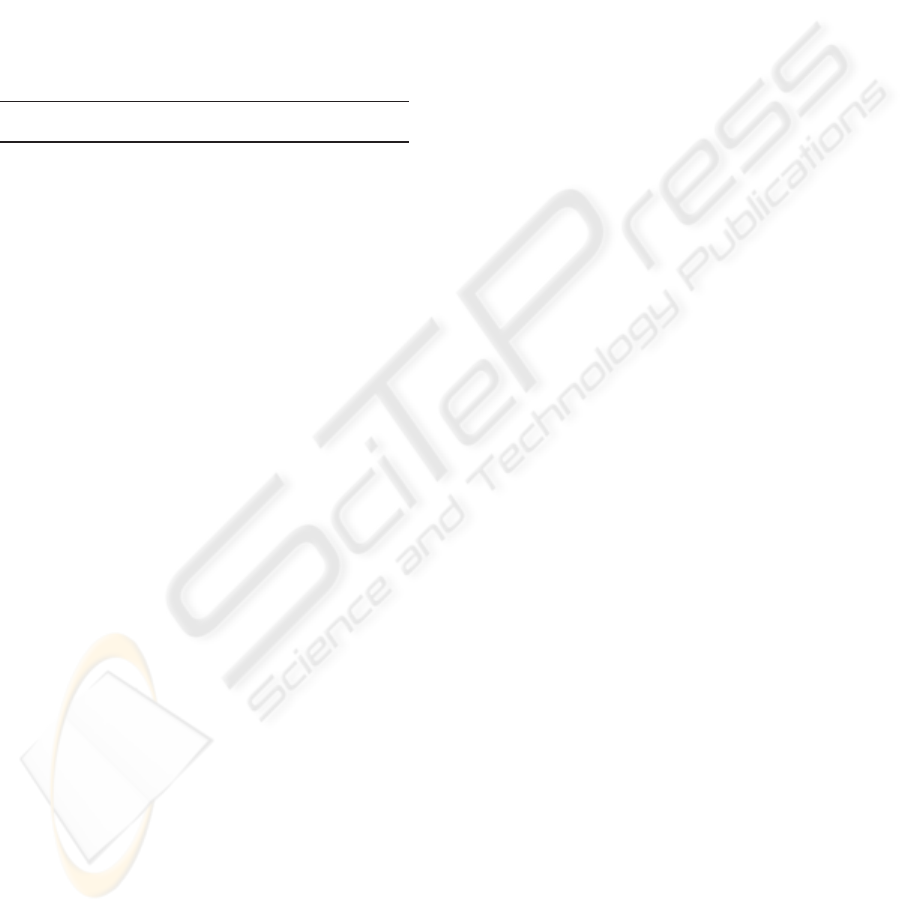
below havebeen carried out on the sequence database.
Table 2 consider the FR performance with or without
tracking and presents the classification results. For
each sequence, these results are compared to tracking
results in terms of FAR (False Acceptance Rate) and
FRR (False Rejection Rate). To be more consistent,
the only images involving face detection have been
taken into account. We note that the runs involving
tracking are more robust to environmental changes,
mainly due to spatio-temporal effects.
Table 2: Face classification performance for the database
image subset involving detected frontal faces.
Face classif. without tracking with tracking
FAR 35.09% 26.47% (σ = 1.97%)
FRR 60.22% 25.73% (σ = 0.25%)
6 CONCLUSIONS AND
PERSPECTIVES
This paper presented the development of a still-image
FR system dedicated to Human/Robot interaction in
a household framework. The main contribution is the
improvement of the known FR algorithms thanks to a
genetic algorithm for free-parameter optimization.
Off-line evaluations on sequences acquired from
the robot show that the overall system enjoys the valu-
able capabilities: (1) efficiency of the recognition pro-
cess against face pose changing, (2) robustness to il-
lumination changes. Eigenface subspace and SVM
makes it possible to avoid misclassification due to the
environment while NSGA-II improves the FR pro-
cess. Moreover, the fusion of FR outputs in the track-
ing loop enables the overall system to be more robust
to natural and populated settings.
