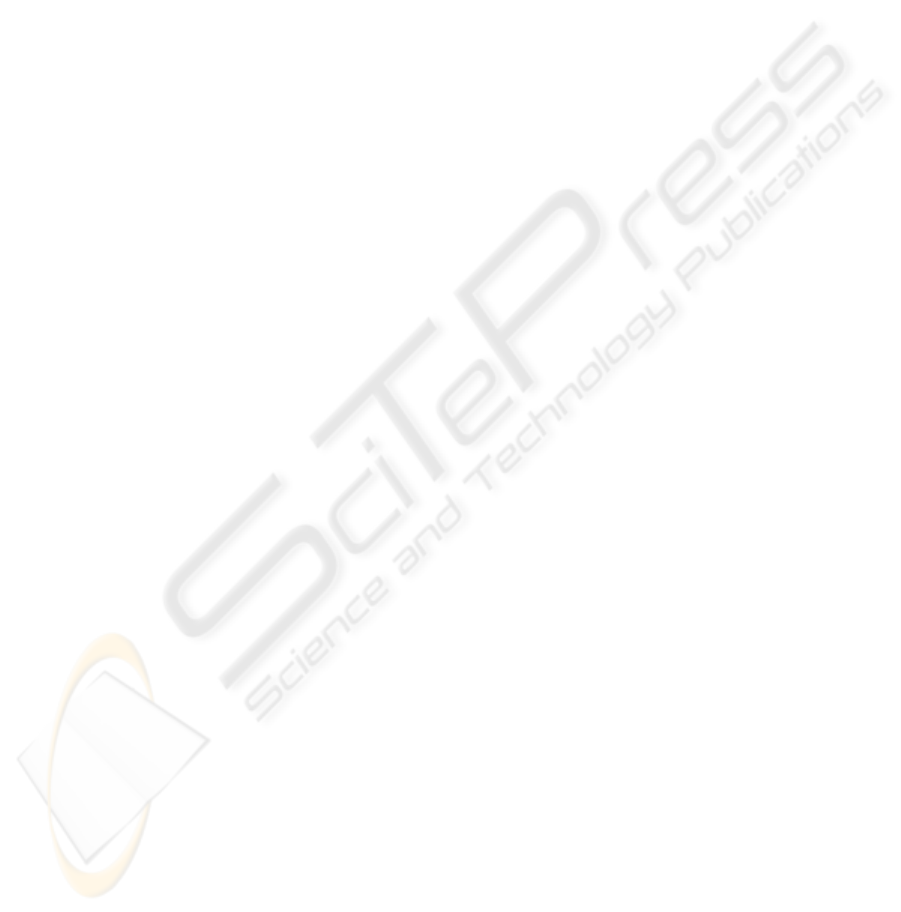
gium). This paper presents research results of the
Belgian Network BIOMAGNET (Bioinformatics and
Modeling: from Genomes to Networks), funded by
the Interuniversity Attraction Poles Programme, initi-
ated by the Belgian State, Science Policy Office. The
scientific responsibility rests with the authors.
REFERENCES
Amit, Y. and Geman, D. (1997). Shape quantization and
recognition with randomized trees. Neural computa-
tion, 9:1545–1588.
Bertelli, L., Byun, J., and Manjunath, B. S. (2007). A varia-
tional approach to exploit prior information in object-
background segregation: application to retinal images.
In ICIP.
Borenstein, E. and Malik, J. (2006). Shape guided object
segmentation. In CVPR, pages 969–976.
Bosch, A., Zisserman, A., and Munoz, X. (2007). Image
classification using random forests and ferns. In Proc.
ICCV.
Breiman, L., Friedman, J., Olshen, R., and Stone, C. (1984).
Classification and Regression Trees. Wadsworth and
Brooks, Monterey, CA.
Cour, T. and Shi, J. (2007). Recognizing objects by piecing
together the segmentation puzzle. In CVPR.
Dollar, P., Tu, Z., and Belongie, S. (2006). Supervised
learning of edges and object boundaries. In CVPR,
pages 1964–1971.
Dumont, M., Mar´ee, R., Wehenkel,
L., and Geurts, P. (2009).
http://www.montefiore.ulg.ac.be/services/stochastic/
pubs/2009/DMWG09/.
Ernst, D., Mar´ee, R., and Wehenkel, L. (2006). Reinforce-
ment learning with raw image pixels as input state.
