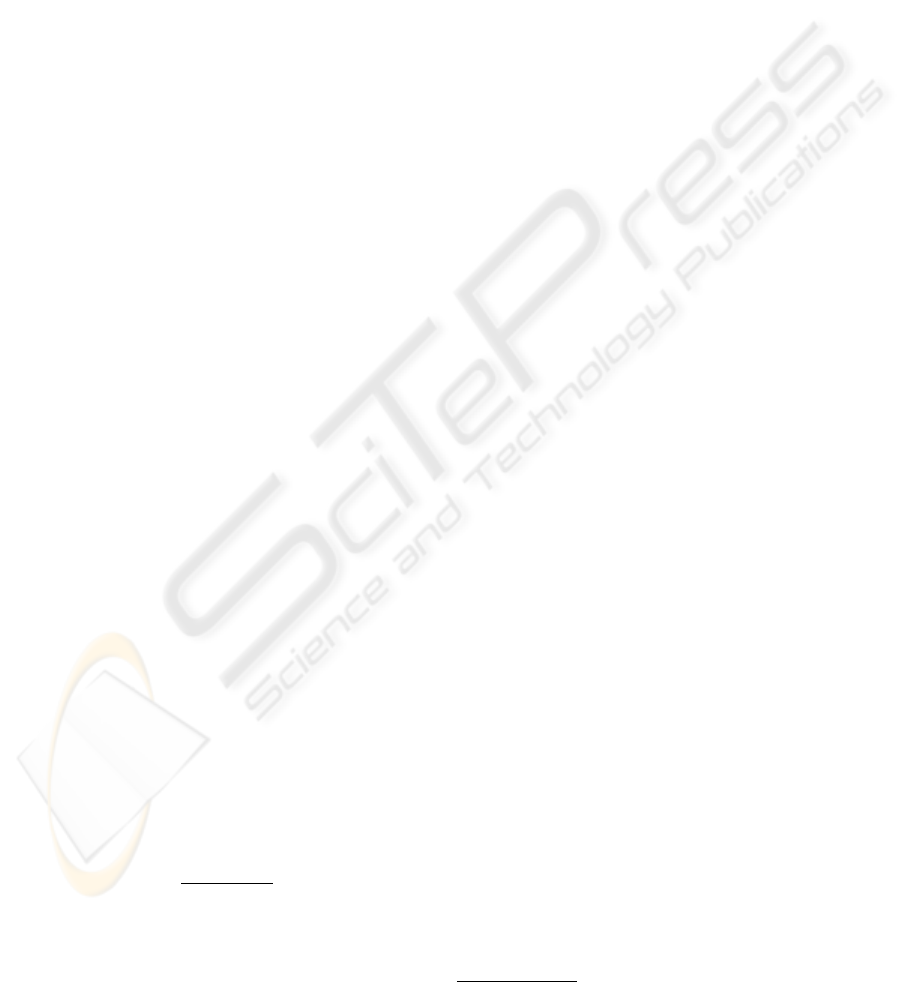
mask. The formfactor is sensitive to both the elonga-
tion of a region and the jaggedness of its border. The
higher the jaggedness of the border, the less the form-
factor. The formfactor is equal to 1 for a circle and is
less for any other shape. Since the final shape of the
segmentation should be close to circle with a regular
border, the formfactor provides a convenient measure
for the confidence of the segmentation.
Since low thresholds can produce a coin segmen-
tation that is near the rectangular shape of the whole
image (providing a comparatively high formfactor), a
segmentation is furthermore only accepted if the area
of the segmented region is lower than 90 % of the
image area. In the case that thresholding produces
more than one connected component in the image,
the one with highest formfactor covering at least 5 %
of the image area is selected. An example for differ-
ent segmentations obtained with different thresholds
is shown in Figure 2. The segmentation obtained with
T
5
= 0.5 shown in Figure 2d has the highest formfac-
tor and is therefore chosen as the final segmentation.
The discretization of T
i
= 0.3, 0.35, ..., 0.6 was
chosen empirically. Tests have shown that a finer
discretization does not improve the accuracy of the
method.
3 EXPERIMENTS
The proposed method was tested on a set of 92 images
acquired at the Kunsthistorisches Museum Vienna,
Austria, the Fitzwilliam Museum, Cambridge UK, and
the Romanian National History Museum representing
a wide range of different coin images. The images
differ in various ways (resolution, background, coin
size relative to image size, illumination conditions).
For the experiments presented here, all color im-
ages were converted to gray-level images. Compres-
sion artifacts due to chroma subsampling are highly
present in the data and make the use of color informa-
tion infeasible.
For each image a ground truth segmentation was
manually obtained by means of a commercial image
editing program. For the evaluation of the segmen-
tation the mutual overlap (MO) (Bowyer, 2000), also
known as dice coefficient, is measured:
MO =
2 · |A
1
∩ A
2
|
|A
1
| + |A
2
|
(3)
where A
1
is the set of pixels in the segmented re-
gion and A
2
the set of pixels in the ground truth seg-
mentation.
To demonstrate the appropriateness of the pro-
posed method for the segmentation of coin images,
the results are compared to the outputs of various
other segmentation methods: (1) the adaptive thresh-
olding method used in (Zaharieva et al., 2007) for
the segmentation of ancient coins, (2) the mean shift
method proposed by (Comaniciu and Meer, 2002) for
a comparison with a state-of-the-art method in image
segmentation and (3) our method when the threshold-
ing is directly applied to gray values instead of the
sum of entropy and gray value range.
It must be noted that the output of the mean
shift segmentation method is not implicitly a parti-
tion into foreground and background, as needed here.
Mean shift partitions the image in a set of disjoint
regions without labeling the foreground and back-
ground. From our point of view the segmentation has
to extract the single most salient object in the image,
which is in our case the coin. Therefore, to make the
mean shift segmentation results comparable, the pa-
rameter M for the minimum allowable region area has
to be manually adapted for each image to produce a
two-segment partition of the image. Evaluation was
performed on the mean shift implementation of the
EDISON system
1
.
In Table 1 the average and median MO for the dif-
ferent methods are listed. The average MO of 0.517
and median MO of 0.720 of the adaptive threshold-
ing method indicate its low robustness. Although the
parameters of the method can be adjusted to perform
well on a given type of coin image it is not able to
handle the wide range of different images contained
in the test set. A second conclusion of the results
is that the local entropy and range filtering is a rea-
sonable preprocessing step to provide a more appro-
priate intensity image for the thresholding. This can
be seen by the lower average and median MO when
the original gray values are used. From the results in
Table 1 it can also be seen that our method achieves
a similar performance than the state-of-the-art mean
shift segmentation. The average MO is equal (0.983)
and the median MO of our method is even higher
(0.993 to 0.988 of the mean shift method). However,
our method has two advantages: firstly, in contrast to
mean shift no parameter has to be adapted manually.
And secondly, our method is computationally faster:
our method (written in MATLAB 7.1) takes 0.38s for
a 178 × 184 image and 8.40s for a 1154 × 866 image,
whereas the means shift implementation (written in
C++) takes 0.73s for the 178 × 184 image and 29.37s
for the 1154 × 866 image on the same machine.
Figure 3 shows results on selected images where
the obtained coin border is outlined by a black or
white line. Figure 3a-c belong to the best segmenta-
1
http://www.caip.rutgers.edu/riul/research/code/EDISON/
index.html, last visited: November 18th 08
ROBUST AUTOMATIC SEGMENTATION OF ANCIENT COINS
275