Several directions are studied regarding our still-
image FR system. A first line of investigation con-
cerns the fusion of heterogeneous information such
as RFID or sound cues in order to keep the identifi-
cation process more robust to the environment. De-
tection of an RFID tag worn by individuals will allow
us to drive the camera thanks to a pan-tilt unit and
so trigger tracker initialization, and will contribute as
another measurement in the tracking loop. The sound
cue will endow the tracker with the ability to switch
its focus between known speakers.
REFERENCES
Adini, Y., Moses, Y., and Ullman, S. (1997). Face recog-
nition: the problem of compensating for changes in
illumination direction. 19(7):721–732.
Arulampalam, S., Maskell, S., Gordon, N., and Clapp, T.
(2002). A tutorial on particle filters for on-line non-
linear/non-gaussian bayesian tracking. Trans. on Sig-
nal Processing, 2(50):174–188.
Belhumeur, P., Hespanha, J., and Kriegman, D. (1996).
Eigenfaces vs. fisherfaces. In European Conf. on
Computer Vision (ECCV’96), pages 45–58.
Gavrila, D. and Munder, S. (2007). Multi-cue pedestrian
detection and tracking from a moving vehicle. Int.
Journal of Computer Vision (IJCV’07), 73(1):41–59.
Germa, T., Br`ethes, L., Lerasle, F., and Simon, T. (2007).
Data fusion and eigenface based tracking dedicated to
a tour-guide robot. In Int. Conf. on Vision Systems
(ICVS’07), Bielefeld, Germany.
Heseltine, T., Pears, N., and Austin, J. (2002). Evaluation of
image pre-processing techniques for eigenface based
recognition. In SPIE: Image and Graphics, pages
677–685.
Isard, M. and Blake, A. (1998). I-CONDENSATION: Uni-
fying low-level and high-level tracking in a stochastic
framework. In European Conf. on Computer Vision
(ECCV’98), pages 893–908.
Jonsson, K., Matas, J., Kittler, J., and Li, Y. (2000). Learn-
ing support vectors for face verification and recogni-
tion. In Int. Conf. on Face and Gesture Recognition
(FGR’00), pages 208–213, Grenoble, France.
Lam, K. and Yan, H. (98). An analytic-to-holistic approach
fo face recognition based on a single frontal view.
7(20):673–686.
Lee, K., Ho, J., Yang, M., and Kriegman, D. (2003). Video-
based face recognition using probabilistic appearance
manifolds. Computer Vision and Pattern Recogni-
tion, 2003. Proceedings. 2003 IEEE Computer Society
Conference on, 1:I–313–I–320 vol.1.
P´erez, P., Vermaak, J., and Blake, A. (2004). Data fusion for
visual tracking with particles. Proc. IEEE, 92(3):495–
513.
Provost, F. and Fawcett, T. (2001). Robust classifica-
tion for imprecise environments. Machine Learning,
42(3):203–231.
Seo, K. (2007). A GA-based feature subset selection and
parameter optimization of SVM for content-based im-
age retrieval. In Int. Conf. on Advanced Data Min-
ing and Applications (ADMA’07), pages 594–604,
Harbin, China.
Shan, S., Gao, W., and Zhao, D. (2003). Face recognition
based on face-specific subspace. Int. Journal of Imag-
ing Systems and Technology, 13(1):23–32.
Turk, M. and Pentland, A. (1991). Face recognition using
eigenfaces. In Int. Conf. on Computer Vision and Pat-
tern Recognition (CVPR’91), pages 586–591.
Viola, P. and Jones, M. (2003). Fast multi-view face de-
tection. In Int. Conf. on Computer Vision and Pattern
Recognition (CVPR’03).
Wu, T., Lin, C., and Weng, R. (2004). Probability esti-
mates for multi-class classification by pairwise cou-
pling. Journal of Machine Learning Research, 5:975–
1005.
A TUNING STRATEGY FOR FACE RECOGNITION IN ROBOTIC APPLICATION
521