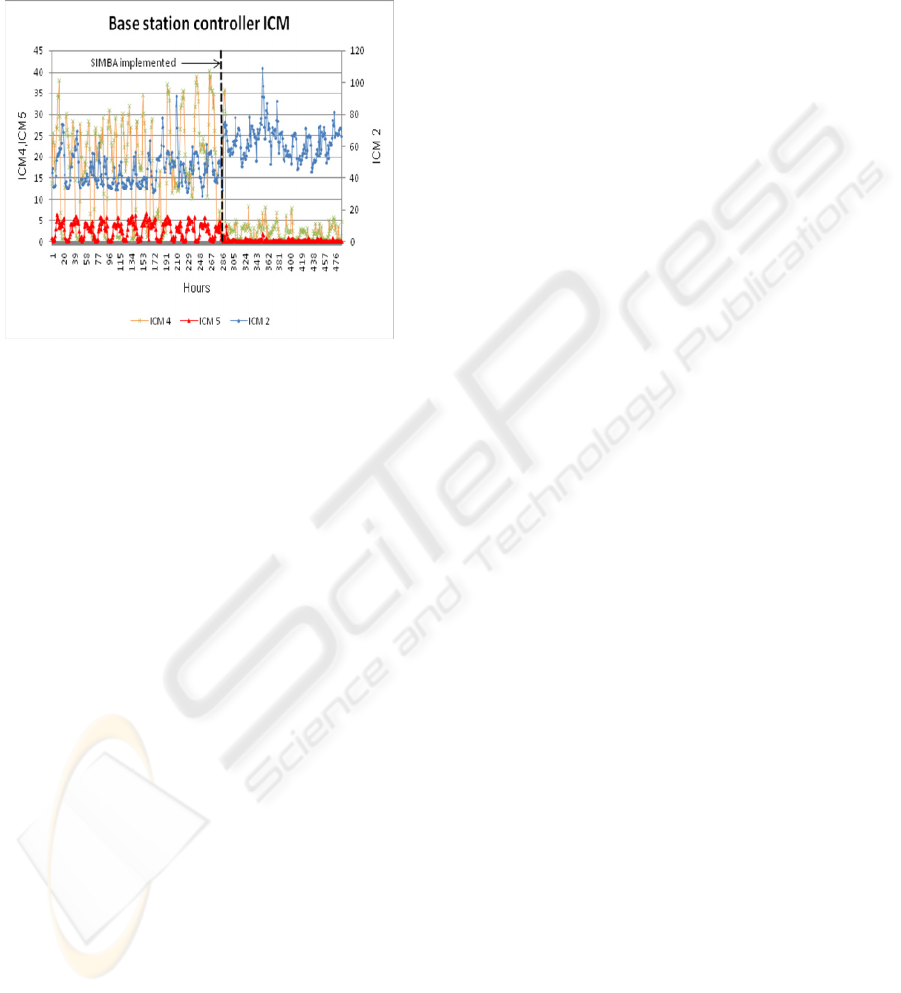
considerable improvements to the quality on the
BSC. The BSC was optimized to the ideal situation
with regard to the ideal channel measurements (see
section 6) as the number of points has decreased in
the ICM4 and ICM5 bands, while the ICM2 band
has increased considerably (see figure 4).
Figure 4: ICM interference bands 2, 4 and 5 before and
after the SIMBA frequency assignment solution was
implemented.
8 CONCLUSIONS
An engineering problem of high practical relevance
has been addressed in this paper. An algorithm based
on swarm intelligence that utilizes a multi-agent
system has been proposed. The SIMBA also
includes a stigmergy single cell optimization
(SSCO) approach which is used during selection of
the best frequencies that minimize interference
patterns. This approach has a strong analogy to
natural stigmergy in natural. One of the most
important characteristics of the SSCO was that
agents recognize interference patterns in the
changing structure of the frequency assignment
solution and are able to augment it with new
components that minimize the interference pattern.
The agents are able to make these decisions as the
changing configuration of the structures acts as a
source of information that aids the agents.
Another important aspect of the SIMBA was the
social model created by the multi-agent system.
Each agent was able to make local changes to its
own local knowledge; however the agent also could
gain addition knowledge from the collective giving
the SIMBA its swarm intelligence characteristics.
The SIMBA was benchmarked against the
COST 259 benchmarks, in particular the Siemens set
of problems and the SIMBA closely match some of
the best results with a search time of 24 hours. The
frequency assignment solutions produced by SIMBA
were also implemented into a commercial cellular
radio network with good results.
ACKNOWLEDGEMENTS
The authors wish to thank the University of
Johannesburg and the Academy for Information
Technology at the University of Johannesburg.
REFERENCES
Beckmann, D., Killat, U., 1999. Frequency Planning with
respect to Interference Minimization in Cellular Radio
Networks. TD(99)032, COST 259, Vienna, Austria,
January.
Bonabeau, E., Dorigo, M., Theraulaz, G., 1999. Swarm
Intelligence: From Natural to Artificial Systems (Santa
Fe Institute Studies in the Sciences of Complexity
Proceedings), Oxford University Press.
Eisenblätter A. and Koster A., 2008. FAP web page,
http://fap.zib.de
Hale, W.K., 1980. Frequency assignment: theory and
applications. Proc. IEEE, vol. 68, pp 1497-1514, Dec.
Hao J., Dorne R., Galinier P., 1998. Tabu Search for
Frequency Assignment in Mobile Radio Networks.
Journal of Heuristics, vol 4 pp 47-62.
Hellebrandt, H., and H. Heller, H., 2000. A new heuristic
method for frequency assignment. TD(00)003, COST
259, Valencia, Spain, January.
Kristensen, L.K., 2000. Aintz: Collective Problem Solving
by Artificial Ants.
http://www.evalife.dk/publications2.php
Mannino, C., Oriolo, G., Ricci, F., 2002. Solving Stability
Problems on a Superclass of Interval Graphs.
Universit'a di Roma La Sapienza, Dipartimento di
Informatica e Sistemistica, vol.26 no. 02.
Michalewicz, Z., Schmidt, M., Michalewicz, M., Chiriac,
C., 2007. Adaptive Business Intelligence. Springer.
Montemanni, R., Moon, J.N.J., Smith D.H., 2003. An
Improved Tabu Search Algorithm for the Fixed-
Spectrum Frequency Assignment Problem. IEEE
Transactions on Vehicular Technology, vol. 52, no. 3,
pp 891-901, May.
O’Reilly, G.B., and Ehlers E.M., 2008. The Swarm Effect
Minimization Algorithm. 10
th
International Conference
on Enterprise Information Systems (ICEIS),
Barcelona, Spain, June.
Valckenaers, P., Kollingbaum, M., van Brussel, H.,
Bochmann, O., and Zamfirescu, C., 2001. The Design
of Multi-Agent Coordination and Control Systems
using Stigmergy. Proc. of the IWES'01 Conference.
http://www.csd.abdn.ac.uk/~mkolling/publications/De
signMultiAgentCoordControlStigmergy.pdf
ICEIS 2009 - International Conference on Enterprise Information Systems
32