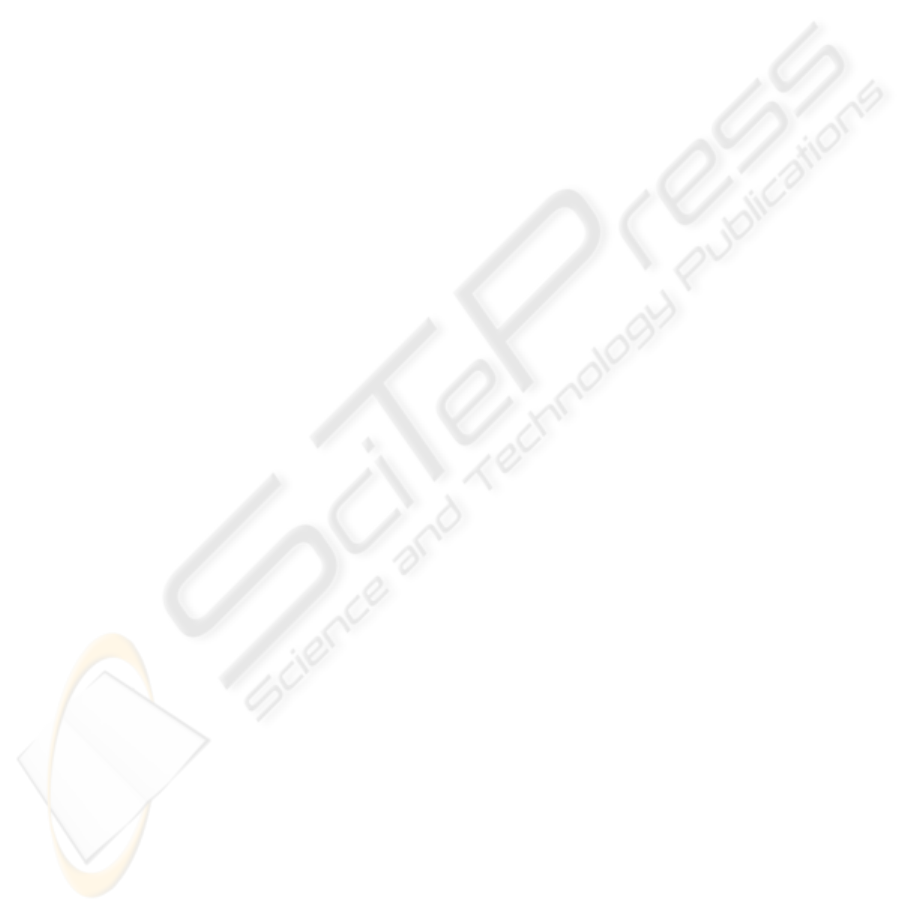
precisely even part of the object has moved out of
the image. In Fig. 7 & Fig. 8, tracking with
significant object appearance variation is tested. In
the two experiments, vehicles’ visible parts change
with its changing steering angel and view point; and
the shadow in Fig. 7 and highlight in Fig. 8 were
cast onto the vehicles when they move into the upper
part, they all bring significant appearance variations
to vehicles. The feature set for GMM updating
becomes sparse as the number of patches decreases
with vehicle’s decreasing scale. But in both
experiments the proposed method tracks the vehicle
accurately with correct pose (scaling & rotation)
estimate.
4 CONCLUSIONS
In this paper, to solve the above limitations in FDA
based object representation method in tracking, we
propose a robust multi-scale FDA object
representation method for tracking, and provide the
robust FDA updating method. Scale robustness is
achieved by both robust feature extraction and
robust density estimation. The Bayesian model
updating method is proposed using model prior
knowledge extracted from historical model.
Experiment shows the effectiveness of the method.
Our future directions could include explorations to
different features and tracking by multiple feature
fusion. Finally the aim is to develop it to a multiple
interacting objects tracking method.
REFERENCES
Arulampalam M. S., Maskell S., Gordon N., Clapp T.,
2002. “A tutorial on particle filters for online
nonlinear/non-Gaussian Bayesian tracking”. IEEE
Transactions on Signal Processing, Vol. 50(2),
pp.174-188.
Carreira-Perpinan M.A., 2007. “Gaussian Mean-Shift Is an
EM Algorithm”. IEEE Transactions on Pattern
Analysis and Machine Intelligence, Vol. 29(5), pp.767
– 776.
Comaniciu D., Meer P., 2002. “Mean Shift: A Robust
Approach toward Feature Space Analysis”, IEEE
Transactions on Pattern Analysis and Machine
Intelligence, Vol. 24(5), pp. 603-619.
Comaniciu D., Ramesh V., Meer P., 2003. “Kernel based
object tracking”. IEEE Transactions on Pattern
Analysis and Machine Intelligence, Vol. 25(5), pp. 564
– 577.
Dempster A. P., Laird N. M., Rubin D. B., 1977.
"Maximum Likelihood from Incomplete Data via the
EM Algorithm". Journal of the Royal Statistical
Society, Series B, Vol. 39, No. 1, pp.1-38.
Gamerman D., 1997. “Markov chain Monte Carlo:
stochastic simulation for Bayesian inference”.
CHAPMAN & HALL/CRC.
Gauvain J. L., Lee C.H., 1994. “Maximum a Posteriori
Estimation for Multivariate Gaussian Mixture
Observations of Markov Chains”. IEEE Transactions
on Speech and Audio Processing, 2(2):291-298.
Goldberger J., Greenspan H., 2006. “Context-based
segmentation of image sequences”. IEEE Transactions
on Pattern Analysis and Machine Intelligence, Vol.
28(3), pp. 463 – 468.
Han B., Comaniciu D., Zhu Y., Davis L., 2008.
“Sequential Kernel Density Approximation and Its
Application to Real-Time Visual Tracking”. IEEE
Transactions on Pattern Analysis and Machine
Intelligence, Vol. 30(7), pp. 1186 – 1197.
Jepson A. D., D. Fleet J., El-Maraghi T. F., 2003. “Robust
online appearance models for visual tracking”. IEEE
Transactions on Pattern Analysis and Machine
Intelligence, Vol. 25(10), pp.1296- 1311.
Lindeberg T., 1994. “Scale-Space Theory in Computer
Vision”. Kluwer Academic Publishers, Dordrecht, the
Netherlands.
Liu C.Y., Yung N.H.C., 2008. “Multi-scale feature density
approximation for object representation and tracking”.
IASTED Signal Processing, Pattern Recognition and
Applications.
Raja Y., Mckenna S. J., Gong S., 1999. “Tracking color
objects using adaptive mixture models”. Image and
Vision Computing, Vol. 17(3-4), pp.225–231.
Timor K., Michael B.
,2001. “Saliency, Scale and Image
Description”, International Journal of Computer
Vision, 45(2), 83-105.
Yu T., Wu Y., 2006. “Differential Tracking based on
Spatial-Appearance Model (SAM)”, Proceedings of
the IEEE CVPR2006, Vol.1(17-22), pp.720-727, New
York City, NY.
VISAPP 2009 - International Conference on Computer Vision Theory and Applications
540