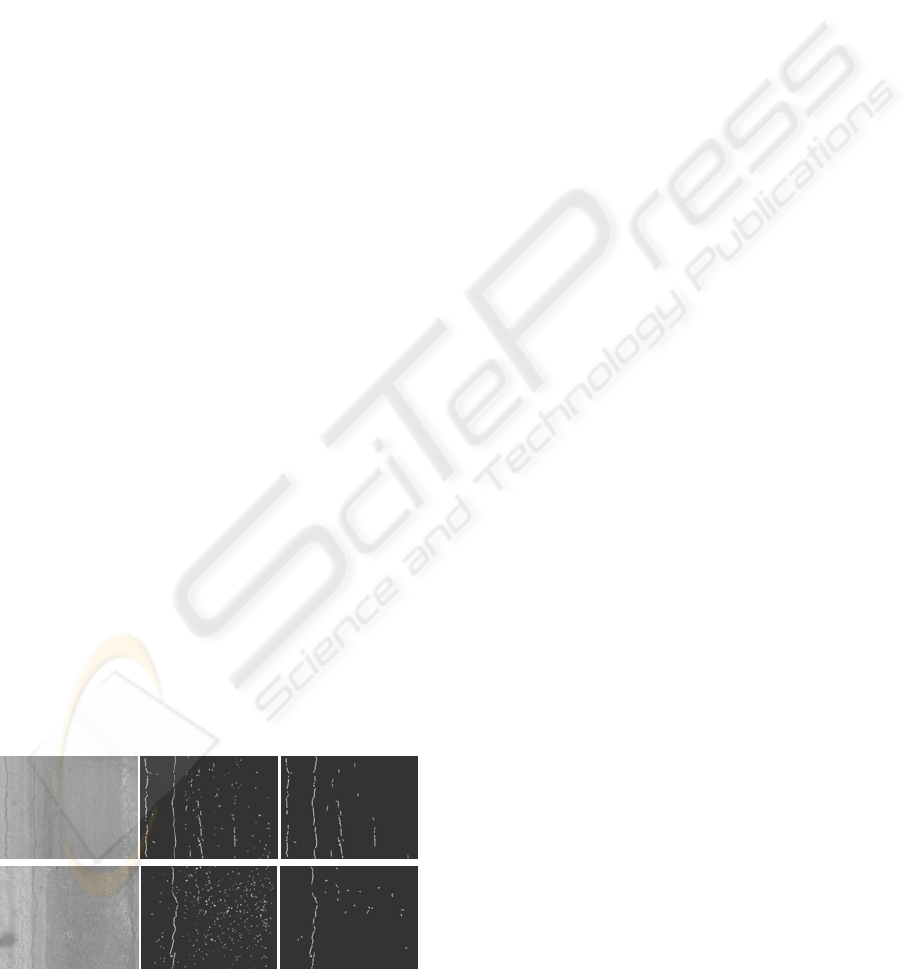
Figure 4, it is on a crack line with an area of 301
pixels. The other significant object information is
shown in Table 1 with their feature values arranged
in descending order. The object (1) is on the same
crack line as the considered object (0). Moreover,
the object (1) is the closest object to the object (0).
Unlike object (1), the object (2) is smaller and
farther than the object (1). As a result, object (1)
gives a value of 1,207.54 which is the highest value
of the gravitational force towards object (0) while
the object (2) gives a value of 147.63 which is a
much smaller value.
Looking at object (3) in the 04, it is the biggest
object but very far away from the considered object
(0). Consequently, it gives a value of 16.85 which is
a small amount of feature value.
0 shows the object where the considered object
(0) is noise object with an area of 61 pixels. Partial
object information is shown in Table 2. Since the
considered object (0) is small, the biggest object
gives a little feature value, 4.46, compared to feature
value of the object (1), which is 1,207.54, in 0.
Due to a high range of the feature value, it has
the ability to distinguish an object as a noise object
or a crack. This concept was applied to the example
images in 0. 0 column (a) shows the original
pavement surface with the crack lines. 0 column (b)
shows the result image with too many noise objects
from crack detection algorithm. After applying this
feature for removing noise, the crack lines appear
obviously. The results are shown in 0 column (c).
6 CONCLUSIONS
This paper introduces an image processing feature
for noise removal in the intermediate result images
of the crack detection algorithm. Unlike normal
noise, noise of this kind of image is unique in terms
of size and dispersal. This feature of noise removal
is based on the theory of universal gravitation. This
theory is applied to the objects for keeping crack
objects separated form noise.
(a) (b) (c)
Figure 7: Result images.
Applying this feature to noisy images, the crack
lines are easier to be notice and classified in the
identification phase. With less noise, the
identification algorithm gives more accurate output
for highway management system. In the big picture,
the proposed method helped improve the accuracy of
the crack detection algorithm (Sorncharean, 2008)
and providing more reliable information to the
highway management system.
REFERENCES
Pynn, J., Wright, A., Lodge, R., 1999. Automatic
identification of cracks in road surfaces. In 7th
International Conference on Image Processing and Its
Applications.
Yu, S.-N., Janga, J.-H., Han, C.-S., 2007. Auto inspection
system using a mobile robot for detecting concrete
cracks in a tunnel. In Automation in Construction.
Subirats, P., Dumoulin, J., Legeay, V., Barba, D., 2006.
Automation of Pavement Surface Crack Detection
using the Continuous Wavelet Transform. In IEEE
International Conference on Image Processing 2006.
Xu, B., Huang, Y., 2006. Automatic Inspection of
Pavement Cracking Distress. In Journal of Electronic
Imaging, vol. 15.
Sorncharean, S., Phiphobmongkol, S., 2008. Crack
Detection on Asphalt Surface Image Using Enhanced
Grid Cell Analysis. In DELTA2008, IEEE
International Symposium on Electronic Design, Test
and Applications.
Zhang, H. G., Wang, Q., 2004. Use of Artificial Living
System for Pavement Distress Survey. In 30th Annual
Conference on Industrial Electronics Society 2004.
IEEE Computer Society.
Tomikawa, T., 1999. A study of road crack detection by
the meta-genetic algorithm. In AFRICON. IEEE.
Meignen, D., Bernadet, M., Briand, H., 1997. One
Application of Neural Networks for Detection of
Defects Using Video Data Bases: Identification of
Road Distresses. In Proceedings of the 8th
International Workshop on Database and Expert
Systems Applications. IEEE Computer Society.
Transportation Information Center, University of
Wisconsin – Madison, 2002. Pavement Surface
Evaluation and Rating Asphalt Roads, PASER
Manual.
Gonzalez, R. C., Woods, R. C., 1992. Digital Image
Processing.
Drakos, N., 1999. Newton's Law of Gravity. Online
Document URL: http://theory.uwinnipeg.ca/
mod_tech/node54.html.
VISAPP 2009 - International Conference on Computer Vision Theory and Applications
272