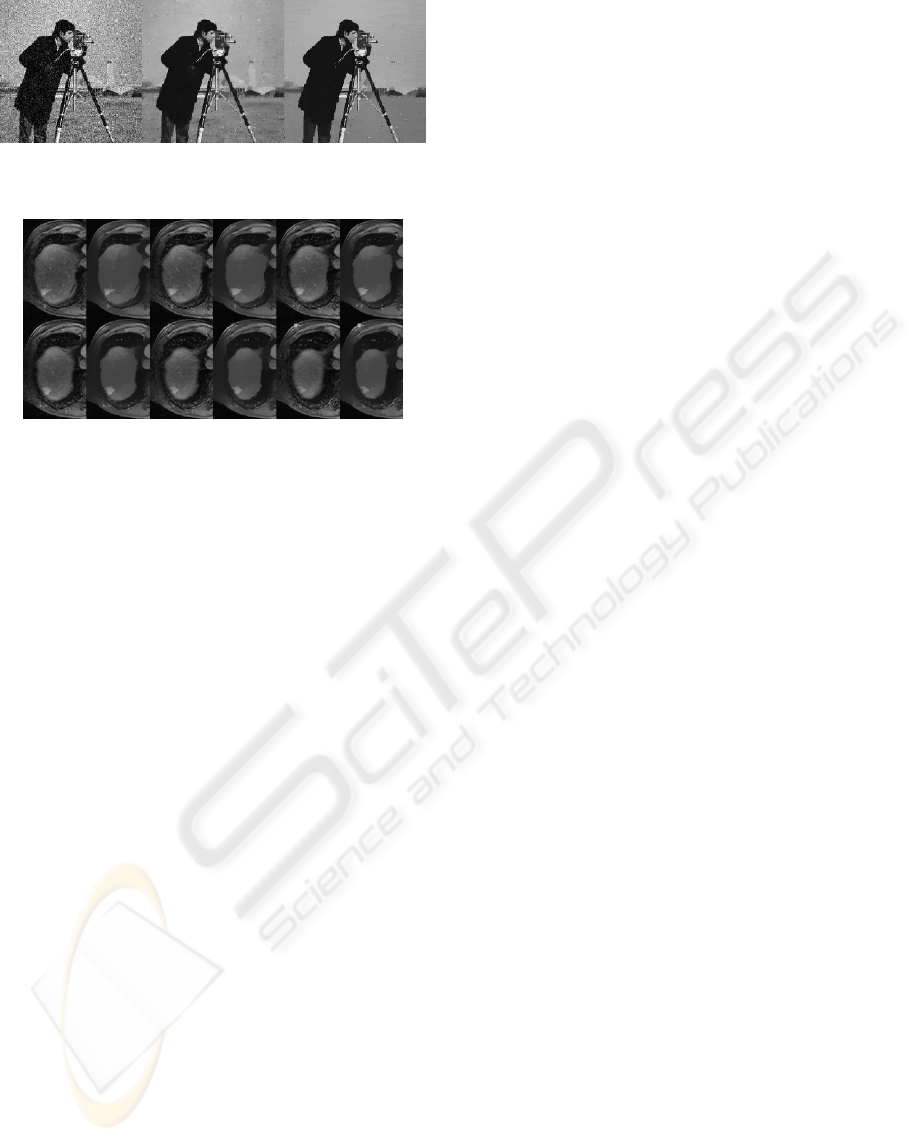
Figure 2: 2D filter with AOS a) Original, b) p = 2, k =
1.8ms, c) p = 3, k = 0.54ms
Figure 3: 3D Diffusion where p = 3, k = 0.04ms (original
on the left and on the right, processed)
does not exceed the threshold. If p = 2 the noise in-
cidence is higher and the contrast is accentuated to a
lesser degree.
