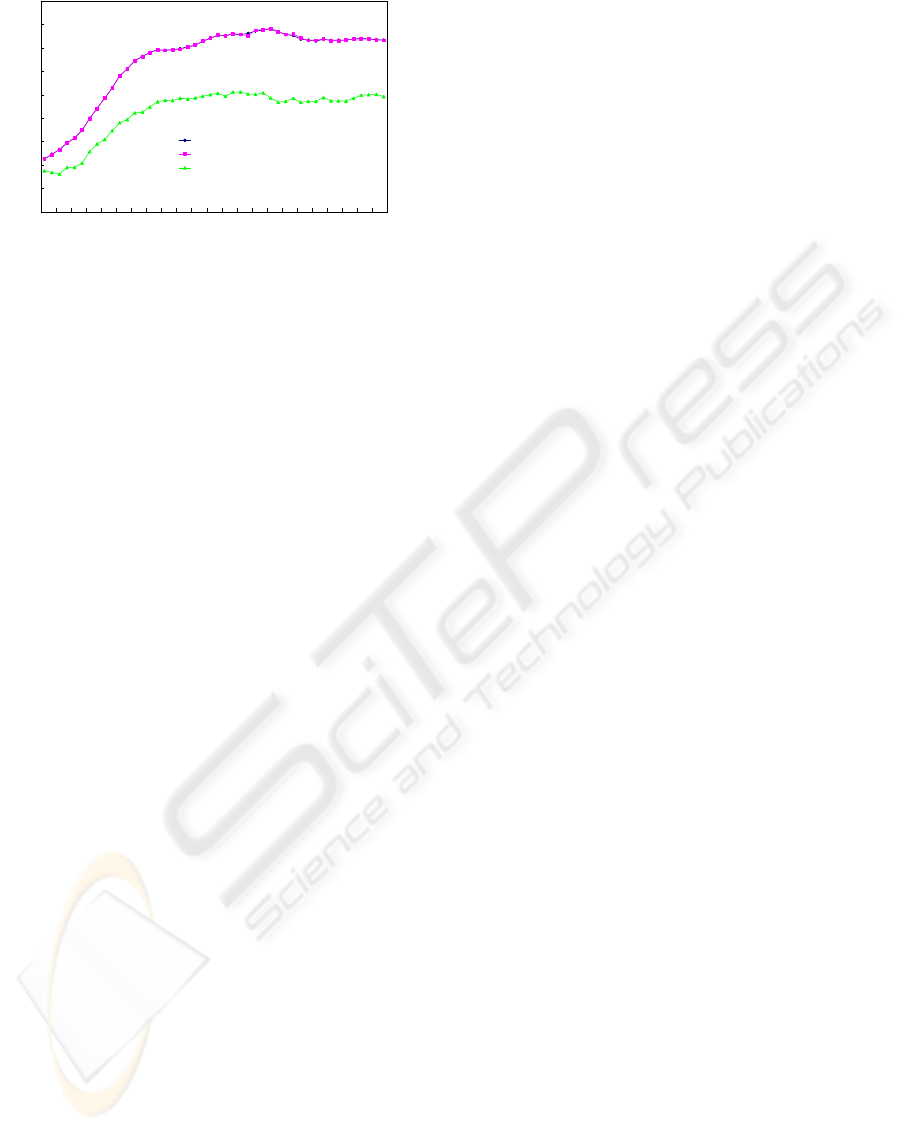
0
0.1
0.2
0.3
0.4
0.5
0.6
0.7
0.8
0.9
400 450 500 550 600 650 700 750 800 850
Wavelength [nm]
Reflectance
Gold powder (PIM)
Gold powder (AIC)
Gold powder (Measured)
Figure 9: Spectral reflectance reconstruction of glossy
target.
5 SUMMARY AND
CONCLUSIONS
In this study the optimum regression model and
channel selection for hyperspectral image
reconstruction from multispectral image was
investigated. Adopting Akaike’s information
criterion (AIC) as the selection model, the optimum
regression model was explored. In addition, a new
statistical approach for determining the number of
channels and selecting the best combination of
channels was presented.
The optimum regression model was successfully
constructed based on AIC. AIC-based method was
evaluated by comparing it with pseudoinverse
method which is a widely used technique for
spectral reflectance reconstruction from
multispectral images. The results show that the
model based on AIC is more accurate than the model
based on pseudoinverse method and indicate that the
AIC-based method is stable even with noise.
The results also show that it is quite difficult to
reconstruct the spectral reflectance of a target with
strong specular reflection. This is due to the lighting
condition and measuring condition. In order to
obtain the hyperspectral image of glossy samples,
further investigation on the image acquisition system
and the mathematical approach of spectral
reflectance reconstruction is required.
ACKNOWLEDGEMENTS
This work has been done as part of the project “An
Integrated System for Secure and Dynamic Display
of Cultural Heritage” sponsored by Japan Science
and Technology Agency, Regional Resources
Development Program. This collaborative project
was organized by Kyoto University Graduate School
of Engineering, S-tennine Kyoto (Ltd) and Kyushu
National Museum. The Authors would like to
express their thanks to Imazu Setsuo of Kyushu
National Museum and other staff of the museum and
to Oshima Yasushi of S-tennine Kyoto and his group
for supporting this work.
REFERENCES
Hardeberg, J. Y., 2003, Filter Selection for Multispectral
Color Image Acquisition, PICS Conference, VA 2003,
pp.177-181
Ukishima M., Nakaguchi T., Kato K., Fukuchi Y.,
Tsumura N., Matsumoto K., Yanagawa N., Morita H.,
Miyake Y., 2005, An Evaluation of Sharpness in
Different Image of an Internal Organ, The 13
th
Color
Imaging Conference, (CIC13), pp.261-263
Carcagni P., Della Patria A., Fontana R., Greco M.,
Mastroianni M., Materazzi M., Pampaloni E., Pezzati
L., 2006, Multispectral imaging of paintings by optical
scanning, Optics and Lasers in Engineering 45,
pp.360-367
Burns P. D. and Berns R. S., 1996, Analysis of
multispectral image capture, Proceedings of the
Fourth Color Imaging Conference, pp.19-22
Farrell J. E., Cupitt J., Saunders D., and Wandell B. A.,
1999, Estimating spectral reflectances of digital
images of art, International Symposium of
Multispectral Imaging and Color Reproduction for
Digital Archives, pp. 58-64
Shimano N., Terai K., Hironaga M., 2007, Recovery of
spectral reflectances of objects being imaged by
multispectral camera, Optical Society of America, 24,
pp.3211-3219
Cotte P., Dupraz D., 2006, Spectral imaging of Leonardo
Da Vinci’s Mona Lisa: A true color smile without the
influence of aged varnish, CGIV Final Program and
Proceedings
Zhao Y., Taplin L. A., Nezamabadi. M, Bern R. S. , 2005,
Using the matrix R method for spectral image archives,
Proceedings of the 10
th
Congress of the International
Colour Association, pp.469-472
RECONSTRUCTION OF HYPERSPECTRAL IMAGE BASED ON REGRESSION ANALYSIS - Optimum Regression
Model and Channel Selection
55