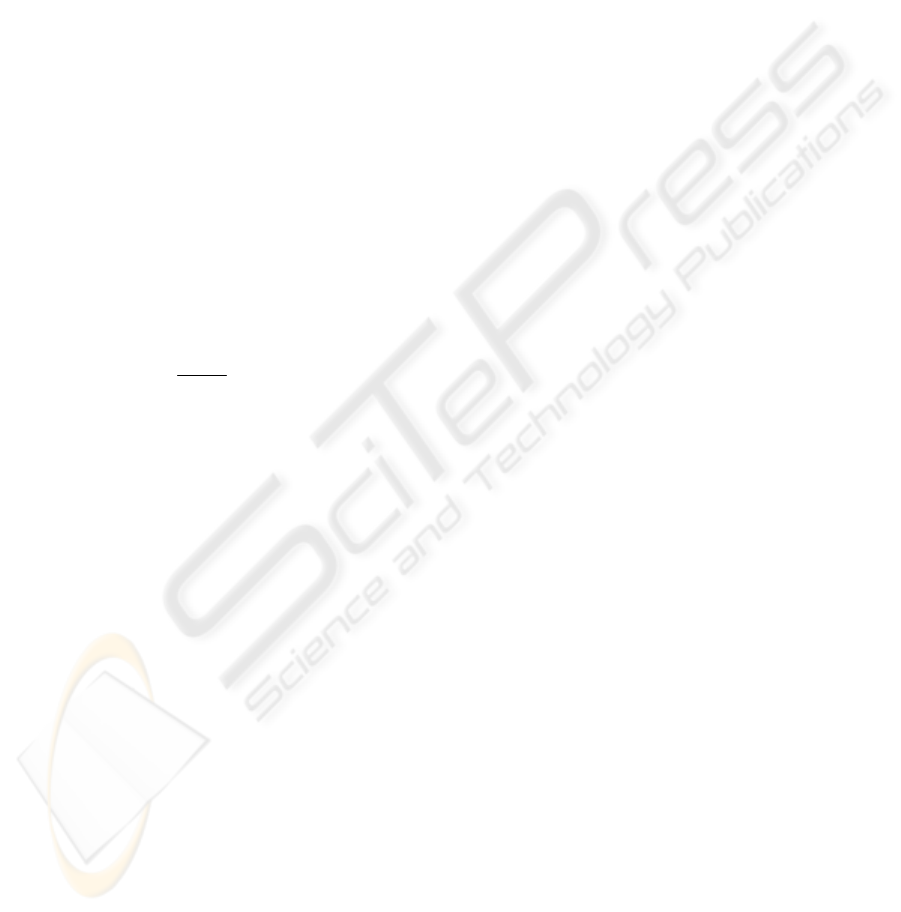
()
PAP
1mm
QI +QI / 2th =
()
AP G
2mm
QI +QI / 2th =
()
GAE
3mm
QI +QI / 2th =
()
AE E
4mm
QI +QI / 2th =
where
PAPGAE E
mm mm m
QI , QI , QI , QI and QI are the mean
QI value of the elements belonging to the class
Poor, Acceptable Poor, Good, Acceptable
Excessive
and Excessive, respectively. Such
partition of the data can be carried out by using these
thresholds as follows: (a) if QI<th
1
, then soldering is
Poor; (b) if th
1
<QI<th
2
, then soldering is
Acceptable Poor; (c) if th
2
<QI<th
3
, then soldering
is
Good; (d) if th
3
<QI<th
4
, then soldering is
Acceptable Excessive; (e) if QI>th
4
, then soldering
is
Excessive. The obtained partition is compared to
experts' one. In particular, a pin is considered as
correctly classified by the system if it belongs to the
same set when considering both the former and the
latter partition. On the contrary, if this condition is
not satisfied, then a misclassification takes place, as
it is shown by the diamond marks in Figure 4. The
performances of the architecture are measured by
defining the Recognition Rate index as:
C
TOT
N
RR = ×100
N
being N
C
and N
TOT
the number of correctly
classified cases and the number of the considered
ones, respectively. Values of RR equal to 96.87%
and 95.83% concerning training and testing data
have been obtained. The results can be considered
encouraging, in fact the obtained values show that
the designed neurofuzzy system yields a
classification similar to that given by the experts,
providing a refined evaluation of the solder joints.