This procedure has been applied to the multi-
phase segmentation of the liver based on magnetic
resonance (Platero et al., 2008). Due to the high vol-
ume of information, the number of iterations is re-
duced to 5. The figure below illustrates just six con-
secutive slices showing a hepatic lesion. We have se-
lected α
th
= 0.1. The slices show the increase in con-
trast of both the organ and the tumour.
5 CONCLUSIONS
The proposed objective is to determine a numerical
method that allows for images to be automatically
enhanced at a low computational cost. In this in-
stance, the method is based on the nonlinear diffu-
sion filter. We have selected a family of diffusivities
without control parameters. Based on the analytical
expression, obtained on the discrete evolution of 3
pixels through the resolution of a semi-implicit Eu-
ler method, we have experimentally verified stability,
consistency and enhancement properties. Using the
analytical model, we have determined the relationship
between the diffusion time and the gradient module.
Experimentally, the value of p = 3 has been consid-
ered the most suitable based on the convergence to the
analytical model presented, to the conclusions drawn
and the lower incidence of the staircase.
ACKNOWLEDGEMENTS
This work is supported DPI-2007-63654 project of
Spanish Ministry of Science.
REFERENCES
Andreu, F., Caselles, V., Daz, J., and Mazn, J. (2002). Some
qualitative properties for the total variation flow. Jour-
nal of Functional Analysis, 188:516–547.
Buades, A., Coll, B., and Morel, J. M. (2005). A review
of image denoising algorithms with a new one. Multi-
scale Modeling & Simulation, 4:490–530.
Catte, F., Lions, P. L., Morel, J. M., and Coll, T. (1992). Im-
age selective smoothing and edge detection by nonlin-
ear diffusion. SIAM Journal on Applied Mathematics,
29(1):182–193.
Keeling, S. L. and Stollberger, R. (2002). Nonlinear
anisotropic diffusion filters for wide range edge sharp-
ening. Inverse Problems, 18:175–190.
Kichenassamy, S. (1997). The perona-malik paradox. SIAM
Journal on Applied Mathematics, 57(5):1328–1342.
Perona, P. and Malik, J. (1990). Scale-space and edge detec-
tion using anisotropic diffusion. IEEE Transaction on
pattern analysis and machine intelligence, 12(7):629–
639.
Platero, C., Poncela, J. M., Gonzalez, P., Tobar, M. C., San-
guino, J., Asensio, G., and Santos, E. (2008). Liver
segmentation for hepatic lesions detection and char-
acterisation. Biomedical Imaging: From Nano to
Macro, 2008.ISBI 2008.5th IEEE International Sym-
posium on, pages 13–16.
Steidl, G., Weickert, J., Brox, T., Mrzek, P., and Welk, M.
(2004). On the equivalence of soft wavelet shrinkage,
total variation diffusion, total variation regularitation,
and sides. SIAM Journal on Applied Mathematics,
42(2):686–713.
Teboul, S., Blanc-Feraud, L., Aubert, G., and Barlaud, M.
(1998). Variational approach for edge-preserving reg-
ularization using couple pdes. IEEE Trasanctions on
Image Processing, 7:387–397.
Tsurkov, V. I. (2000). An analytical model of edge protec-
tion under noise suppression by anisotropic diffusion.
Journal of Computer and Systems Sciences Interna-
tional, 39(3):437–440.
Weickert, J. and Benhamouda, B. (1997). A semidiscrete
nonlinear scale-space theory and its relation to the
perona-malik paradox. Advances in Computer Vision,
pages 1–10.
Weickert, J., ter Haar Romeny, B., and Viergever, M. A.
(1998). Efficient and reliable schemes for nonlinear
diffusion filtering. IEEE Trasanctions on Image Pro-
cessing, 7(3):398–410.
Welk, M., Steidl, G., and Weickert, J. (2008). Locally an-
alytic schemes: A link between diffusion filtering and
wavelet shrinkage. Applied and Computational Har-
monic Analysis, 24(2):195–224.
ANALYTICAL APPROXIMATIONS FOR NONLINEAR DIFFUSION TIME IN MULTISCALE EDGE
ENHANCEMENT
81