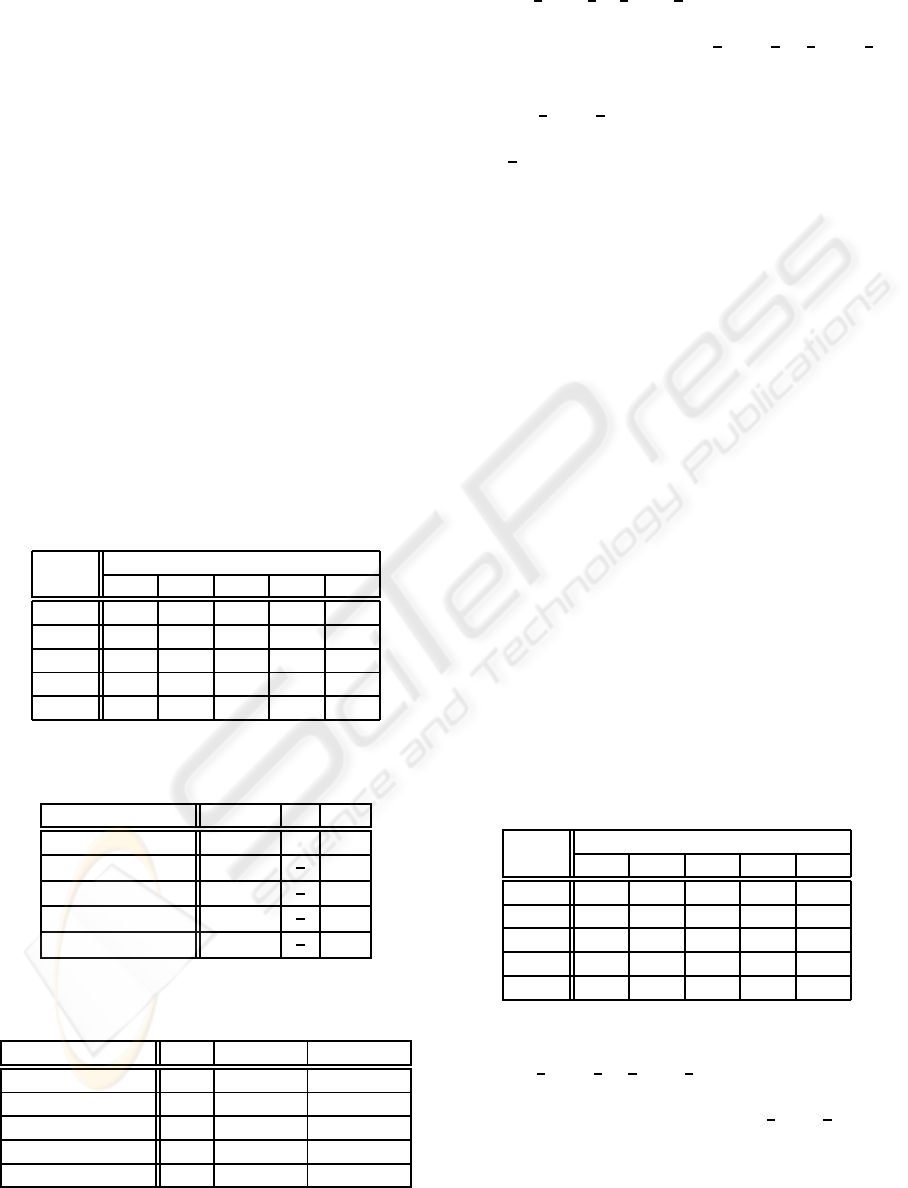
shows 100% recognition rate. Voting method is per-
fect with all five classifiers, but CIAB method is better
than voting method because the CIAB method is per-
fect with at least four classifiers. And, it is recognized
that C-D mutual information tends to show as similar
as the performance of CIAB method, but Q and CD
did not show any meaningful indication on the selec-
tion of classifiers. Therefore, when CIAB method is
used as the combination method of a multiple classi-
fier system, the number of classifiers can be decided
with reference to C-D mutual information of them,
and the number of selected classifiers is four.
