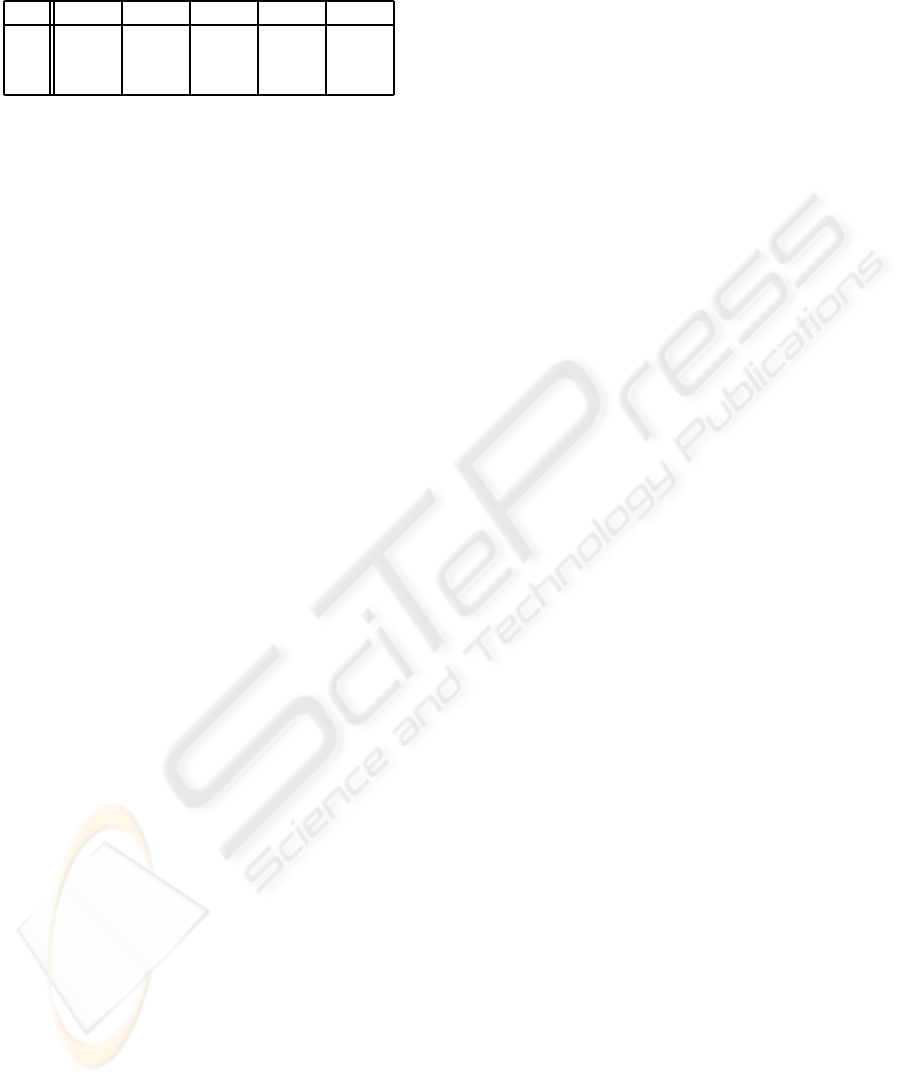
Table 4: SVD + k-NN accuracy rates for Words, Lemmas
and Nouns.
100 200 300 400 500
W. 82.98 84.30 84.89 84.76 84.63
L. 85.95 86.61 86.81 87.33 87.07
N. 84.37 85.36 84.83 85.03 84.76
6 CONCLUSIONS AND FUTURE
WORK
Along this paper, we have analyzed the categorization
of documents written in Basque with the purpose of
facilitating the construction of the domain module in a
CSLS. This work constitutes an important step in the
process of semi-automatically acquiring the domain
module of CSLSs. The two experiments performed in
this study show that advancesin the field of Electronic
Document Technologies can find interesting applica-
tions in the field of Artificial Intelligence in Educa-
tion. Results demonstrate that the k-NN classifica-
tion algorithm combined with the SVD dimensional-
ity reduction technique gives very good results even
for a lesser-used and highly inflected language such
as Basque. We would like to emphasize that when
lemmatization is used, results increase up to 87.33%.
