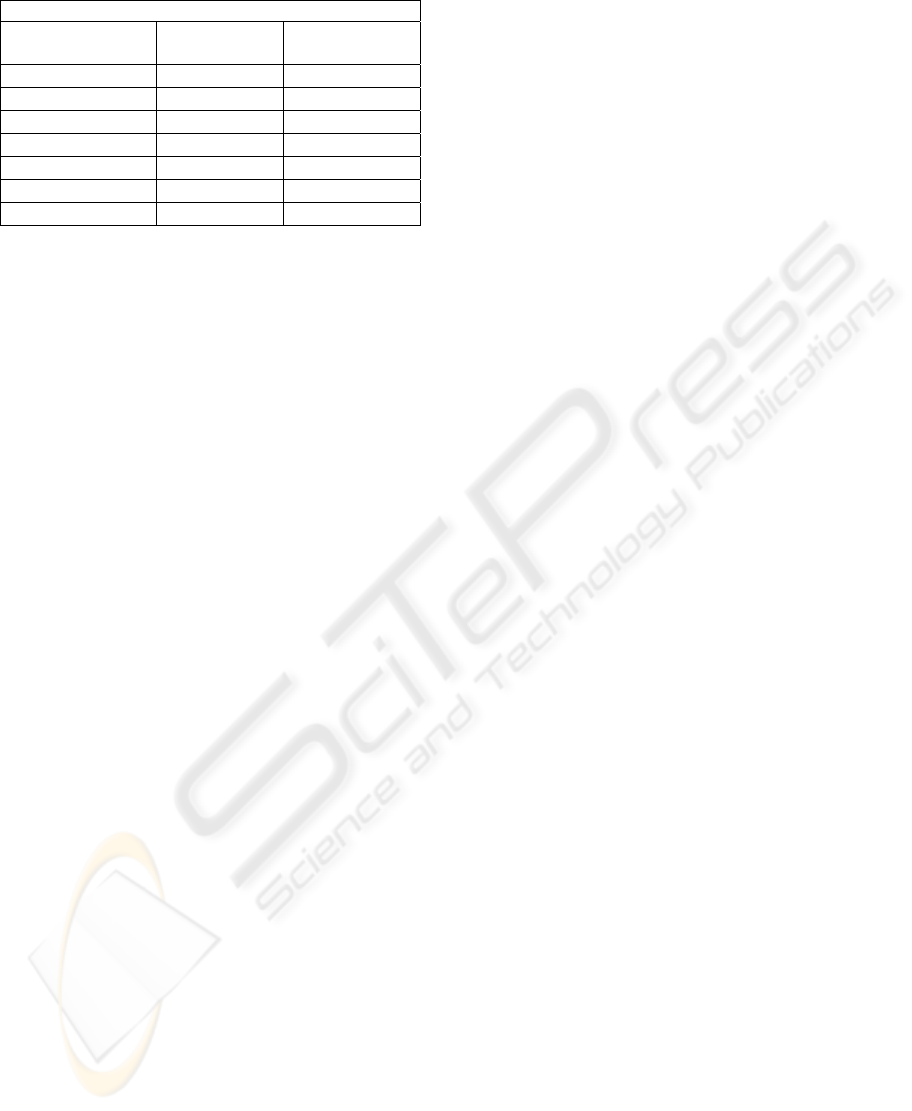
Table 2: Tracking evaluation results for test 2.
Test 2 (tracking left ball in test sequence)
Tracking Method
Spatial
Overlap
Centroid
Distance
TMC 0.22±0.27 44.34±52.24
BM 0.23±0.29 42.51±50.42
HRS 0.25±0.31 44.93±51.96
VRFS 0.28±0.35 42.82±52.62
PDFS 0.50±0.30 36.27±86.95
GCBT 0.20±0.27 70.69±68.80
PIORT 0.60±0.23 3.94±4.98
Regarding the failure ratio, a value of zero was
obtained for all methods except FR=0.09 for GCBT
in test 1 and FR=0.28 for PDFS tracker in test 2.
5 CONCLUSIONS
A previously proposed method for object tracking,
which was integrated in a probabilistic framework
for object recognition and tracking in video
sequences (Amézquita, 2007; Amézquita, 2008), has
been extended in this work to deal with same-class
object crossing and occlusion. The new method is
able to select and track only the target object after it
crosses or is occluded by another object which is
recognised as belonging to the same class. However,
this has been achieved under the assumption that the
trajectory of the target object is relatively stable in
the preceding part of the sequence. The method may
fail in a large changing motion of this object (either
caused by its own motion or by a moving camera).
