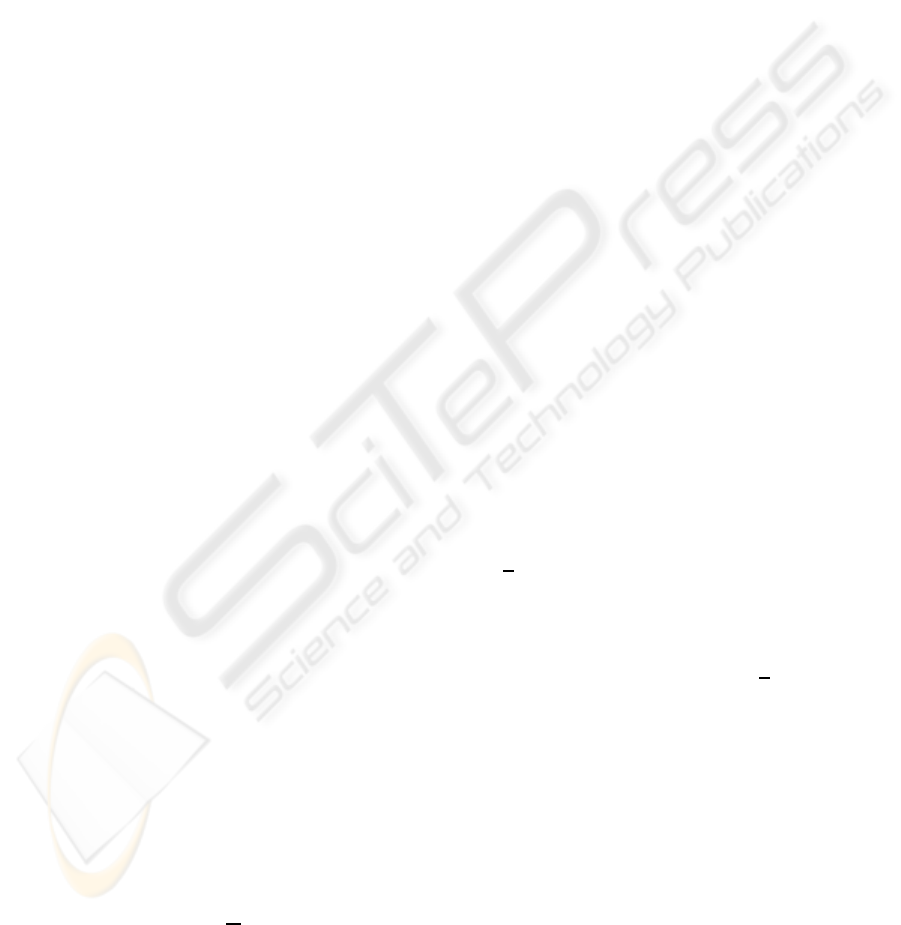
In this paper, a novel method for the stereo vision
is presented using pairs of heterogeneous images. To
achieve such a result, the focal ratio between the focal
lengths of the two images is computed for resizing the
narrower image. The resized image has homogeneous
focal information with respect to the wider image and
to make it homogenous in terms of image resolution,
zero padding is performed around the resized image.
Once the images are made homogeneous by these two
steps, then rectification process is run. Scale invari-
ant features (Lowe, 2004) and (Micheloni and Foresti,
2003) are detected from both images to obtain pairs of
matching points . Rectifying transformations are ob-
tained by solving a nonlinear constrained minimiza-
tion problem (Fusiello and Irsara, 2006), (Isgro and
Trucco, 1999). The gray-level values are normalized
in stereo images based on the intensities information
of matching pairs. Disparity values have been com-
puted to build range images from the given pairs of
stereo images (Scharstein and Szeliski, 2002). In the
disparity estimation, SSD criterion (Tao et al., 2001)
is used to find the best candidate for matching.
The rest of the paper is organized as follows: Sec-
tion 2 is devoted to the detailed description of trans-
forming process from heterogeneous to homogeneous
pair of images. In section 3, SIFT matching is ex-
plained. Section 4 contains the stereo matching pro-
cess. In section 5, experimental results using our
methodology are given and finally in section 6, the
concluding remarks are given.
2 TRANSFORMING PAIR INTO
HOMOGENEOUS IMAGES
The images captured by a pair of heterogenous cam-
eras have different imaging parameters. These make
the acquired images heterogeneous due to camera po-
sitions, orientations, zoom and illumination. If we di-
rectly perform the further operations like SIFT, recti-
fication and stereo matching on these images, the re-
sults would be affected by major performance degra-
dation. To overcome this difficulty, the pair of images
is made homogeneous before performing further op-
erations. The process to make the heterogeneous pair
of images as homogeneous is shown in Figure 1.
Let f
s
and f
d
be the focal lengths of the static and
the PTZ cameras respectively when images are cap-
tured. The focal ratio is R =
f
s
f
d
is computed and the
image captured by the PTZ camera is shrunk by a fac-
tor of R. The shrunk image is then made homoge-
neous with respect to the static image by performing
zero padding. Pairs of corresponding points (m
i
, m
′
i
)
are then extracted by exploiting a SIFT matching al-
gorithm. Such points are therefore used to compute
the rectification transformations H and H
′
by mini-
mizing
∑
i
(m
′
i
T
H
′T
F
∞
Hm
i
)
where F
∞
is the fundamental matrix for rectified
pair. To perform this minimization we choose the
Levenberg-Marquardt algorithm because of its effec-
tiveness and popularity. However, rectification pro-
cess is performed to simplify a stereo matching pro-
cedure, and if the first row of H and H
′
is not chosen
carefully in minimization, it may lead to a larger er-
ror and so failure in matching. Therefore, it is nec-
essary to introduce some constraints in minimization
process. Here, we have used the constraint that the
distance between corresponding epipolar lines along
vertical axis should be zero or very close to zero.
3 SIFT MATCHING
The process to obtain the matching points from the
pair of stereo images is divided into two steps. First,
we detect the scale invariant features in each image
separately. In the next step, matching process of these
features is performed between stereo pair of images.
The process of identifying locations in image
scale space that are invariant with respect to image
translation, scaling and rotation is based on the local-
ization of a key. This task can be performed in fol-
lowing steps:
1. Perform the convolution operation on input image
I with the Gaussian function with variance σ =
√
2. Let this operation gives an image I
1
.
2. Repeat the step 1 on image I
1
to get a new image
I
2
.
3. Subtract image I
2
from image I
1
to obtain the dif-
ference of Gaussian function as
√
2.
4. Resample the image I
2
using bilinear interpolation
with a pixel spacing of 1.5 in each direction. A
1.5 spacing means that each new sample will be a
constant linear combination of 4-adjacent pixels.
From this we generate a new pyramid level.
5. Determine the maxima and minima of this scale-
space function by comparing each pixel in the
pyramid to its neighbors.
6. Select key locations at maxima and minima of a
difference of Gaussian function applied in scale
space.
The scale invariant features can be detected from the
locations of these keys. These features are detected
VISAPP 2009 - International Conference on Computer Vision Theory and Applications
552