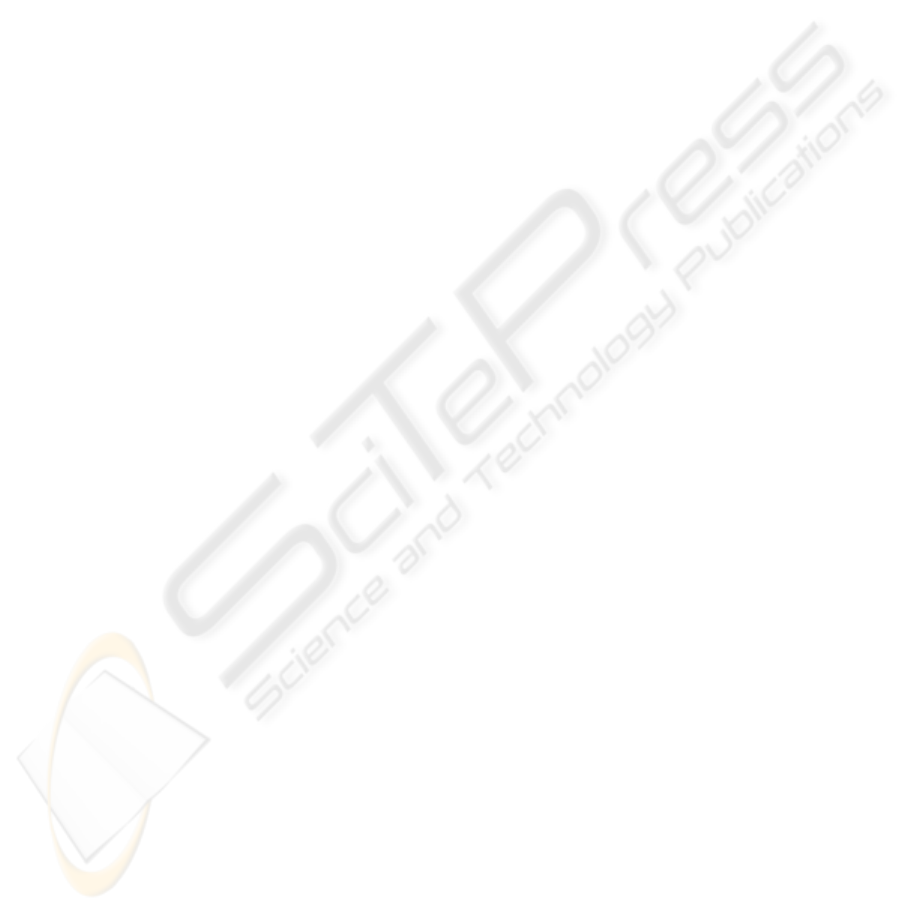
nal space, but in a much higher 599-dimensional fea-
ture space composed of non-linear transformations of
the original pixels. In such limited sample and high-
dimensional problem, where N(= 600) > n(= 256),
it seems that we can further improve the classification
accuracy by exploring more features than is possible
in the linear case.
7 CONCLUSIONS
In this work, we extended the MLDA approach to its
non-linear version. This non-linear version of MLDA,
here called KMDA, is a KPCA+MLDA two-stage
method. To evaluate the KMDA effectiveness, ex-
periments on face recognition using the well-known
ORL and FERET face databases were carried out
and compared with other existing kernel discrimi-
nant methods, such as GDA and RKDA. The classi-
fication results indicate that KMDA performs as well
as GDA and RKDA, with the advantages of being a
straightforward stabilization approach for the within-
class scatter matrix that uses a pre-defined number of
higher-order features whenever the number of train-
ing samples is larger than the original dimensionality
of the input data.
As future work, we intend to directly regularize
the eigen-analysis of the within-class scatter matrix in
the feature space, without a KPCA intermediate step.
ACKNOWLEDGEMENTS
The authors would like to thank the support provided
by PCI-LNCC, FAPESP (2005/02899-4), CNPq
(472386/2007-7) and CAPES (094/2007). Also, por-
tions of the research in this paper use the FERET
database of facial images collected under the FERET
program.
REFERENCES
Baudat, G. and Anouar, F. (2000). Generalized discriminant
analysis using a kernel approach. Neural Computa-
tion, 12(10):2385–2404.
Belhumeur, P. N., Hespanha, J. P., and Kriegman, D. J.
(1997). Eigenfaces vs. fisherfaces: Recognition us-
ing class specific linear projection. IEEE Transac-
tions on Pattern Analysis and Machine Intelligence,
19(7):711–720.
Chen, L., Liao, H., Ko, M., Lin, J., and Yu, G. (2000).
A new lda-based face recognition system which can
solve the small sample size problem. Pattern Recog-
nition, 33(10):1713–1726.
Devijver, P. and Kittler, J. (1982). Pattern Classification: A
Statistical Approach. Prentice-Hall.
Fukunaga, K. (1990). Introduction to Statistical Pattern
Recognition. Morgan Kaufmann, San Francisco, 2nd
edition.
Lu, J., Plataniotis, K. N., and Venetsanopoulos, A. N.
(2003). Face recognition using kernel direct discrimi-
nant analysis algorithms. IEEE Transactions on Neu-
ral Networks, 14(1):117–126.
Park, C. H. and Park, H. (2005). Nonlinear discriminant
analysis using kernel functions and the generalized
singular value decomposition. SIAM J. Matrix Anal.
Appl., 27(1):87–102.
Phillips, P. J., Wechsler, H., Huang, J., and Rauss, P. (1998).
The feret database and evaluation procedure for face
recognition algorithms. Image and Vision Computing,
16:295–306.
Samaria, F. and Harter, A. (1994). Parameterisation of a
stochastic model for human face identification. In
Proceedings of 2nd IEEE Workshop on Applications
of Computer Vision.
Scholkopf, B., Smola, A., and Muller, K.-R. (1998). Non-
linear component analysis as a kernel eigenvalue prob-
lem. Neural Computation, 10(5):1299–1319.
Swets, D. L. and Weng, J. J. (1996). Using discrimi-
nant eigenfeatures for image retrieval. IEEE Trans-
actions on Pattern Analysis and Machine Intelligence,
18(8):831–836.
Thomaz, C. E., Gillies, D. F., and Feitosa, R. Q. (2004).
A new covariance estimate for bayesian classifiers in
biometric recognition. IEEE Transactions on Circuits
and Systems for Video Technology, Special Issue on
Image- and Video-Based Biometrics, 14(2):214–223.
Thomaz, C. E., Kitani, E. C., and Gillies, D. F. (2006). A
maximum uncertainty lda-based approach for limited
sample size problems - with application to face recog-
nition. Journal of the Brazilian Computer Society,
12(2):7–18.
Yang, J., Jin, Z., yu Yang, J., Zhang, D., and Frangi, A. F.
(2004). Essence of kernel fisher discriminant: Kpca
plus lda. Pattern Recognition, 37:2097–2100.
Yang, J. and Yang, J. (2003). Why can lda be performed in
pca transformed space? Pattern Recognition, 36:563–
566.
Yu, H. and Yang, J. (2001). A direct lda algorithm for high
dimensional data - with application to face recogni-
tion. Pattern Recognition, 34:2067–2070.
Zheng, W., Zou, C., and Zhao, L. (2005). An improved al-
gorithm for kernel principal component analysis. Neu-
ral Process. Lett., 22(1):49–56.
VISAPP 2009 - International Conference on Computer Vision Theory and Applications
346