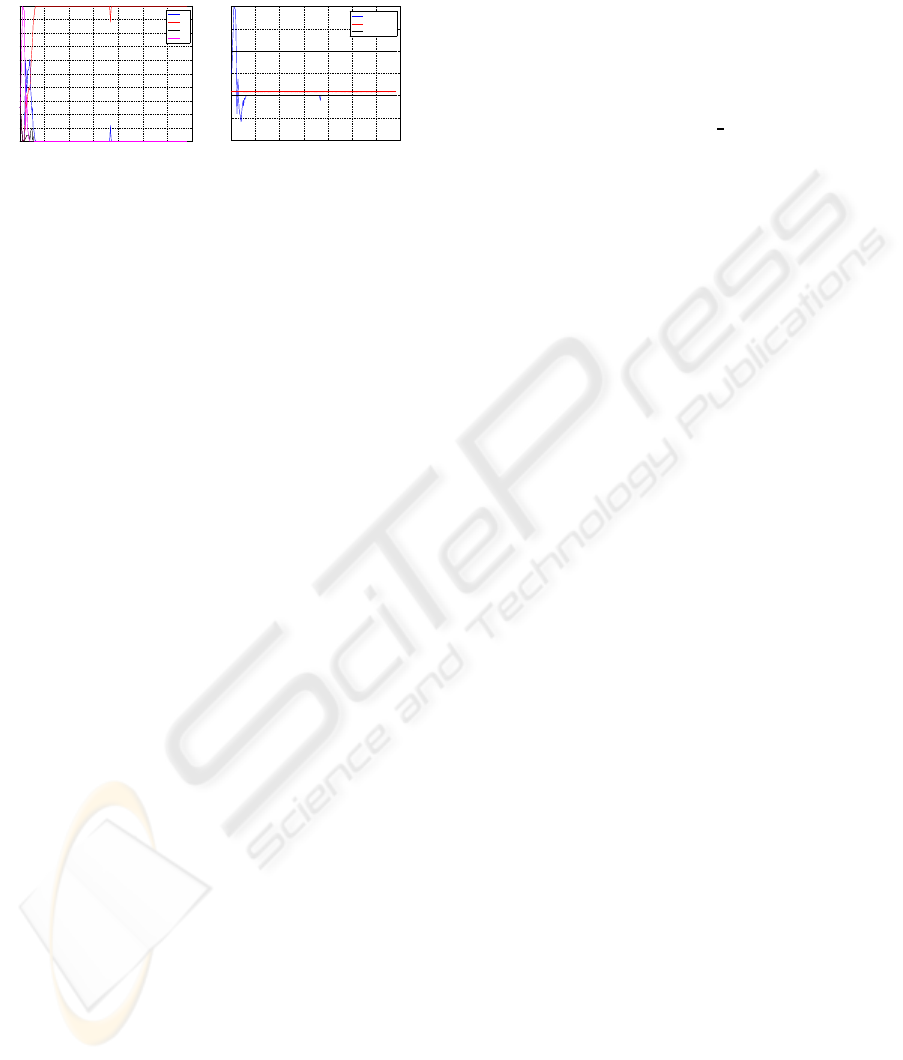
on the left panel of Fig. 6. On the right panel of that
figure, the real and estimated angular velocities are
plotted.
0 20 40 60 80 100 120 140
0
0.1
0.2
0.3
0.4
0.5
0.6
0.7
0.8
0.9
1
probabilidades
t [s]
p1
p2
p3
p4
0 20 40 60 80 100 120 140
0
0.01
0.02
0.03
0.04
0.05
0.06
w [º/s]
t [s]
w estimado
w real
4 modelos
Figure 6: MMAE evolution over time. On the left, the a
posteriori hypothesis probabilities. On the right, real (red)
and estimated (blue) target angular velocity.
