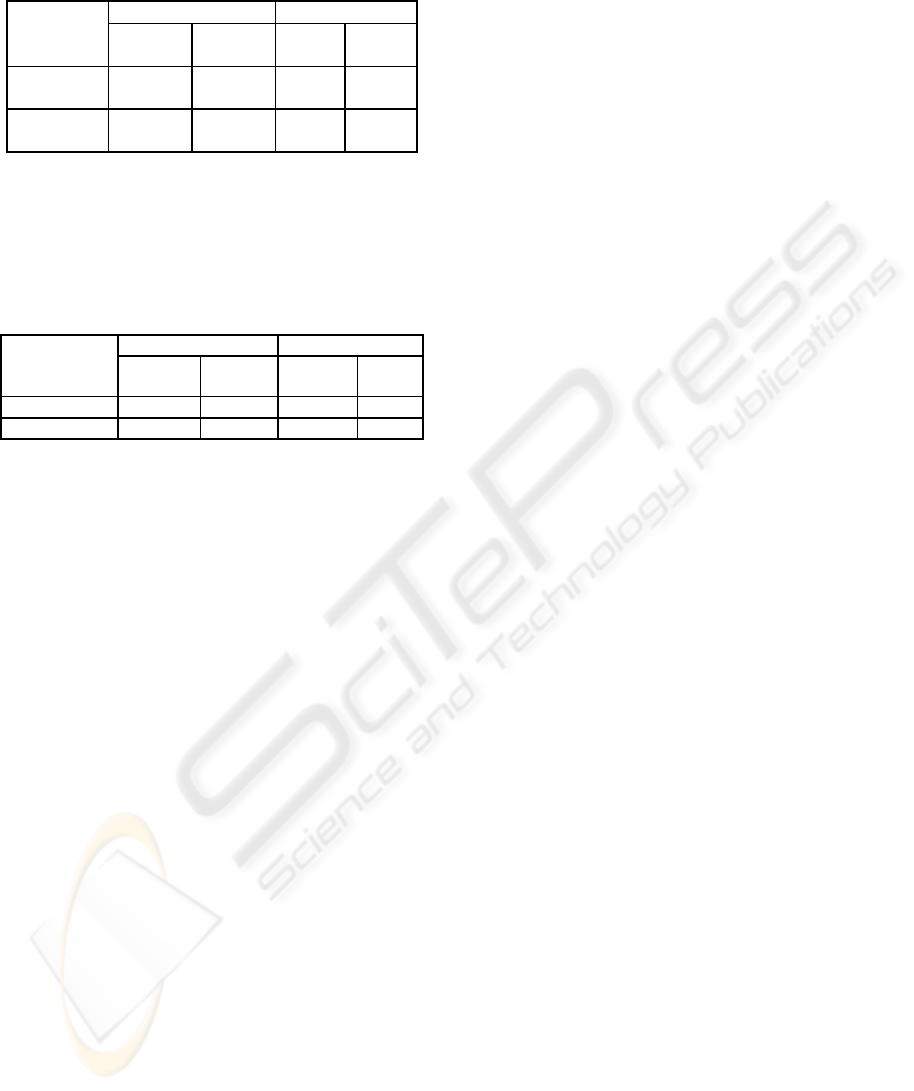
Table 2: Result of using successive difference between
frames and computing FPh and FPv.
nm-05 nm-06
Rank 1 Rank 5 Rank
1
Rank
5
Key frame
differences
68.9% 85.7% 71.2% 83.9%
Successive
differences
74.8% 84% 73.7% 84.7%
mean of each vector from it and then dividing it by
its standard deviation. We obtain considerable
increase in recognition rate when we use mean
vectors.
Table 3: Result of using row mean of frieze patterns
instead of frieze patterns themselves.
