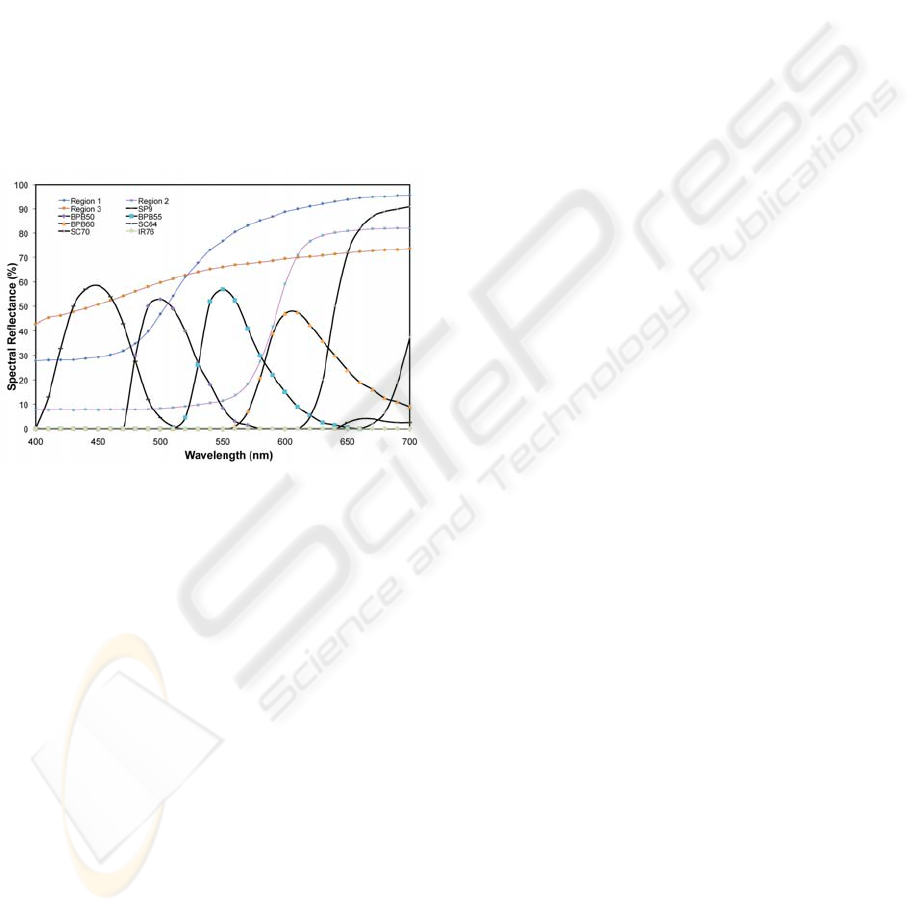
be observed that only two filters were able to collect
information.
In addition, it is interesting to note how well the
estimated spectral reflectances above 700 nm were
convergent except for Region 1. Evidently, the
influence of high specular reflection also affected
the reconstructed reflectance at that wavelength. It is
difficult to ascertain its accuracy because the
measured data only goes up to 700 nm but it may be
assumed that it might be good enough.
Why is important to reconstruct the spectral
reflectance above the visible range? This is because
previous studies have shown that some materials
have unique spectral features at the near infrared
range (Anderson, 1947). In addition, acquiring
images beyond the visible range can help increase
the amount of information available from the image.
Figure 11: Spectral characteristics of the filters used in
capturing the multispectral images along with the
measured spectral reflectance of the three selected regions.
4 CONCLUSIONS
In this study, the influence of lighting condition on
image stitching and spectral reflectance
reconstruction was explored. Using multispectral
images, spectral reflectance of a traditional Japanese
painting was estimated by the pseudoinverse
method. Results show that specular reflection, which
is influenced by illumination, significantly affects
the accuracy of the reconstruction. It was also
observed that the spectral features of the filters used
play an important role. According to the comparison
of spectral reflectance curves, the estimation is more
accurate between 420-580 nm especially for Region
2 because at least four filters were able to collect
information within that range. On the other hand, it
was shown that image stitching was greatly
influenced by the light distribution on the target and
its surface reflection characteristics. Stitching lines
were highly visible when specular reflection is
severe. It is also observed that the calibration
technique was only effective within a 20-pixel
standard deviation-threshold. Beyond this, more
advanced calibration technique is necessary.
ACKNOWLEDGEMENTS
This work has been done as part of the project “An
Integrated System for Secure and Dynamic Display
of Cultural Heritage” sponsored by Japan Science
and Technology Agency, Regional Resources
Development Program. This collaborative project
was organized by Kyoto University Graduate School
of Engineering, S-tennine Kyoto (Ltd) and Kyushu
National Museum. The Authors would like to
express their thanks to Imazu Setsuo of Kyushu
National Museum and other staff of the museum
and, Oshima of S-tennine Kyoto and his group for
supporting this work.
REFERENCES
Anderson, J.A., 1947. The diffuse reflectance of paints in
the near-infrared. Journal of the Optical Society of
America. 37(10), pp. 771-777.
Balas, C., Papadakis, V., Papadakis, N., Papadakis, A.,
Vazgiouraki, E., Themelis, G., 2003. A novel hyper-
spectral imaging apparatus for the non-destructive
analsyis of objects of artistic and histori values.
Journal of Cultural Heritage, 4, pp 330s-337s.
Biehl, L., and Landgrebe, D., 2002. MultiSpec—a tool for
multispectral–hyperspectral image data analysis
Computers & Geosciences, 28(10), pp.1153-1159
Conde, J., Haneisihi, H., Yamaguchi, M., Ohyama, N.,
Baez, J., 2004. Spectral reflectance estimation of
ancient Mexican codices, multispectral images
approach. Revisita Mexhicana De Fisica, 50(5), pp.
484-489.
Elaksher, A., 2008, Fusion of hyperspectral images and
lidar-based dems for coastal mapping. Optics and
Lasers in Engineering, 46(7), pp. 493-498
Lane, G.R., Martin, C., Pirard, E., 2008. Techniques and
applications for predictive metallurgy and ore
characterization using optical image analysis.
Minerals Engineering, 21(7), pp. 568-577.
Lee, H-C., 2005. Introduction to color imaging science.
Cambridge, UK, Cambridge University Press.
MacAdam, D., 1993. Selected Papers on Colorimetry-
Fundamentals, volume 77 of Milestone Series.
Bellingham, Washington, SPIE Optical Engineering
Press.
Marengo, E., Liparota, M.C., Robotti, E., Bobba, M.,
2005. Multivariate calibration applied to te field of
MULTISPECTRAL IMAGING - The Influence of Lighting Condition on Spectral Reflectance Reconstruction and Image
Stitching of Traditional Japanese Paintings
19