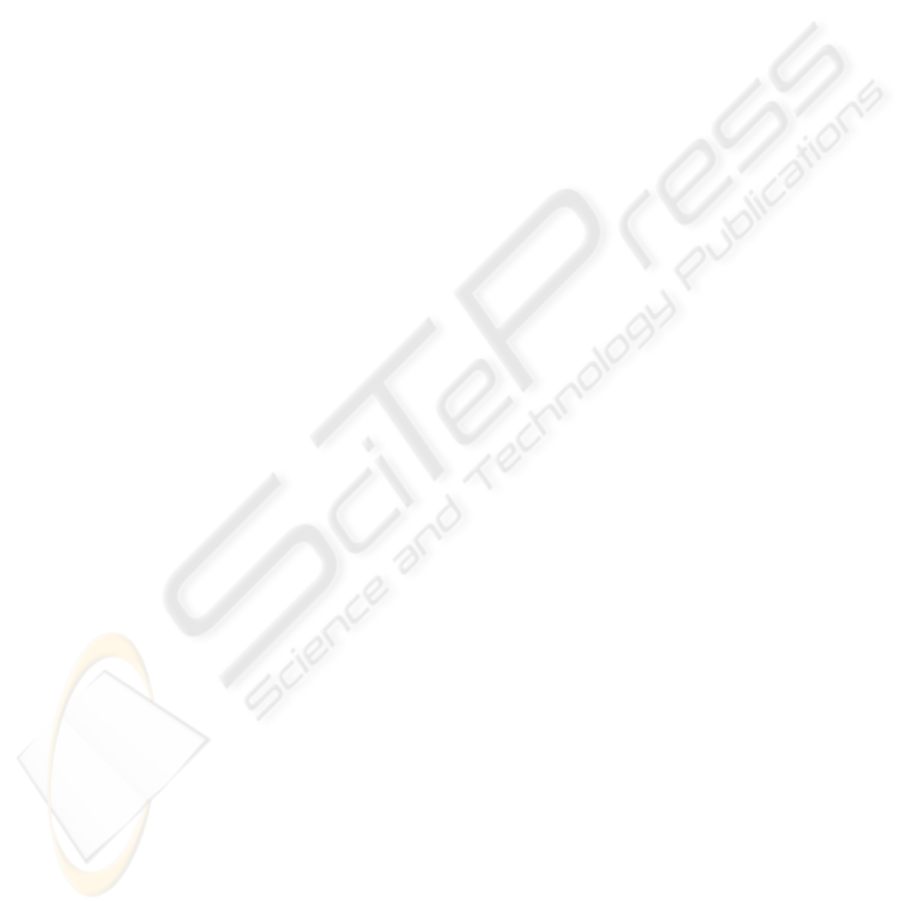
5 CONCLUSIONS
A new algorithm for segmenting MR images is pro-
posed. The algorithm is based on the level set ap-
proach and is conceived to overcome some of the dif-
ficulties of the original level set method: the solution
is repeatable as regard as changes in initial conditions
and the precision of the result is very high. This algo-
rithm can be used for many applications in the field of
Computer Aided Diagnosis.
Currently we are working on the extension of the ex-
periments to assess the results. Then we will analyse
the new results to find other improvements to the de-
scribed method.
REFERENCES
Adams, R. and Bischof, L. (1994). Seeded region growing.
IEEE Transaction on Pattern Analysis and Machine
Learning, 16(6):641–647.
Ardizzone, E., Pirrone, R., and Gambino, O. (2007). Fuzzy
C-Means Segmentation on Brain MR Slices Corrupted
by RF-Inhomogeneity, chapter 48, pages 378–384.
Springer-Verlag.
Banga, C., Ghorbel, F., and Pieczynski, W. (1992). Un-
supervised bayesian classifier applied to the segmen-
tation of retina image. IEEE Proceedings of the An-
nual International Conference of the Engineering in
Medicine and Biology Society, 5:1847–1848.
Caselles, V., Kimmel, R., and Sapiro, G. (1997). Geodesic
active contours. International Journal of Computer
Vision, 22-1:61–79.
Chan, T. and Vese, L. (2001). Active contours without edge.
IEEE Transaction on Image Processing, 10(2):266–
277.
Clark, M. C., Hall, L. O., Goldgof, D., Clarke, L. P.,
Velthuizen, R., and Silbiger, M. S. (1994). Mri seg-
mentation using fuzzy clustering techniques. IEEE
Engineering in Medicine and Biology, 13(5):730–742.
Cremers, D., Rousson, M., and Deriche, R. (2005). Review
of statistical approaches to level set segmentation: In-
tegrating color, texture, motion and shape. Interna-
tional Journal on Computer Vision, 19(10):1–35.
Dervieux, A. and Thomasset, F. (1979). A finite element
method for the simulation of raleigh-taylor instability.
Springer Lecture Notes in Mathematics, 771:145–158.
Foggia, P., Guerriero, M., Percannella, G., Sansone, C.,
Tufano, F., and Vento., M. (2006). A graph-based
method for detecting and classifying clusters in mam-
mographic images. In et al, D.-Y. Y., editor, Lecture
Notes in Computer Science, volume 4109, pages 484–
493. Springer-Verlag, Berlin.
Krause, B., Wells, W., Kikinis, R., Held, K., and Kops, E.
(1997). Markov random field segmentation of brain
mr images. IEEE Transaction on Medical Imaging,
16(6):878–886.
Leitner, F. and Cinquin, P. (1991). Complex topology 3d ob-
jects segmentation. In SPIE Conference on Advances
in Intelligent Robotics Systems.
McInerney, T. and Terzopoulos, D. (1995). Topologically
adaptable snakes. In 5th International Conference on
Computer Vision, pages 840–845. IEEE Comp. Soc.
Press.
Osher, S. and Santosa, F. (2001). Level set medhods for
optimization problems involving geomertry and con-
straints i. frequencies of a two-density inhomogeneous
drum. Journal of Computational Physics, 171:272–
288.
Osher, S. J. and Sethian, J. A. (1988). Fronts propagation
with curvature dependent speed: Algorithms based
on hamiltonjacobi formulations. Journal of Compu-
tational Physics, 79:12–49.
Pappas, T. (1992). An adaptive clustering algorithm for im-
age segmentation. IEEE Transaction on Signal Pro-
cessing, 40.
Russon, M. and Paragios, N. (2002). Shape priors for level
set representation. Lecture Notes in Computer Sci-
ence, 2351:78–92.
Vemuri, B. C., Rahman, S. M., and Li, J. (1995). Multires-
olution adaptive k-means algorithm for segmentation
of brain mri. In ICSC ’95: Proceedings of the Third
International Computer Science Conference on Image
Analysis Applications and Computer Graphics, pages
347–354. Springer-Verlag.
Vrooman, H. A., Cocosco, C. A., van der Lijn, F., Stokking,
R., Ikram, M. A., Vernooij, M. W., Breteler, M. M.,
and Niessen, W. J. (2007). Multi-spectral brain tissue
segmentation using automatically trained k-nearest-
neighbor classification. NeuroImage, 37(1):71–81.
Yan, P. and Kassim, A. A. (2006). Segmentation of volu-
metric mra images by using capillary active contour.
Medical Image Analysis, 10:317–329.
Zhang, H., Zhang, J., Cao, J., Wang, W., Gong, J., and
Wang, X. (2007). Robust fast marching method based
on anisotropic diffusion. In Third International Con-
ference on Natural Computation, volume 3, pages
159–162.
EVALUATION AND IMPROVEMENTS OF THE LEVEL SET METHOD FOR RM IMAGES SEGMENTATION
215