6 CONCLUSIONS
In this paper, we introduced one way to recognize
people based on their gait, proposed S. Lee et al.
from the Penn state university. We tried to omit
redundant used features in this algorithm. Then we
applied differences between consecutive images to
extract features instead of computation of difference
between a key frame and other frames. Using these
frames, vertical and horizontal frieze patterns are
computed. In calculation of distance function, mean
value of each row of frieze patterns in form of a
vertical mean vector and a horizontal mean vector
are used. We showed that applying mean vectors is
more successful than direct use of frieze patterns.
We implemented our algorithm and previous
work, on CASIA database. We indicated that our
algorithm has better performance in comparison.
ACKNOWLEDGEMENTS
The authors thank Chinese Academy of Sciences.
Portions of the research in this paper use the CASIA
Gait Database collected by Institute of Automation,
Chinese Academy of Sciences.
REFERENCES
Z. Liu, S. Sarkar, 2004, "Simplest representation yet for
gait recognition: averaged silhouette", Proceedings of
the 17th International Conference on Pattern
Recognition, Vol. 2,704-711.
C. Chen, J. Liang, H. Zhao, H. Hu, 2006, "Gait
recognition using hidden markov model", ICNC, Part
I, LNCS 4221, pp.399-407, Springer.
A. Sundaresun, A. R. Chowdhury, R. Chellappa, 2003, "A
hidden markov model based framewok for recognition
of humans from gait sequences", Proceeding of IEEE
International Conference on Image Processing.
A. Kale, A. Rajagopalan, N. Cuntoor, and V. Kruger,
2002, "Gait-based recognition of humans using
continuous HMMs", Proceeding of the IEEE
Conference on Automatic Face and Gesture
Recognition.
H.I. Suk, B.K. Sink, 2006, "HMM-based gait recognition
with human profiles", SSPR and SPR, LNCS 4109,
pp.596-603.
H. Murase, R. Sakai, 1996, "Moving object recognition in
eigenspace representation: gait analysis and lip
reading", Pattern Recognition Lett.17, 155–162.
J. B. Hayfron-Acquah, M. S. Nixon, J. N. Carter, 2003,
"Automatic gait recognition by symmetry analysis",
Pattern Recognition Letters, ELSEVIER Science B.V.
S. Lee, Y. Liu, and R. Collins, 2007 , "Shape Variation-
based Frieze Pattern for Robust Gait Recognition,"
Proceedings of CVPR 2007, 1-8.
M. S. Nixon, J. Carter, 2006, "Automatic Gait Recognition
by Gait", Proceedings of the IEEE, Vol. 94, No. 11.
CASIA Gait Database,
http://www.sinobiometrics.com
M.P. Murray, A.B. Drought, R.C. kory, 1964, "Walking
Pattern of Normal Men", J. Bone Joint Surg. 46 A(2)
33-360.
G. Johansson, 1973 “Visual Perception of Biological
Motion and a Model for its Analysis”, Perception and
Psychophysics, Vol. 14, No. 2, pp. 201–211.
P.S. Huang, C.J. Harris, M.S. Nixon, 1999, "Human Gait
Recognition in Canonical Space Using Temporal
Templates", IEE Proceeding Computer Vision, Image
& Signal Processing.
L. wang, T. Tan, 2003, "Silhouette Analysis-Base Gait
Recognition for Human Identification", IEEE Trans.
PAMI, 25(12) 1505-1518.
L. Wang,T. Tan, H. Ning, W. Hu, 2004, "Fusion of Static
and Dynamic Body Biometrics for Gait Recognition",
IEEE Trans. Circuit Sys. Video Techno., vol. 14, no.
2, pp. 149-158.
C. BenAbdelkader, 2002, "Gait as a Biometric for Person
Identification in Video Sequences", PhD Dissertation,
University of Maryland, College Park.
nm-05 nm-06
Rank 1 Rank 5 Rank 1
Rank
1
FPh + FPv 74.8% 84% 73.7% 84.7%
MFPh+MFPv 90.7% 95% 87.3% 95.8%
HUMAN GAIT RECOGNITION USING DIFFERENCE BETWEEN FRAMES
331