In Proc. International Workshop on Intelligent Com-
puting in Pattern Analysis/Synthesis, volume 4153 of
Lecture Notes in Computer Science, pages 446–454.
Geurts, P., Ernst, D., and Wehenkel, L. (2006a). Extremely
randomized trees. Machine Learning, 36(1):3–42.
Geurts, P., Wehenkel, L., and d Alch´e-Buc, F. (2006b). Ker-
nelizing the output of tree-based methods. In ICML,
pages 345–352.
He, X., Zemel, R., and Carreira-Perpinan, M. (2004). Mul-
tiscale conditional random fields for image labelling.
In CVPR, volume 2, pages 695–702.
He, X., Zemel, R., and Ray, D. (2006). Learning and in-
corporating top-down cues in image segmentation. In
ECCV, pages 338–351.
Jodogne, S., Briquet, C., and Piater, J. (2006). Approxi-
mate policy iteration for closed-loop learning of vi-
sual tasks. In Proc. of the 17th European Conference
on Machine Learning, volume 4212, pages 222–226.
Lepetit, V. and Fua, P. (2006). Keypoint recognition us-
ing randomized trees. IEEE Transactions on Pattern
Analysis and Machine Intelligence, 28(9):1465–1479.
Mar´ee, R., Geurts, P., Piater, J., and Wehenkel, L. (2005).
Random subwindows for robust image classification.
In CVPR, volume 1, pages 34–40.
Mar´ee, R., Geurts, P., Visimberga, G., Piater, J., and We-
henkel, L. (2003). An empirical comparison of ma-
chine learning algorithms for generic image classifi-
cation. In Proc. SGAI-AI, pages 169–182.
Mar´ee, R., Geurts, P., and Wehenkel, L. (2007). Content-
based image retrieval by indexing random subwin-
dows with randomized trees. In Proc. ACCV, volume
4844 of LNCS, pages 611–620.
Meurie, C., Lebrun, G., Lezoray, O., and Elmoataz, A.
(2003). A comparison of supervised pixels-based
color image segmentation methods. application in
cancerology. WSEAS Transactions on computers, spe-
cial issue on SSIP’03, 2:739–744.
Moosmann, F., Triggs, B., and Jurie, F. (2006). Randomized
clustering forests for building fast and discriminative
visual vocabularies. In Neural Information Processing
Systems (NIPS).
Nowak, E. and Jurie, F. (2007). Learning visual similarity
measures for comparing never seen objects. In Proc.
IEEE CVPR.
Nowak, E., Jurie, F., and Triggs, B. (2006). Sampling strate-
gies for bag-of-features image classification. In Proc.
ECCV, pages 490–503.
Philbin, J., Chum, O., Isard, M., Sivic, J., and Zisserman, A.
(2007). Object retrieval with large vocabularies and
fast spatial matching. Proc. IEEE CVPR.
Schroff, F., Criminisi, A., and Zisserman, A. (2008). Object
class segmentation using random forests. In Proc. of
the British Machine Vision Conference (BMVC).
Shotton, J., Johnson, M., and Cipolla, R. (2008). Semantic
texton forests for image categorization and segmenta-
tion. In Proc. IEEE Conference on Computer Vision
and Pattern Recognition (CVPR).
Shotton, J., Winn, J., Rother, C., and Criminisi, A. (2006).
Textonboost: Joint appearance, shape and context
modeling for multi-class object recognition and seg-
mentation. In ECCV, pages I: 1–15.
Shotton, J., Winn, J., Rother, C., and Criminisi, A. (2007).
Textonboost for image understanding: Multi-class ob-
ject recognition and segmentation by jointly modeling
appearance, shape and context. In International Jour-
nal on Computer Vision.
Verbeek, J. and Triggs, B. (2007). Scene segmentation with
conditional random fields learned from partially la-
beled images. In Proc. NIPS.
Yin, P., Criminisi, A., Winn, J., and Essa, I. (2007). Tree-
based classifiers for bilayer video segmentation. In
Proc. CVPR.
Yu, S. X. and Shi, J. (2003). Object-specific figure-ground
segregation. In CVPR, volume 2, pages 39–45.
FAST MULTI-CLASS IMAGE ANNOTATION WITH RANDOM SUBWINDOWS AND MULTIPLE OUTPUT
RANDOMIZED TREES
203