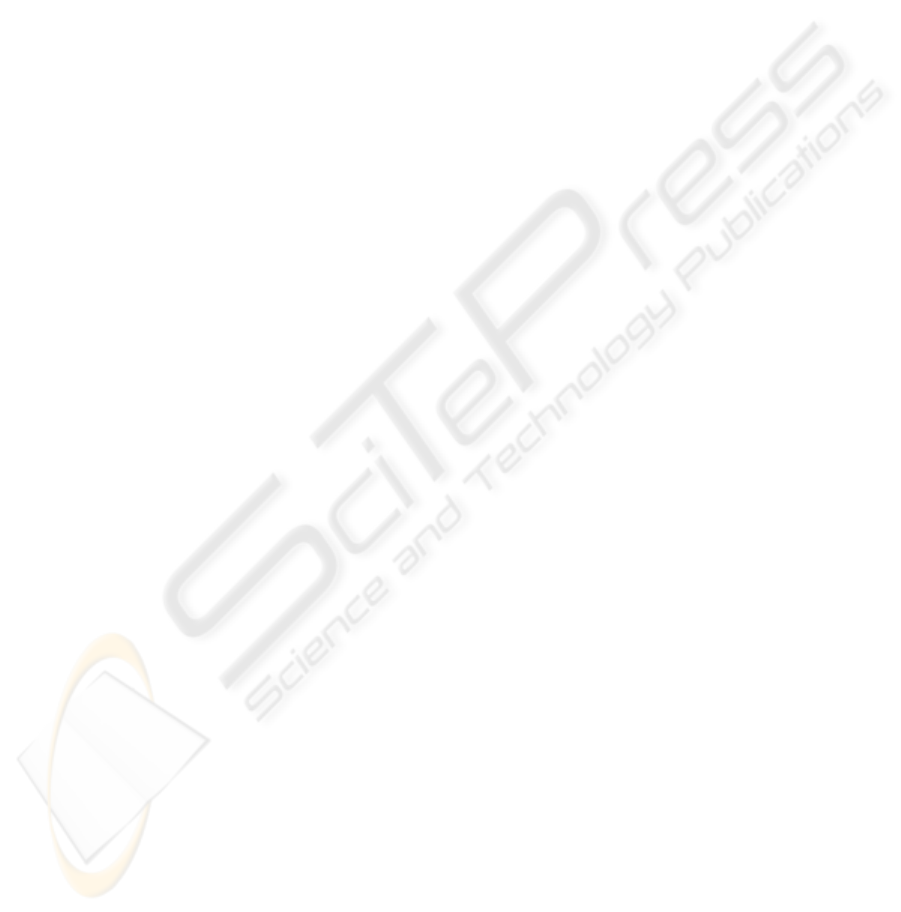
enabling computation across distinct machines,
therefore improving overall performance and
reliability. This feature also enabled simultaneous
multi-terminal access, both to the real-time analysis
tool and the historical statistical software.
Taking into consideration the project’s tools,
both were classified, by the retail company’s end-
users – mainly shop managers, marketing directors
and board administrators – as extremely useful and
allowed swift knowledge extraction, preventing
them the excruciating, and not often useless process
of getting through massive indirect location data.
The immediate visual information provided by the
system proved to be effective in direct applications
such as queue management and hot and cold zones
identification, and most significant, in what concerns
to visit’s pattern extraction across different time
dimensions, thus enhancing marketing and logistic
decisions’ impact. One must refer to Oracle’s APEX
technology adoption. It has demonstrated to be able
to allow multiple simultaneous accesses and,
consequently, dramatically enhancing analysis
empowerment, while, at the same time, eliminated
heavy data computation from end-users terminals,
concentrating it in controlled and expansible
clusters.
Regarding future work areas, there has been
identified a set of potential project enhancements
that would be able to suppress some hurdles and,
somehow, wide potential new applications.
Considering business intelligence extraction, it
would be useful to build or reuse an inference engine
capable of determining the odds of a given customer
turn right or left in the next decision point, taking for
that, into account his past actions and comparing
them to other customers’ action that are classified in
the same cluster. This aspect should be also applied
to historical data so that efficient customer clusters
would be defined and maintained.
There have been identified several application
domains that go beyond the retail segment. Amongst
these, one shall mention the possible system’s
adoption by large warehouse management where
traffic jams are not unusual.
As a summary, it is fair to state that the project’s
initial ambitions were fully met and that the close
cooperation with an important stakeholder in the
global retail market was extremely important for
better measuring the system’s positive impact and
potential firstly unseen applications. The technology
transparency, allied with the future work areas, is
believed to greatly improve potential applications in
several domains, thus significantly widening the
project’s initial horizons.
REFERENCES
C, C., J, Y., and W, J. Determining technology trends and
forecasts of RFID by a historical review and
bibliometric analysis from 1991 to 2005. In
Technovation27, Elvisier Ltd, page 268-279, 2007.
Cai, Q. and Aggarwal, J. Tracking human motion in
structured environments using a distributed camera
system. In IEEE Trans. Patt. Analy. Mach.
Intell.,pages 1241–1247, 1999.
Collins, R., Lipton, A., Fujiyoshi, H. and Kanade, T.
Algorithms for cooperative multisensory surveillance.
In Proceedings of IEEE, pages 1456–1477, 2001.
Elgammal, A., Duraiswami, R., Harwood, D. and Davis,
L. Background and foreground modeling using
nonparametric kernel density estimation for visual
surveillance. In Proceedings of IEEE, pages 1151–
1163,2000.
Huang, T. and Russel, S. Object identification in a
Bayesian context. In Proceedings of International
Joint Conference on Artificial Intelligence, pages
1276–1283, 1997.
Jappinen, P. and J, P. Preference-aware ubiquitous
advertisement screen. In e-commerce 2007
Proceedings, IADIS Press, pages 99–105, 2007.
Javed, O., Rasheed, Z., Shafique, K. and Shah, M.
Tracking across multiple cameras with disjoint views.
In IEEE International Conference on Computer Vision
(ICCV), pages 952–957, 2003.
Kettnaker, V. and Zabih, R. Bayesian multi-camera
surveillance. In IEEE Conference on Computer Vision
and Pattern Recognition, pages 117–123, 1999.
Khan, S. and Shah, M. Consistent labeling of tracked
objects in multiple cameras with overlapping fields of
view. In IEEE Trans. Patt. Analy. Mach.Intell., pages
1355–1360, 2003.
Lee, L., Romano, R. and Stein, G. Monitoring activities
from multiple video strams:establishing a common
coordinate frame. In IEEE Trans. Patt.Recogn.
Mach.Intell., pages 758–768, August 2000.
MacCormick, J. and Blake, A. Probabilistic exclusion and
partitioned sampling for multiple object tracking. In
Int. J. Comput. Vision, pages 57–71, 2000.
Mingkhwan, A. Wi-fi tracker: An organization wi- fi
tracking system. In IEEE CCECE/CCGEI,
Ottawa,page 1353-1356, 2006.
Mittal, A. and Davis, L. M2 tracker: A multiview
approach to segmenting and tracking people in a
cluttered scene. In Int.J. Comput. Vision
, pages 189–
203, 2003.
Nejikovsky, B., Kesler, K. and Stevens, J. Real time
monitoring of vehicle/track interaction. In Rail
Transportation, pages 25–31, 2005.
Yu, Z. GPS train location and error analysis which based
on the track fitting of the railway’s geometric locus. In
ICEMI 2005: Conference Proceedings of the Seventh
International Conference on Electronic Measurement.
ICEIS 2009 - International Conference on Enterprise Information Systems
16