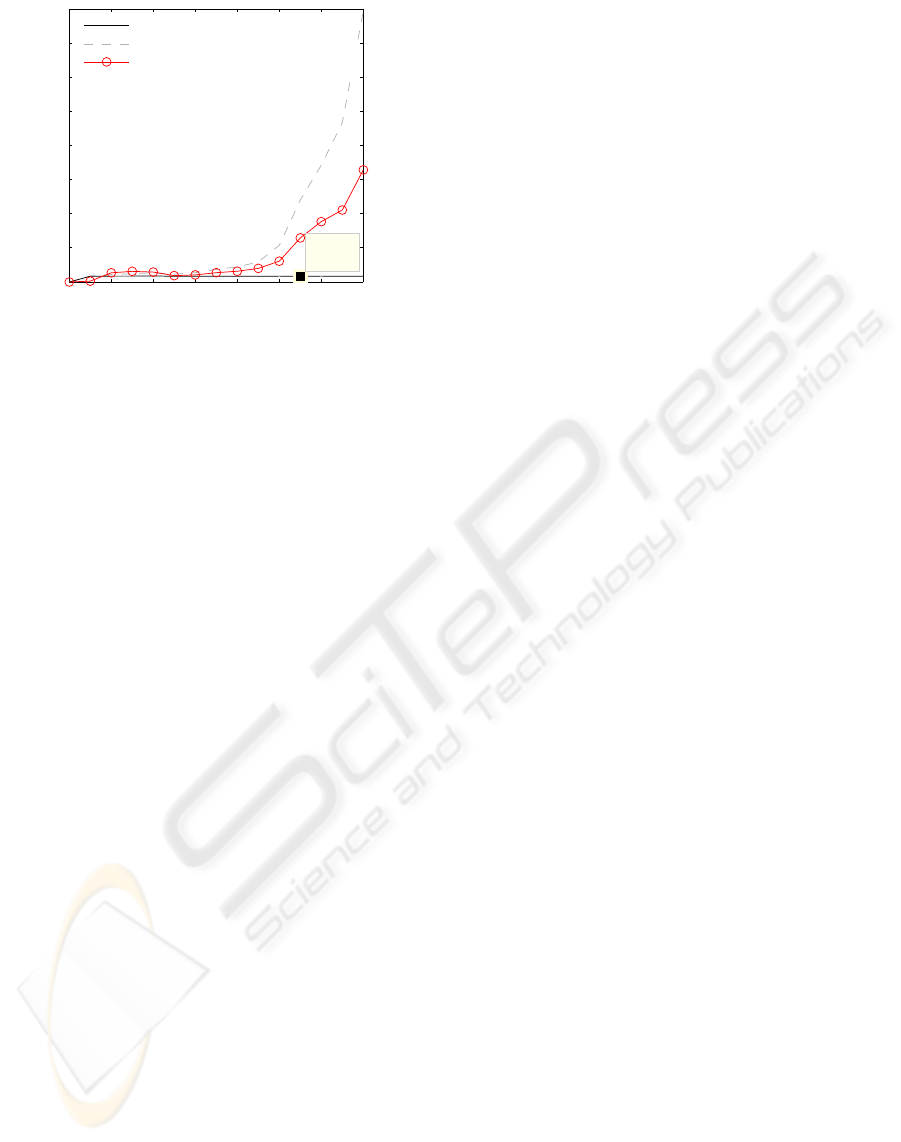
0 20 40 60 80 100 120 140
0
10
20
30
40
50
60
70
80
Mean Error [mm] / No. of iterations
X: 110
Y: 1.685
Registration result (error<=10mm)
Registration result (with errors)
Wrong results (error>10mm)
Figure 2: Influence of initial misregistration on the mean
error after registration in successful registration cases and
cases where the registration did not decrease the error under
10 mm level. Percentage of these failure cases is shown by
the third series.
could be understood as a mean distance of every point
of a volume to its transformed counterpart.
We continuously generated random transforms, so
that there was at least one hundred of different trans-
forms for each 1 mm level of initial misregistration.
For each misregistration level, the results are the mean
values over all transforms that introduced misregistra-
tion of that level. The graph in Fig. 2 shows two al-
ternative views of the results. First, we filtered only
those results that successfully converged under some
reasonable error (here 10 mm – explained below).
Then the graph also plots values that include all re-
sults. The figure also shows the statistics of failures,
i.e. the percentage of cases when the method was not
able to decrease under the error level 10 mm. The
failure happens due to the local solution found during
the optimization or due to the maximum number of
iterations reached (the limit was 120 iterations).
The results can be interpreted such that until the
misregistration is up to about 100 mm, the method
converges to the pixel level precision with at least
90% reliability. As misregistration grows over 100
mm (which is approximately the radius of the vol-
ume), the failure rate increases and method’s perfor-
mance decreases mainly due to cases in which method
converged to some false position. We should point out
that these results and trends do not depend on the spe-
cific value of reasonable error mentioned above. We
use value of 10 mm that is one order higher than the
pixel size and is still reasonable small, but we could
use values 5-45 mm without any significant effect on
the graph.
4.2 Influence of Noise and Rotation Axis
Error on Rotation Estimation
Algorithms for estimation of rotation angle (basic and
improved version described above) were tested for ro-
bustness under non-ideal conditions. First, we want
to examine the influence of these conditions on algo-
rithm’s behaviour and second, we want to justify the
improvement.
In the first part of this experiment, a simulated
MRI brain image (BrainWeb (Collins et al., 1998)
simulated MRI brain image — volume size is 181 ×
217 × 180 with regular 1 mm spacing in all dimen-
sions) was rotated around fixed axis by random an-
gle and Gaussian noise was added to the rotated im-
age. The rotation angle was then recovered by both
basic and improved version of the algorithm and an
absolute difference of estimated and original angle
was measured as an estimation error. We generated
many random angles for each level of noise with a
new instance of noise for each measurement. Graph
3a shows averaged errors over all measurements for
each level of noise.
The second part of this experiment was similar but
instead of adding noise we shifted rotation axis in a
random direction. Hence the algorithms were estimat-
ing rotation around different axis than the image was
originally rotated (note that these algorithms may not
recover original rotation axis). Graph 3b shows de-
pendency of rotation angle error on the distance of
the axis shift (again, error was averaged over many
measurements for each shift distance).
Algorithms proved extreme robustness to noise.
This can be explained due to averaging nature of
PCM: we look for a single peak (ideally delta-
function) in a correlation surface which is result of
an inverse DFT of frequency spectra combined from
the two images. The single peak is kind-of-average
(linear combination) of all frequency samples that are
affected by the same noise as spatial samples of orig-
inal images. The variance of noise is reduced by av-
eraging, hence thanks to large number of samples of
3D volume is the error of estimated rotation angle low
even for really extreme noise.
If the rotation axis is shifted during rotation esti-
mation, the algorithms were able to recover the angle
as long as some structures in the data match. In both
parts of the experiment, it is clearly observable pos-
itive effect of the improvements given in section 3.
The effect of noise is reduced as well as the effect
of disturbances between the two images (shifting the
axis affects mainly the area near rotation axis which
causes most problems in basic version of the algo-
rithm).
3D PHASE CORRELATION USING NON-UNIFORM CYLINDRICAL SAMPLING
257