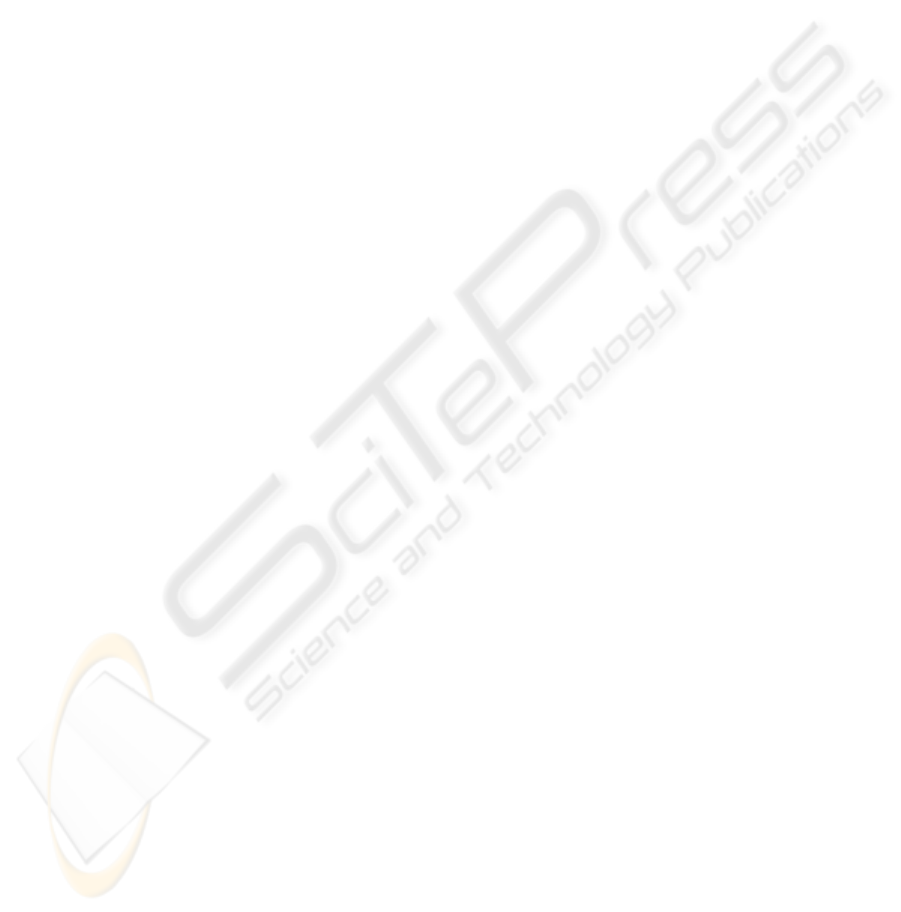
is only low motion intensity and to integrate
distance-variation- and velocity-based clustering
when the motion intensity increases as well as to
estimate the reliability of the actual result, which
could be useful for subsequent processing.
6 CONCLUSIONS
We presented three different feature clustering
methods and evaluated them with respect to their
applicability for articulated body tracking. We
showed that moving features can be clustered just by
their local and temporal properties without any
additional image information and so, that the feature
motion can allow determining the structure of the
underlying e.g. rigid or articulated body. The results
showed that an acceptable correctness can be
archived by the presented cluster techniques,
according to various circumstances. The here
presented evaluation can serve as a basis to combine
the strong points of every cluster criterion. This
becomes important with regarding further
development up to a consistent cluster tracking for
longer motion sequences, but also regarding e.g. the
connection of the feature clusters in order to define
an underlying articulated motion model.
So, the here presented alignment and grouping of
features provides a basis for the reconstruction of
complex structures and their recognition.
ACKNOWLEDGEMENTS
This work was supported by the grant from the
Ministry of Science, Research and the Arts of
Baden-Württemberg, Germany.
REFERENCES
Aggarwal, J.K., Cai, Q., 1999. Human Motion Analysis: A
Review. In Computer Vision and Image
Understanding, Vol. 73, No. 3, pp. 428-440.
Aggarwal, J.K., Cai, Q., Liao, W., Sabata, B., 1994.
Articulated and elastic non-rigid motion: A review. In
Proc. IEEE Workshop on Motion of Non-Rigid and
Articulated Objects, pp 2-14.
Bouguet, J.-Y., 2002. Pyramidal implementation of the
Lucas Kanade feature tracker, description of the
algorithm. Technical report, Intel Corporation.
Cedras, C., Shah, M. 1994. A Survey of Motion Analysis
from Moving Light Displays. In IEEE Conf. on
Computer Vision and Pattern Recognition, pp. 214-
221.
Corazza, S., Mündermann, L., Andriacchi, T., 2007. A
framework for the functional identification of joint
centers using markerless motion capture, Validation
For The Hip Joint. In Journal of Biomechanics
Giese, M. A., Poggio, T., 2003. Neural mechanisms for
the recognition of biological movements and action. In
Nature Reviews Neuroscience, Vol. 4, pp. 179-192.
Holstein, H., Li, B., 2002. Low Density Feature Point
Matching for Articulated Pose Identification. In
British Machine Vision Conference 2002, pp 678 - 687
Johansson, G., 1973. Visual perception of biological
motion and a model for its analysis. In Perception &
Psychophysics, Vol. 14, No. 2, pp. 201 - 211.
Koehler, H., Pruzinec, M., Feldmann, T., Woerner, A.,
2008. Automatic Human Model Parametrization From
3D Marker Data For Motion Recognition. In Int. Conf.
in Central Europe on Computer Graphics,
Visualization and Computer Vision, Pilsen, 2008
Moeslund, T.B., Granum, E., 2001. A survey of computer
vision-based human motion capture. In Computer
Vision and Image Understanding, Vol. 81, No. 3, pp.
231-268.
Moeslund, T.B., Hilton. A., Krüger, V., 2006. A survey of
advances in vision-based human motion capture and
analysis. In Computer Vision and Image
Understanding, Vol. 104 , No. 2, pp. 90 – 126.
Nicolescu, M., Medioni, G., 2002. Perceptual Grouping
from Motion Cues Using Tensor Voting in 4-D. In
European Conf. on Computer Vision, LNCS 2352, pp.
423 - 437
Shi, J., Tomasi, C., 1994. Good Features to Track. In IEEE
Conf. on Computer Vision and Pattern Recognition,
pp. 593 - 600.
Silaghi, M.-C., Plänkers, R., Boulic, R., Fua, P.,
Thalmann, D., 1998. Local and Global Skeleton
Fitting Techniques for Optical Motion Capture. In
Proc. of the International Workshop on Modelling and
Motion Capture Techniques for Virtual Environments,
LNCS 1537, pp. 26-40.
Song, Y., Goncalves, L., Di Bernardo, E., Perona,P., 1999.
Monocular Perception of Biological Motion -
Detection and Labeling. In Proc. of the Int. Conf. on
Computer Vision, Vol. 2, pp. 805-812.
Song, Y., Goncalves, L., Perona, P., 2003. Unsupervised
Learning of Human Motion. In IEEE Transactions on
Pattern Analysis and Machine Intelligence, Vol. 25 ,
No. 7, pp. 814-827
Tomasi, C., and Kanade, T., 1991. Detection and tracking
of point features.
Technical Report, School of
Computer Science, Carnegie Mellon University
Ullman, S., 1983. Computational Studies in the
Interpretation of Structure and Motion: Summary and
Extension. In Human and Machine Vision, Academic
Press
VISAPP 2009 - International Conference on Computer Vision Theory and Applications
584