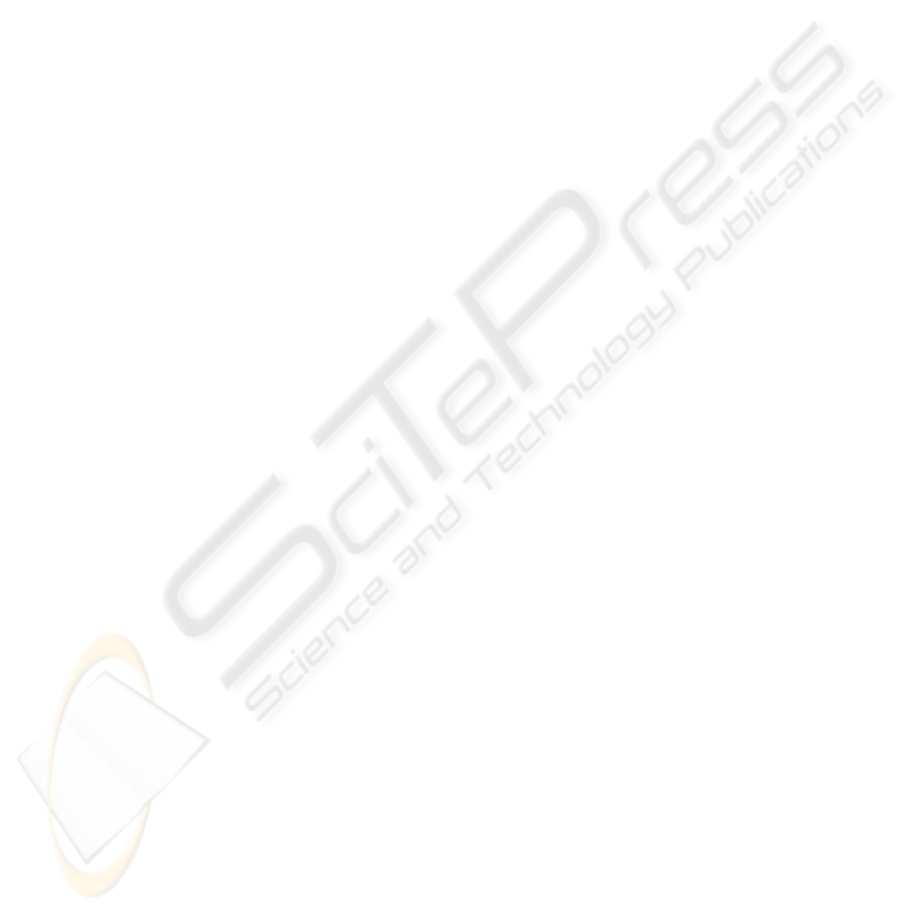
in a given period. From the results in table 4 we can
see that operational costs increased 8% when
comparing with the method used by (Castro and
Oliveira, 2007). If we read this number as is we have
to say that our hypothesis is false. An 8% increased
on operational costs can represent a lot of money.
However, we should read this number together with
the flight delay figure. As we can see, although
method B increased the operational costs in 8% it
was able to choose solutions that decrease, in
average, 36% of the flight delays. This means that,
when there are multiple solutions to the same
problem, our method is able to choose the one with
less operational cost, less quality costs (hence, better
passenger satisfaction) and, because of the relation
between quality costs and flight delays, the solution
that produces less flight delays. From this
conclusion, one can argue that if we just include the
operational costs and the expected flight delay,
minimizing both values, the same results can be
achieved having all passengers happy. In general,
this assumption might be true. However, when we
have to choose between two solutions with impact
on other flights, which one should we choose? In our
opinion, the answer depends on the profile of the
passengers of each flight and on the importance they
give to the delays, and not only in minimizing the
flight delays. Our method takes into consideration
this important information when taking decisions.
This paper has presented an improved version of
the distributed multi-agent system in (Castro and
Oliveira, 2007) as a possible solution to solve airline
operations recovery problems, including sub-
organizations with specialized agents, dedicated to
solve crew, aircraft and passenger recovery
problems, which take into consideration the
passenger satisfaction in the decision process. We
have introduced a process of calculating the quality
costs that, in our opinion, represents the importance
that passengers give to flight delays. We show how,
through a passenger survey, we build four types of
passenger profiles and, for each one of these
profiles, how we calculate a formula to represent
that information. We have introduced an updated
multi-criteria algorithm for selecting the solution
with less cost from those proposed as part of the
negotiation process, taking into consideration the
quality costs. A case study, taken from a real
scenario in an airline company where we tested our
method was presented and we discuss the results
obtained. We have shown that our method is able to
choose solutions that contribute to a better passenger
satisfaction and that produce less flight delays when
compared with a method that only minimizes
operational costs.
ACKNOWLEDGEMENTS
This work is supported by FCT research grant
SFRH/BD/44109/2008. The authors are grateful to
TAP Portugal for allowing the use of real data from
the company.
REFERENCES
Abdelgahny, A., Ekollu, G., Narisimhan, R., and
Abdelgahny, K., 2004. A Proactive Crew Recovery
Decision Support Tool for Commercial Airlines
during Irregular Operations, Annals of Operations
Research, 127, 309-331.
Barnhart, C., Belobaba, P., and Odoni, A., 2003.
Applications of Operations Research in the Air Transport
Industry, Transportation Science, 37, 368-391.
Bratu, S., and Barnhart, C., 2006. Flight Operations
Recovery: New Approaches Considering Passenger
Recovery, Journal of Scheduling, 9,3, 279-298.
Castro, A. and Oliveira, E. (2008) ‘The rationale behind
the development of an airline operations control centre
using Gaia-based methodology’, Int. J. Agent-Oriented
Software Engineering, Vol. 2, No. 3, pp.350–377.
Castro, A.J.M., and Oliveira, E. 2007. Using Specialized
Agents in a Distributed MAS to Solve Airline
Operations Problems: a Case Study. Proceedings of
IAT 2007 (Intelligent Agent Technology Conference),
pp. 473-476, Silicon Valley, California, USA 2-5
November 2007, IEEE Computer Society, ISBN: 0-
7695-3027-3.
Clausen, J., Larsen, A., and Larsen, J.,2005. Disruption
Management in the Airline Industry – Concepts,
Models and Methods. Technical Report 2005-01,
Informatics and Mathematical Modeling, Technical
University of Denmark, DTU.
Kohl, N., Larsen, A., Larsen, J., Ross, A., and Tiourline,
S., 2004. Airline Disruption Management –
Perspectives, Experiences and Outlook. Technical
Report CRTR-0407, Carmen Research.
Kohl, N., and Karish, S., 2004. Airline Crew Rostering:
Problem Types, Modeling and Optimization, Annals of
Operations Research, 127, 223-257.
Lettovsky, L.,1997. Airline Operations Recovery: An
Optimization Approach, Ph.D. Thesis, Georgia
Institute of Technology, Atlanta, USA.
Rosenberger, J., Johnson, E., and Nemhauser, G., 2001.
Rerouting aircraft for airline recovery, Technical
Report TLI-LEC 01-04, Georgia Institute of
Technology.
Rosenberger, J., Schaefer, A., Goldsmans, D., Johnson, E.,
Kleywegt, A., and Nemhauser, G., 2002. A Stochastic
Model of Airline Operations, Transportation Science,
36,4, 357-377.
ICEIS 2009 - International Conference on Enterprise Information Systems
24