4 CONCLUSIONS
In this paper a neurofuzzy architecture for
computing a Quality Index of a solder joint in a
SMT assembled PCB has been proposed. The
system offers some interesting advantages. In
particular, the suggested solution does not need a
complex illumination and positioning system,
implying that the equipment costs could be reduced
and the assessment of a solder joint could be shifted
on the fuzzy evaluation phase. Moreover, the typical
low computational costs of the fuzzy systems could
satisfy urgent time constrains in the in-line detection
of some industrial productive processes. The
proposed architecture provides a refined evaluation
of the solder joints, automating the human expert
classification.
Basing on the obtained results, it can be argued
that the correct working of the proposed system is
due to its capability to reproduce human experts’
modus operandi properly. Therefore, future
developments will be aimed at identifying the
characteristics, that a human operator evaluates in
order to express the assessments of solder joints. As
a consequence, the focus of future works will be
constituted by the identification of the features
which contain sufficient and useful information to
perform a correct diagnosis.
REFERENCES
Krippner, P., and Beer, D., 2004. “AOI Testing Positions
in Comparision”, Circuit Assembly, Apr. 2004, pp. 26-
32.
Manjeshwar, P., Craik, J., Phadnis, S., and Srihari, K.,
2006. ‘Effectiveness Study of an Automated 3D
Laminography X-Ray Inspection System in a High
Volume–Low-Mix SMT line’, The Int. J. of Adv.
Manuf. Technol., vol. 30 (11-12), pp. 1191 – 1201.
Teramoto, A., Murakoshi, T., Tsuzaka, M., and Fujita, H.,
2007. “Automated Solder Inspection Technique for
BGA-Mounted Substrates by Means of Oblique
Computed Tomography”, IEEE Trans. on Electronics
Packaging Manufacturing, vol. 30 (4) , pp. 285 – 292.
Wu, Y. P., Tu, P. L., and Chan, Y. C., 2001. “The effect of
solder paste volume and reflow ambient atmosphere
on reliability of CBGA assemblies,” J. Electron.
Packag., vol. 123 (3), pp. 284–289.
Zhang, L., Ume, I.C., Gamalski, J., and Galuschki K. P.,
2006. “Detection of Flip Chip Solder Joint Cracks
Using Correlation Coefficient and Auto-Comparison
Analyses of Laser Ultrasound Signals”, IEEE Trans.
on Comp. and Pack. Technol., vol. 29 (1), pp 13-19.
Hsu-Nan, Y., Du-Ming, T., and Jun-Yi Y., 2006. “Full-
Field 3-D Measurement of Solder Pastes Using LCD-
Based Phase Shifting Techniques”, IEEE Trans. on
Electron. Packag. Manuf., vol. 29 (1), pp. 50 – 57.
Ko, K.W., and Cho H.S., 2000. “Solder joint inspection
using a neural network and fuzzy rule-based
classification method”, IEEE Trans. on Electron.
Packag. Manuf., vol. 23 (2), pp. 93–103.
Jagannathan, S., 1997. “Automatic Inspection of Wave
Soldered Joints Using Neural Networks”, J. of Manuf.
Syst., vol. 16 (6), pp. 389-398.
Acciani, G., Brunetti, G., and Fornarelli G., 2006. “A
Multiple Neural Network System to Classify Solder
Joints of Integrated Circuits”, Int. J. of Computational
Intelligence Research., vol. 2 (4), pp. 337-348.
AOI BASED NEUROFUZZY SYSTEM TO EVALUATE SOLDER JOINT QUALITY
53