In our experiments we have confirmed that cate-
gorization results are also good when documents are
written in Basque. This will permit us to face the
Basque document categorization problem for an ed-
ucational environment in a more established way. It
will be a great advance in the process of constructing
the domain module for CSLSs in a semi-automatic
way. However, the lack of a Basque educational col-
lection of documents makes this first step of acquisi-
tion of learning material be harder. Our future work
will be conducted to construct such a corpus (Ghani
et al., 2001) and repeat the experiments in order to
confirm the good results.
Regarding the domain acquisition task, we are
currently working in the automatic extraction of the
main topics and the pedagogical relations among
them represented, explicitly or implicitly, in the ta-
ble of contents of a document. A set of heuristics
that infer such relations and the part-of-speech infor-
mation have been already defined (Larra˜naga et al.,
2004) (Larra˜naga et al., 2008).
ACKNOWLEDGEMENTS
This work is supported by the MEC (TIN2006-14968-
C02-01) and by the University of the Basque Country
(UE06/19).
REFERENCES
Alegria, I., Artola, X., Sarasola, K., and Urkia, M. (1996).
Automatic morphological analysis of basque. Literary
& Linguistic Computing, 11.
Aleven, V., Hoppe, U., Kay, J., Mizoguchi, R., Pain, H.,
Verdejo, F., and Yacef, K., editors (2003). Technolo-
gies for Electronic Documents for Supporting Learn-
ing.
Berry, M. and Browne, M. (1999). Understanding Search
Engines: Mathematical Modeling and Text Retrieval.
SIAM Society for Industrial and Applied Mathemat-
ics, ISBN: 0-89871-437-0, Philadelphia.
Carlson, A., Cumby, C., Rosen, J., and Roth, D. (1999).
Snow. UIUC Tech report UIUC-DCS-R-99-210. Uni-
versity of Illinois.
Dagan, I., Karov, Y., and Roth, D. (1997). Mistake-driven
learning in text categorization. In Proceedings of
the 2nd Conference on Empirical Methods in Natural
Language Processing, pages 55–63.
Dasarathy, B. (1991). Nearest neighbor (nn) norms: Nn pat-
tern recognition classification techniques. IEEE Com-
puter Society Press.
Deerwester, S., Dumais, S., Furnas, G., Landauer, T., and
Harshman, R. (1990). Indexing by latent semantic
analysis. Journal of the American Society for Infor-
mation Science, 41:391–407.
Dolin, R., Pierre, J., Butler, M., and Avedon, R. (1999).
Practical evaluation of ir within automated classifica-
tion systems. Proceedings of the International Con-
ference on Information and Knowledge Management
CIKM, pages 322–329.
Dumais, S. (1995). Using lsi for information filtering: Trec-
3 experiments. In Harman, D., editor, Third Text RE-
trieval Conference (TREC3), pages 219–230.
Dumais, S. (2004). Latent semantic analysis. ARIST
(Annual Review of Information Science Technology),
38:189–230.
Ezeiza, N., Aduriz, I., Alegria, I., Arriola, J., and Urizar, R.
(1998). Combining stochastic and rule-based meth-
ods for disambiguation in agglutinative languages.
COLING-ACL’98.
Ghani, R., Jones, R., and Mladenic, D. (2001). Using the
web to create minority language corpora. In Inter-
national Conference on Infroamtion and Knowledge
Management (CIKM 2001).
Graesser, A., Person, N., Harter, D., and Group, T. T. R.
(2001). Teaching tactics and dialog in autotutor. In-
ternational Journal of Artificial Intelligence in Educa-
tion, 12(3):257–279.
homepage, L. L. O. M. W. G. (2001). IEEE P1484.12.
http://ltsc.ieee.org/wg12/.
Joachims, T. (1999). Transductive inference for textclassifi-
cation using support vector machines. Proceedings of
EXPLORING BASQUE DOCUMENT CATEGORIZATION FOR EDUCATIONAL PURPOSES USING LSI
9