In that case, a more complex criterion would be
needed to select the target object after crossing or
occlusion. This is left for future work.
ACKNOWLEDGEMENTS
This research was partially supported by Consolider
Ingenio 2010, project CSD2007-00018, by the
CICYT project DPI 2007-61452 and by a grant from
the Universitat Rovira i Virgili (URV).
REFERENCES
Amézquita Gómez N., Alquézar R. and Serratosa F., 2007.
A new method for object tracking based on regions
instead of contours. In Proc. IEEE Int. Conf. on
Computer Vision and Pattern Recognition (CVPR),
Minneapolis, Minnesota.
Amézquita Gómez N., Alquézar R. and Serratosa F., 2008.
Dealing with occlusion in a probabilistic object
tracking method. In Proc. IEEE Int. Conf. on
Computer Vision and Pattern Recognition (CVPR),
Anchorage, Alaska.
Bugeau A. and Pérez P., 2008. Track and cut:
simultaneous tracking and segmentation of multiple
objects with graph cuts. In Proc. Third Int. Conf. on
Computer Vision Theory and Applications, Funchal,
Madeira, Portugal.
Collins R. and Liu Y., 2005. On-line selection of
discriminative tracking features. In IEEE Trans.
Pattern Anal. Machine Intell., 27 (10), 1631-1643.
Collins R., Zhou X. and Teh S.K., 2005. An open source
tracking testbed and evaluation web site. In IEEE
International Workshop on Performance Evaluation of
Tracking and Surveillance (PETS’2005).
Comaniciu D. and Meer P., 1999. Mean shift analysis and
applications. In Proceedings ICCV’99, 1197-1203.
Comaniciu D. and Meer P., 2002. Mean shift: a robust
approach toward feature space analysis. In IEEE
Trans. Pattern Anal. Machine Intell., 24 (5), 603-619.
Comaniciu D., Ramesh V. and Meer P., 2000. Real-time
tracking of non-rigid objects using mean shift. In
Proc. IEEE Conf. on Computer Vision and Pattern
Recognition, Hilton Head, SC, vol. II, 142–149.
Comaniciu D., Ramesh V. and Meer P., 2003. Kernel-
based object tracking. In IEEE Trans. Pattern Anal.
Machine Intell., 25 (4), 564-577.
Hariharakrishnan K. and Schonfeld D., 2005. Fast object
tracking using adaptive block matching: In IEEE
Trans. Multimedia, 7 (5), 853-859.
Ito K. and Sakane S., 2001. Robust view-based visual
tracking with detection of occlusions: In Proc. Int.
Conf. Robotics Automation, vol. 2, 1207-1213.
Jepson A.D., Fleet D.J., and EI-Maraghi T.F., 2003.
Robust online appearance models for visual tracking.
In IEEE Trans. PAMI, 25 (10), 1296-1311.
Nguyen H.T. and Smeulders A.W.M., 2004. Fast occluded
object tracking by a robust appearance filter. In IEEE
Trans. Pattern Anal. Mach. Intell., 26 (8), 1099-1104.
Pan J., and Hu B., 2007. Robust occlusion handling in
object tracking. In Proc. IEEE Int. Conf. on Computer
Vision and Pattern Recognition (CVPR), Minneapolis,
Minnesota.
Senior A. et al., 2006. Appearance models for occlusion
handling. In J. Image Vis. Comput., 24 (11), 1233-
1243.
Yin F., Makris D. and Velastin S.A., 2007. Performance
evaluation of object tracking algorithms. In Proc. 10th
IEEE Int. Workshop on Performance Evaluation of
Tracking and Surveillance (PETS’2007).
Zhou S.K., Chellappa R. and Moghaddam B., 2004.
Visual tracking and recognition using appearance-
adaptive models in particle filters. In IEEE Trans.
Image Process., 13 (11), 1491-1506.
Zhu L., Zhou J. and Song J., 2008. Tracking multiple
objects through occlusion with online sampling and
position. In Pattern Recognition, 41 (8), 2447-2460.
VISAPP 2009 - International Conference on Computer Vision Theory and Applications
594