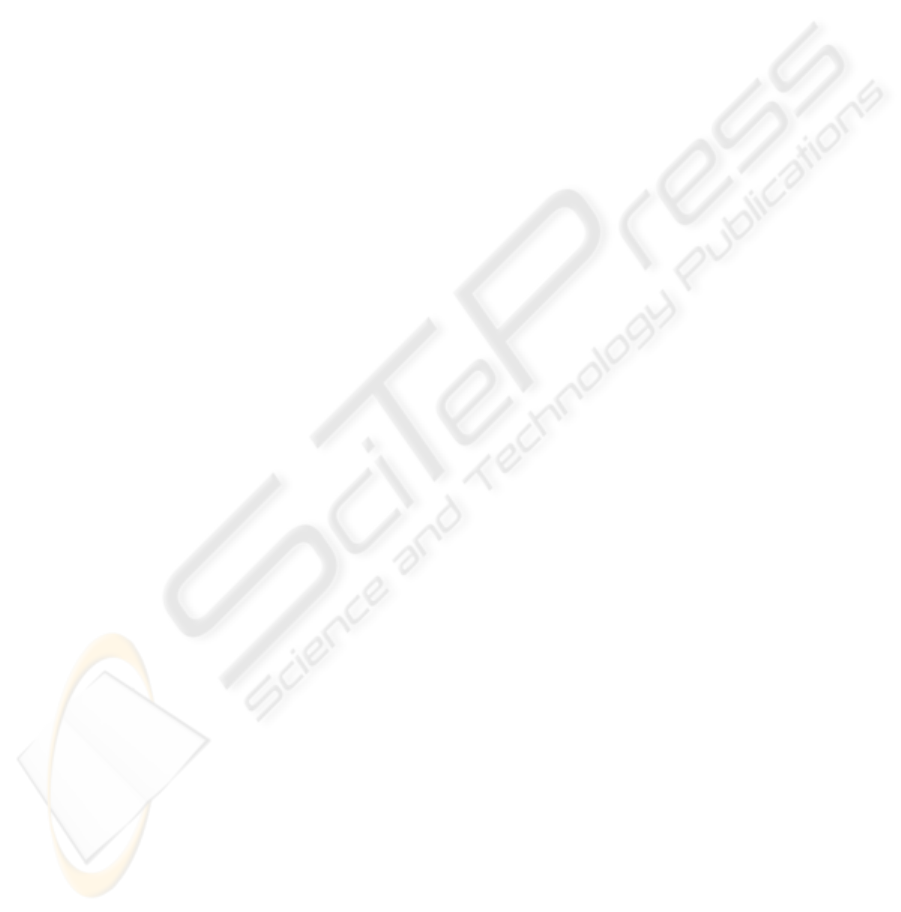
of atypical masses (slightly lobulated or spiculated benign masses and round or cir-
cumscribed malignant tumors) which cause more misclassified cases than the data set
B1. Also, the combination of the five descriptors: SPICUL, SEP, CDV, P and A outper-
forms the use of fractal dimension, that provides as better results with the use of 1D
ruler method A
z
= 0.94 for data set B1 versus A
z
= 0.986 in our case and A
z
= 0.81
for data set B2 versus A
z
= 0.9792.
4 Conclusions
In this paper, we propose an empirical evaluation of three morphological descriptors
which are useful in the analysis of breast masses contours. For evaluation, we use two
independent data sets from Alberta and MIAS. These data sets are widely different and
independent which allows as to generalize from final results. When computing descrip-
tors, we notice their ability to capture diagnostically important details of shape related to
spicules and lobulations. The proposed descriptors, joined to the geometrical features
perimeter and area, have provided high classification accuracies when discriminating
between benign breast masses and malignant tumors. This result outperforms classifi-
cation accuracy of the two descriptors P and A for the two data sets, which prove the
performance and the precision of these descriptors. In future works, we intend to eval-
uate the performance of each descriptor apart and to compare them to other pertinent
descriptors cited in literature which have proven a high performance in mass classifi-
cation. Also, we intend to modify classification tools in order to reduce False Positive
Fraction and to further maximize True Positive fraction.
References
1. C-M Chen, Y-H Chou, K-C Han, G-S Hung, C-M Tiu, H-J Chiou, S-Y Chiou, ”Breast Le-
sions on Sonograms: Computer-aided Diagnosis with Nearly Setting-Independent Features
and Artificial Neural Networks”, Radiology, Fvrier 2003, p504-514.
2. H-K Chiang, C-M Tiu, G-S Hung, S-C Wu, T-Y Chang, Y-H Chou, ”Stepwise Logistic Re-
gression Analysis of Tumor Contour Features for Breast Ultrasound Diagnosis”, IEEE Ul-
trasonic Symposium, 2001, p1303-1306
3. D-R Chena, R-F Changb, C-J Chenb, M-F Hob, S-J Kuoa, S-T Chena, S-J Hungc, W-K
Moond, ”Classification of breast ultrasound images using fractal feature”, Elseiver, Journal
of Clinical Imaging Vol, 29, 2005, p 235-245
4. S Kim and S Yoon, ”Bi-rads features-based computer-aided diagnosis of abnormalities in
mammographic images”. In 6th International Special Topic Conference on ITAB,(2007).
5. A Oliver, J Freixenet, R Marti, J Pont, E Prez, E-R-E. Denton and R Zwiggelaar, ”A
Novel Breast Tissue Density Classification Methodology”, IEEE Transactions On Informa-
tion Technology In Biomedicine, Vol. 12, No. 1, January 2008, p55-65.
6. American College of Radiology ”BI-RADS (Breast Imaging Reporting and Data System”)
Frensh Edition realized by SFR (Societe Francaise de Radiologie), Third Edition, 2003.
7. Alberta Program for the Early Detection of Breast Cancer. Alberta Cancer Board, 2001.
8. H Rangayyan, R., and J Desautels, ”Content-based retrieval and analysis of mammographic
masses”. In Journal of Electronic Imaging.(2005).
9. The mammographic image analysis society digital mammogram database. In
http://www.wiau.man.ac.uk/services/MIAS/MIASweb.html.
99