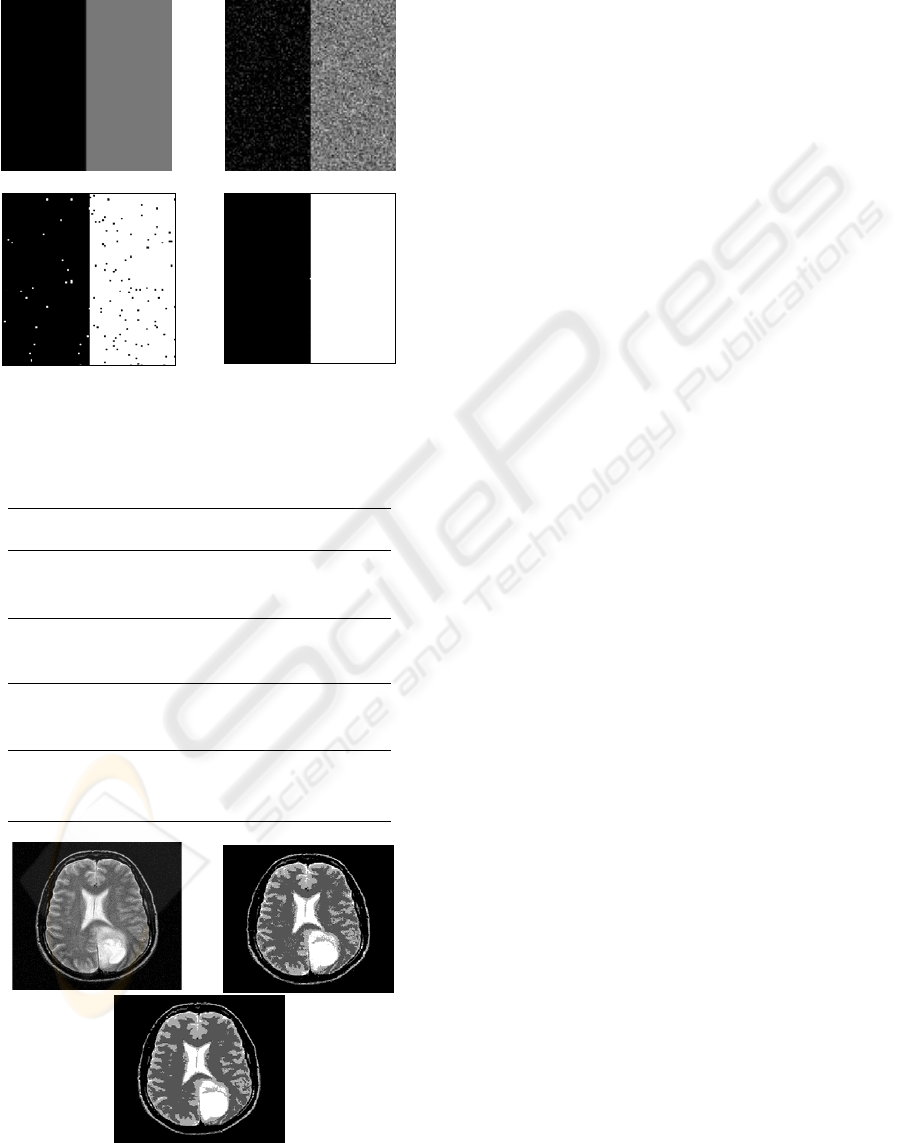
by a parameter α to control the neighborhood effect.
Thus, spurious blobs due to the presence of noise are
eliminated and the algorithm gives more
homogenous regions than other clustering methods.
Figure 2: (a) Original image; (b)image with Gaussian
noise, segmented image by (c) FCM; (d ) FCM(α=0.97).
Table 2: The clustering results of three images using
various FCM techniques.
Images Methods Vpc Vpe
Original MR
image
FCM 0.871 0.267
RFCM 0.967 0.040
Gaussian noise
added MR image
FCM 0.828 0.288
RFCM 0.939 0.081
Salt and pepper
added MR image
FCM 0.928 0.138
RFCM 0.979 0.037
Mixed noise
added MR image
FCM 0.804 0.334
RFCM 0.943 0.097
Figure 3: (a) MR image with additive noise. Segmented
image by (b) FCM; (c) RFCM(α = 0.25).
The proposed method seems to be more robust to
noise and it yields more homogenous labeling.
However, this method has a drawback of blurring
some fine details along the clustering process
especially for high value of the parameter α. Thus,
further works will emphasis on segmenting noisy
image by incorporation spatial information and
preserving image details.
REFERENCES
Ahmed, M.N., Yamany, S.M., Mohamed, N., Farag, A.A.
and Moriarty, T., 2002. A modified fuzzy c-means
algorithm for bias field estimation and segmentation of
MRI data. IEEE Trans. Med. Imaging, v21, 193-199.
Bezdek(a), J., Hall, L., Clarke, L., 1993. Review of MR
image segmentation using pattern recognition. Med
Phys, v20, 1033-1048.
Bezdek(b), JC., 1974. Cluster validity with fuzzy sets. J
Cybern, v3, 58-72.
Bezdek(c), JC., 1975. Mathematical models for systematic
and taxonomy. In: proceedings of eigth international
conference on numerical taxonomy, San Francisco, pp
143-166.
Chen(a), W.J., Giger, M.L., Bick, U., 2006. A fuzzy c-
means (FCM)-based approach for computerized
segmentation of breast lesions in dynamic contrast
enhanced MRI images, Acad. Radiol, Vol. 13, No. 1,
pp. 63–72.
Chen(b), S.C., Zhang, D.Q., 2004. Robust image
segmentation using FCM with spatial constraints
based on new kernel-induced distance measure, IEEE
Trans. Syst. Man Cybern. B, vol.34, no.4, pp.1907–
1916.
Goldszal, A. F., Davatzikos, C., Pham, D. L., Yan, M. X.,
Bryan, H. R. N. and Resnick, S. M, 1998. “An image
processing system for qualitative and quantitative
volumetric analysis of brain images,” J. Comput.
Assist. Tomog, 22(5):827-37.
Leemput, K. V., Maes, F., Vandermeulen, D. and In
Suetens, P., 1999. Automated model-based tissue
classification of MR images of the brain, IEEE Trans.
Med. Imag, vol. 18, n
o
10, pp. 897-908.
Lyer, NS., Kandel, A., Schneider, M., 2002. Feature-
based fuzzy classification for interpretation of
mammograms. Fuzzy Sets Syst, 114:, pp. 271–80.
Pham, D.L., Prince, J.L., 1999. An adaptive fuzzy c-means
algorithm for image segmentation in the presence of
intensity inhomogeneities, Pattern Recognition
Letters, v.20 n.1, pp. 57-68.
Shen, S., Sandham, W., Grant, M., and Ster, A., 2005.
MRI Fuzzy Segmentation of Brain Tissue Using
Neighborhood Attraction with Neural-Network
(
)
(a)
(c)
(
)
(a)
(c)
(
)
IMAGAPP 2009 - International Conference on Imaging Theory and Applications
90