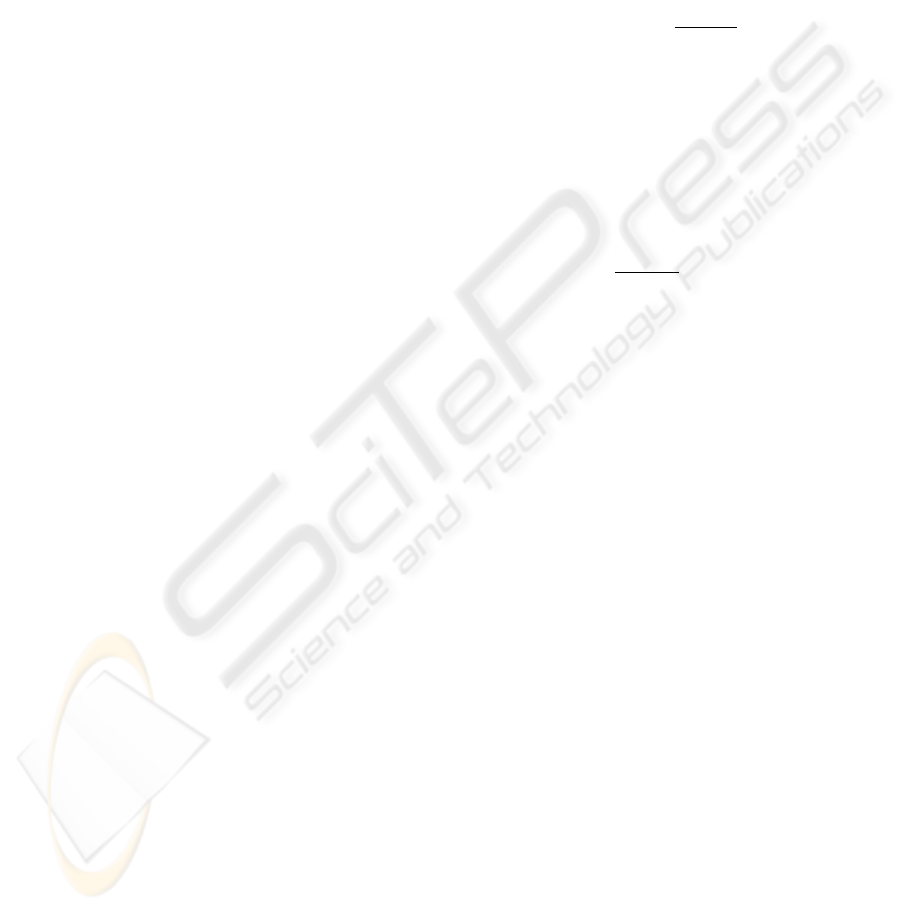
2 ANALYSIS AND RESULTS
2.1 Preliminaries
Amazon is as a pioneer of the Internet. It was founded
as an Internet based bookshop more than 10 years
ago. Today, its product range is similar to the product
range of a classic department store covering kitchen
ware, watches, CD & DVD, computer hard- as well
as software, cloth besides many others products. Be-
sides its own department store business Amazon runs
an Internet-based shop platform for third-party retail-
ers and therefore functions as some kind of Internet
mall.
In the year 2007 Amazon generated net sales of
USD 14,835 millions up from USD 10,711 millions
in the previous year which is an increase of more that
38% (Amazon, 2008). This makes Amazon to the
world’s leading Internet retailer and shopping plat-
form.
On its shopping sites Amazon provides its cus-
tomers a C2C communication platform where reviews
and pictures of products can be exchanged. Amazon
constantly adds new C2C functionality like the re-
cently introduced video messages. The C2C informa-
tion of mostly former buyers shall help potential buy-
ers to make their decisions which product to choose.
So these information influence the level of trust a po-
tential buyer experiences for a product. However,
since the information sources, the authors of the re-
views, are normally not personally known or even
anonymous to the potential buyer she/he faces another
challenge: Can I trust the information sources and
their comments on a certain product.
In this context Amazon provides the possibility to
rate a product review as helpful. So, product reviews
that are mostly considered as helpful can be regarded
as good reviews. The objective of this study is to ana-
lyze correlations between product review features and
the acceptance of a review defined by the helpfulness
parameter. The analysis is conducted within product
categories as well as between different categories.
For our study we choose product categories that
have been used in previous studies on eMarketing and
customer behavior (e.g. (Wang and Head, 2007)) al-
ready. They are listed below:
• Books
• Digital cameras
• Hardware
• Mobile phones
• Software.
For our analysis the following parameters of the
product reviews were selected and group into three
categories:
• Review based Parameters. Text length; ab-
solute and relative numbers of syllables, words,
sentences, paragraphs, punctuation marks, sig-
nal words (superlative and customer-support-
groups), figures, personal pronouns; product
rating given by the reviewer, helpful votes
(Hel p f ulV), total votes (TotalV ), review accep-
tance (Acceptance =
Hel p f ulV
TotalV
), readability in-
dexes (Flesch and SMOG), presence of listed pas-
sages in the review.
• Reviewer based Parameters. Number of re-
views, average rating of all author’s reviews, pres-
ence of the ’real name’ badge, presence of a re-
viewer’s photo.
• Product based Parameters. Average prod-
uct rating (avProdRat), product rating given by
the reviewer (ProdRat), relative product rating
(relProdRat =
ProdRat
avProdRat
), total reviews on prod-
uct, presence of the product name in the review.
As shop systems Amazon was chosen. Besides
its leading position as online shop a main reason for
selecting Amazon was that it provides easy access to
its data.
2.2 Hypotheses
Based on the parameters as given above we defined
hypotheses which can roughly be grouped into the
following categories:
• Letter and Word Analysis
• Semantic Analysis
• Product-based Analysis
• Reviewer-based Analysis
Our hypotheses are defined in the following para-
graphs.
2.2.1 Letter and Word Analysis
In this category we subsume hypotheses that count
letters and words without caring about their meaning.
Text Length. The impact of the text length of a re-
view on its helpfulness is difficult to predict. On the
one hand, a long review may be regarded as more so-
phisticated and comprehensive than a short review;
on the other hand, a short review may be clearer and
more precise.
This motivates to the following hypothesis.
ICEIS 2009 - International Conference on Enterprise Information Systems
42