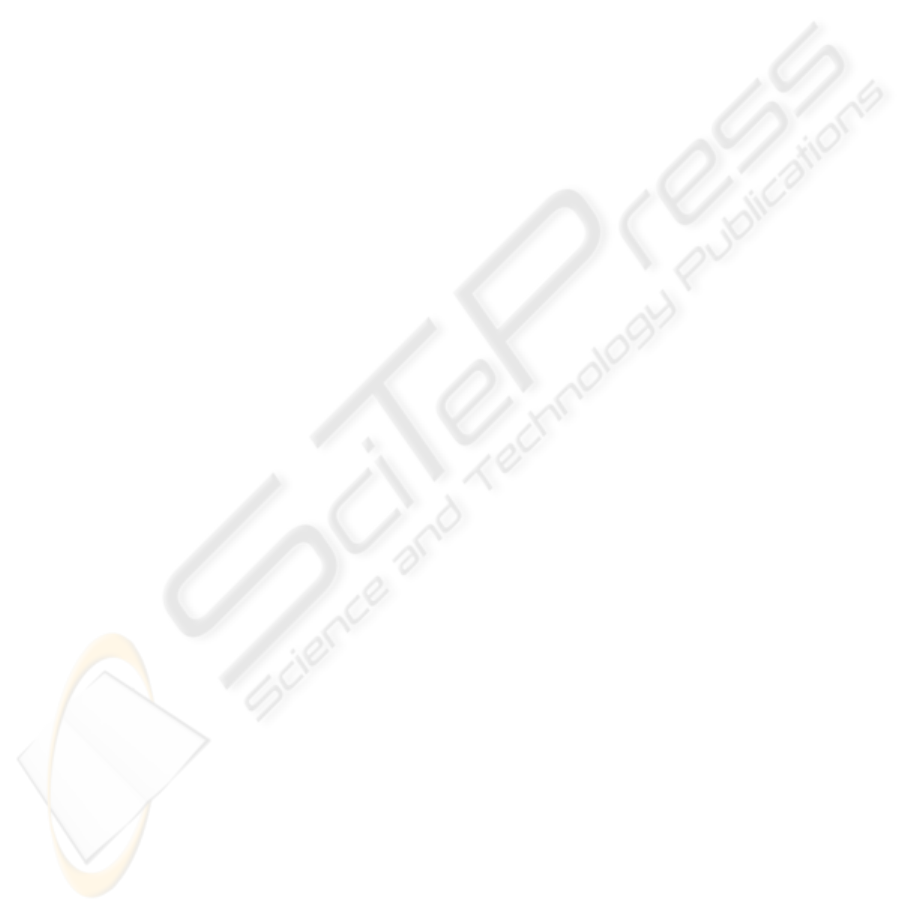
GRAMGE equation discovery tool used here with a
range of grammars describing the possible syntax
(functional terms, dependencies between variables,
maximum complexity) of each equation. The recur-
sive empirical models produced as a result are tested
on their ability to (1) predict the next state of the sys-
tem from past observations for the data used to learn
the model (i.e., using training data to measure the “in-
sample” error), (2) predict the next state from past ob-
servations for data not used in the training (using the
test data to measure “out-of-sample” error), and, fi-
nally, (3) forecast the future of the system (parameter
in question) starting from the last observation used in
training, and recursively using the model’s own pre-
dictions to look another step ahead in time. The re-
sults suggest that the empirical approach is able to re-
produce the results of other approaches, when simi-
larly constrained, and go further to produce complex
nonlinear models able to forecast inflation over con-
siderable periods of time.
2 BACKGROUND
2.1 Empirical Modelling of the Inflation
Process
In addition to the observed decline in inflation volatil-
ity in recent years, the inflation had grown increas-
ingly disconnected from other macro variables. The
inflation process can be modelled as a function of its
own history, in which the possibility of a time trend
is also taken into account. Linear estimations of that
kind are known to produce forecasts that are hard to
outperform in terms of out of sample accuracy. There-
fore, the first model to consider here is a univariate
autoregressive model of the general form:
π
t
= f (π
t−1
, π
t−2
. . . π
t−k
, t) (1)
where π
t
is the inflation rate and t is time.
This type of modelling does not provide, however,
a satisfactory understanding on the co-movement and
dependence between the nominal and the real side of
the economy (i.e., inflation and output) and how these
are influenced by monetary policy (interest rates).
Economic theory suggests that inflation is linked to
output y (Eq. 3), more specifically, it rises when
output increases over a certain level, a relationship
known as the Phillips curve. Similarly, output is cor-
related with the interest rate r (Eq. 2), and is expected
to rise when interest rates are lowered, a relationship
known as the IS (Investment and Saving) curve (Blan-
chard, 2000). Monetary policy is supposed to react to
inflation, as well as the state of the economy mea-
sured through its output, which is a relationship re-
flected in Eq. 4. Here the most common approach is
to model these three equations as linear functions. It is
suggested though that due to the constantly evolving
structure of the economy, a linear specification could
fail in capturing those relationships and might under-
estimate their value for forecasting.
y
t
= f (y
t−1
, y
t−2
, ...r
t
, r
t−1
, ...t) (2)
π
t
= f (π
t−1
, π
t−2
, ...y
t
, y
t−1
, ...t) (3)
r
t
= f (π
t
, π
t−1
, ...y
t
, y
t−1
, ..., t) (4)
2.2 Machine Learning, Equation
Discovery and LAGRAMGE
Machine Learning (ML) aims at describing the prop-
erties of a set of observations from a given source,
and/or making predictions about the nature of future
observations from the same source. Both goals are
achieved by changing the representation of available
data as expressed in its original form (or object lan-
guage) into another representation (using another for-
malism, known as hypothesis language). The new
representation copies closely the information encoded
in the original data, but is usually more general, and
allows statements to be made about yet unseen cases.
ML can be seen as the search for a mapping from a set
of inputs to an output; this mapping is often a func-
tion. In the context of searching for macroeconomic
models, this means functional relationships between
the observed variables can be determined.
No ML algorithm can make predictions unless it
employs a bias (Mitchell, 1997). In general, the bias
will restrict the range of possible functions (models,
hypotheses) that can be described by the hypothe-
sis language. For instance, the set of data points
{(0, 0), (π, 0), (2π, 0)} can be modelled by the func-
tions y = 0, y = cos x or y = x(x − π)(x − 2π), de-
pending on the bias, which may restrict the hypothesis
to a linear, trigonometric or polynomial function.
Such a bias is also called language bias to distin-
guish it from the preference bias, allowing a choice
between alternative models with equal coverage of
the available data. Here some simple, but general
principles (heuristics) are often employed. For in-
stance, Occam’s razor (Mitchell, 1997) favours the
simplest hypothesis language, while the Minimal De-
scription Length (MDL) bias (Rissanen, 1978) sug-
gests a trade-off between the complexity of the hy-
pothesis language and that of the resulting represen-
tation of the data.
The area of ML focusing on the search for quanti-
tative laws, expressed as equations, is known as equa-
EQUATION DISCOVERY FOR MACROECONOMIC MODELLING
319