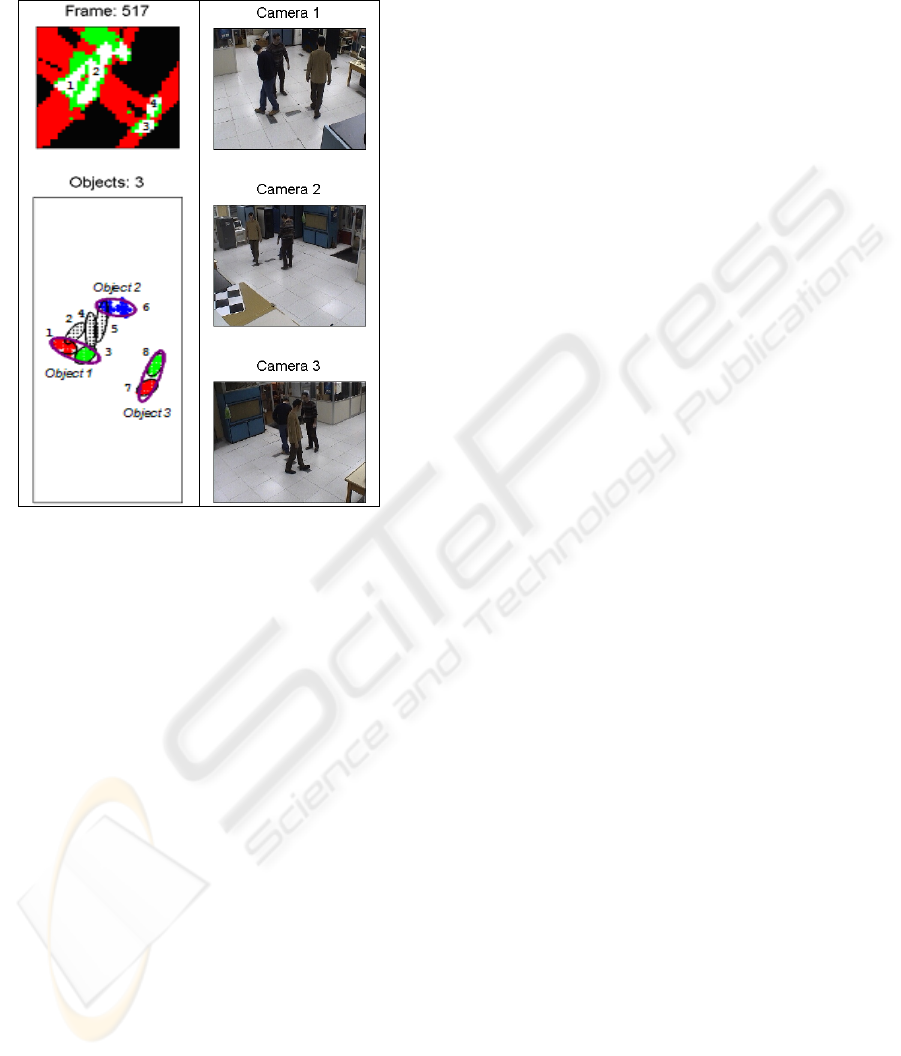
v
t =0.5). The object determination process ignores
them and correctly determines the three objects
consisting by blobs (1,3), (6) and (7,8), respectively.
Figure 5: Blob normalization and pseudo-blob removal for
large blobs. After dividing the large blobs into smaller
ones, only those that are sufficiently visible from the
cameras participate in the object determination process.
5 CONCLUSIONS
In this paper we addressed the problem of detecting
humans in crowded scenes where several occlusions
take place. We have used only geometrical
information given the foreground silhouettes. We
have identified the main sources of errors when
detecting humans based on the homography
constraint. Namely these are the merging – splitting
of accumulator corresponding to maxima and the
appearance of maxima not corresponding to humans.
We have set the criteria for the split operation and
we have shown how to identify and reject the false
positives. The presented experimental results have
verified the proposed approach. Generally if the feet
are partially visible from one camera and detected as
foreground we are able to detect the presence of a
human and not to reject the associated maximum in
the map as false positive. Our next steps include
integrating our detection scheme with a tracker for
consistent monitoring of humans in crowd.
REFERENCES
Makris A., Kosmopoulos D., Perantonis S, Theodoridis S.,
2007. A Hierarchical Feature Fusion Framework for
Adaptive Visual Tracking. In
IEEE Int. Conf. on
Image Processing
, ICIP07, vol 6, pp 289-292.
Eshel, R., Moses, Y., 2008. Homography based multiple
camera detection and tracking of people in a dense
crowd. In
IEEE Conf. on Computer Vision and Pattern
Recognition
, pp. 1-8.
Hu W. , Hu M. , Zhou X. , Tan T., Lou J., Maybank S.,
2006. Principal axis based correspondence between
multiple cameras for people tracking. In
IEEE
Transactions on Pattern Analysis and Machine
Intelligence
, vol. 28, no 4, pp. 663–671.
Hall D., Nascimento J., Ribeiro P., Andrade E., Moreno
P., Pesnel S., List T., Emonet R., Fisher R. B., Victor
J. S., Crowley J. L., 2005. Comparison of target
detection algorithms using adaptive background
models. In
Int. Conf. on Computer Vision, pp. 113–
120.
Khan, S.M., Shah, M., 2006. A multiview approach to
tracking people in crowded scenes using a planar
homography constraint. In
Europ. Conf. on Computer
Vision
, vol. 4, pp. 133–146.
Khan S.M., Yan P., Shah M., 2007. A homographic
framework for the fusion of multi-view silhouettes. In
Int. Conf. on Computer Vision pp. 1-8.
Krumm J., Harris S., Meyers B., Brumitt B., Hale M.,
Shafer S., 2000. Multi-camera multi-person tracking
for easy living. In
3rd IEEE Int. Workshop on Visual
Surveillance
, pp. 3-10.
Mittal A., Davis L. S., 2003. M2tracker: A multi-view
approach to segmenting and tracking people in a
cluttered scene. In
Int. J. Computer Vision, vol. 51, no
3, pp. 189–203.
Kelly P. H., Katkere A., Kuramura D. Y., Moezzi S.,
Chatterjee S., 1995. An architecture for multiple
perspective interactive video. In
3rd ACM Int. Conf.
on Multimedia
, pp. 201–212.
Cai Q., Aggarwal J., 1999. Tracking human motion in
structured environments using a distributed-camera
system. In
IEEE Trans. on Pattern Analysis and
Machine Intelligence
, vol. 21, no 11, pp. 1241–1247.
ROBUST OCCLUSION HANDLING WITH MULTIPLE CAMERAS USING A HOMOGRAPHY CONSTRAINT
565