness and the Spectral Content of the EEG
During Shift Work, Sleep, vol. 14, pp. 221-225.
Makeig, S., T. P. Jung (1995). Changes in Alertness are a
Principal Component of Variance in the EEG
Spectrum, Neuroreport, vol. 7, pp. 213-216.
Makeig, S., T.P. Jung, T. Sejnowski (2000). Awareness
During Drowsiness: Dynamics and Electrophy-
siological Correlates, Can J Exp Psychol, vol. 54, pp.
266-273.
Lal, S., A. Craig (2002). Driver Fatigue: Electroence-
phalography and Psychological Assessment, Psycho-
physiology, vol. 39, pp. 313-321.
Wilhelm, B. et al (2001). Daytime Variations in Central
Nervous System Activation Measured by a
Pupillographic Sleepiness Test, J Sleep Res, vol.10,
pp.1-7.
Sommer, D., M. Golz, J. Krajewski (2008). Consecutive
Detection of Extreme Central Fatigue. Proc 4
th
Europ
Congr Med & Biomed Engineering (EMBEC-2008),
to appear.
Golz, M., D. Sommer, M. Chen, U. Trutschel, D. Mandic
(2007). Feature Fusion for the Detection of
Microsleep Events. J VLSI Signal Proc Syst, vol. 49,
pp. 329-342, 2007.
Sommer, D., M. Golz (2007). Feature Reduction for
Microsleep Detection. In IWK’07, 52nd Int. Sci. Koll.,
Technical Univ. of Ilmenau, Germany, Vol I, 77-82.
DATA REDUCTION OR DATA FUSION IN BISOGINAL PROCESSING?
445