The third example, EX-3, supposes that five imag-
inary classifiers showing 60% recognition rate show
recognition results like Table 7 for the five test data.
Classifier sets composed of one to five classifiers are
considered. Under these circumstances, diversity cal-
culations are shown in Table 8 and performance re-
sults are in Table 9. The reason why a classifier set
composed of three classifiers has 100% pl rate as-
sumes that 60% recognition rate is basic for the three
classifiers and for remaining rate a correct classifier is
rightly selected to decide its decision.
Table 7: Recognition result of EX-3 example.
classifier
data Ea Eb Ec Ed Ee
A A B C A A
B B B C D B
C C C C D E
D A D D D E
E A B E E E
Table 8: Diversity result of a classifier set of the EX-3 ex-
ample.
no. of classifiers U(L;E) Q CD
1 0.9503 - -
2 1.3322
1
3
0.8
3 1.6094
1
3
0.8
4 1.6094
1
3
0.6
5 1.6094
1
3
0.6
Table 9: Recognition performance (%) of a classifier set of
the EX-3 example.
no. of classifiers pl voting CIAB
1 60 60 40,60
2 80 40,60,80 60,80,100
3 100 60 100
4 100 100 100
5 100 100 100
From the Table 8, for a classifier set of one clas-
sifier, U(L;E) is approximately computed to 0.9503
(e.g., −(
3
5
∗log
3
5
+
1
5
∗log
1
5
∗2) ≈ 0.9503), for a clas-
sifier set of two classifiers U(L;E) is approximately
computed to 1.3322 (e.g., −(
2
5
∗ log
2
5
+
1
5
∗ log
1
5
∗
3) ≈ 1.3322), and for a classifier set of three classi-
fiers U(L;E) is approximately computed to 1.6094
(e.g., −(
1
5
∗ log
1
5
∗ 5) ≈ 1.6094), by using the real
probability distributions from the data. And, Q statis-
tic is
1
3
and CD is 0.8 in all cases except that the num-
ber of classifier is one. For a classifier set composed
of more than three classifiers, while voting method
shows 60%, CIAB method shows 100% recognition
rate. Voting method is perfect with at least four clas-
sifiers, but CIAB method is better than voting method
because the CIAB method is perfect with at least three
classifiers. And, it is recognized that C-D mutual in-
formation tends to show as similar as the performance
of CIAB method, but Q and CD did not show any
meaningful indication on the selection of classifiers.
Therefore, when CIAB method is used as the com-
bination method of a multiple classifier system, the
number of classifiers can be decided with reference to
C-D mutual information of them, and the number of
selected classifiers is three.
The fourth example, EX-4, supposes that five
imaginary classifiers showing 80% recognition rate
show recognition results like Table 10 for the five test
data. Classifier sets composed of one to fiveclassifiers
are considered. Under these circumstances, diversity
calculations are shown in Table 11 and performance
results are in Table 12. The reason why a classifier
set composed of two classifiers has 100% pl rate as-
sumes that 60% recognition rate is basic for the two
classifiers and for remaining rate a correct classifier is
rightly selected to decide its decision.
Table 10: Recognition result of EX-4 example.
classifier
data Ea Eb Ec Ed Ee
A A B A A A
B B B C B B
C C C C D C
D D D D D E
E A E E E E
From the Table 11, for a classifier set of one clas-
sifier, U(L;E) is approximately computed to 1.3322
(e.g., −(
2
5
∗ log
2
5
+
1
5
∗ log
1
5
∗ 3) ≈ 1.3322), and for
a classifier set of two classifiers U(L;E) is approxi-
mately computed to 1.6094 (e.g., −(
1
5
∗ log
1
5
∗ 5) ≈
1.6094), by using the real probability distributions
from the data. And, Q statistic is -1 and CD is 1 in
all cases except that the number of classifier is one.
For a classifier set composed of more than two clas-
VISAPP 2009 - International Conference on Computer Vision Theory and Applications
360