In what concerns the range of operation for the
proposed system, it depends significantly on the cam-
era used and on the size of the target to be tracked. In
the experiments reported, an elliptic shape with axes
of length 106mm and 145mm, was identified and lo-
cated, with the mentioned accuracies for distances up
to approximately 7m from the camera. The lower
bound of the range of distances in which the appli-
cation works properly, is limited by the distance at
which the target stops being completely visible, filling
the camera field of vision. For the target considered,
this occurs at distances bellow 40cm.
7 CONCLUSIONS AND FUTURE
WORK
A new architecture for indoor positioning and track-
ing is presented, supported on suboptimal stochastic
multiple-model adaptive estimation techniques. The
proposed approach was implemented using a single
low cost pan and tilt camera, estimating the real time
location of a target which moves in the 3D real world
with accuracies on the order of 20cm.
The main limitations of the implemented system
are the required knowledge on the target dimensions,
and the inability to identify targets with colors other
than red.
In the near future, an implementation of the de-
veloped architecture in C will be pursued, which will
allow for the tracking of more unpredictable targets.
Also, an extension of the proposed architecture to a
multiple camera based system is thought. Distances
to targets will then be computed resorting to triangu-
lation methods, thus avoiding the requirement on the
precise knowledge of their dimensions.
Finally, it is also advised the integration of a sen-
sor in the vision system that retrieves camera orienta-
tion in each time instant, and the implementation of
an image segmentation algorithm that can identify a
wider variety of targets.
ACKNOWLEDGEMENTS
This work was partially supported by Fundac¸˜ao
para a Ciˆencia e a Tecnologia (ISR/IST plurian-
ual funding) through the POS Conhecimento Pro-
gram that includes FEDER funds and by the project
PDCT/MAR/55609/2004 - RUMOS of the FCT.
REFERENCES
Athans, M. and Chang, C. (1976). Adaptive Estimation
and Parameter Identification using Multiple Model
Estimation Algorithm. MIT Lincoln Lab., Lexington,
Mass.
Bar-Shalom, Y., Rong-Li, X., and Kirubarajan, T. (2001).
Estimation with Applications to Tracking and Naviga-
tion: Theory Algorithms and Software. John Wiley &
Sons, Inc.
Borenstein, J., Everett, H. R., and Feng, L. (1996). Where
am I? Sensors and Methods for Mobile Robot Posi-
tioning. Editado e compilado por J. Borenstein.
Faugeras, O. and Luong, Q. (2001). The geometry of multi-
ples images. MIT Press.
Gaspar, T. (2008). Sistemas de seguimento para aplicac¸˜oes
no interior. Master’s thesis, Instituto Superior
T´ecnico.
Gelb, A. (2001). Applied Optimal Estimation. MIT Press,
Cambridge, Massachusetts.
Kass, M., Witkin, A., and Terzopoulos, D. (1987). Snakes:
Active contour models. Int. J. Comput. Vis., 1:321–
331.
Kolodziej, K. and Hjelm, J. (2006). Local Positioning Sys-
tems: LBS Applications and Services. CRC Press.
Leymarie, F. and Levine, M. D. (1993). Tracking de-
formable objects in the plane using an active contour
model. IEEE Trans. Pattern Anal. Machine Intell.,
15:617–634.
Li, X. R. and Jilkov, V. P. (2003). Survey of maneuvering
target tracking. part i: Dynamic models. IEEE Trans-
actions on Aerospace and Electronic Systems, pages
1333–1364.
McInerney, T. and Terzopoulos, D. (1995). A dynamic finite
element surface model for segmentation and tracking
in multidimensional medical images with application
to cardiac 4d image analysis. Comput. Med. Imag.
Graph, 9:69–83.
Terzopoulos, D. and Fleischer, K. (1988). Deformable mod-
els. Vis. Comput., 4:306–331.
Thormahlen, T., Broszio, H., and Wassermann, I. (2003).
Robust line-based calibration of lens distortion from a
single view. Mirage 2003, pages 105–112.
VISAPP 2009 - International Conference on Computer Vision Theory and Applications
530