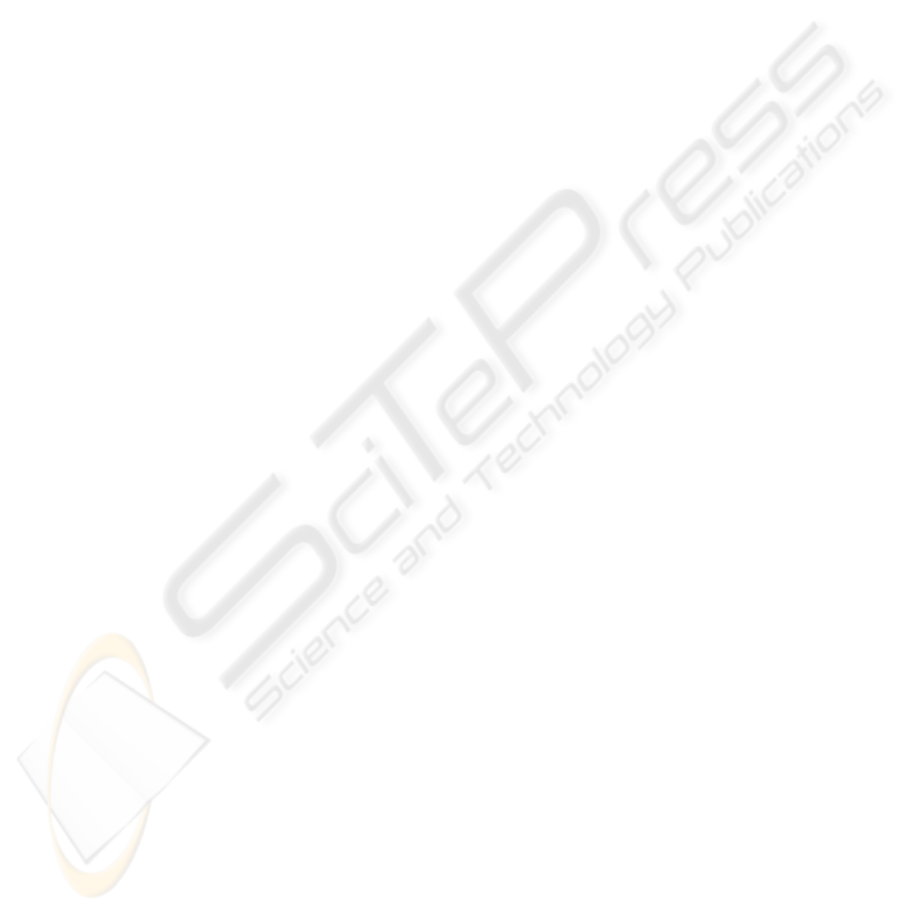
the essential features and capabilities of the essential
features of a knowledge representation technique
and optimization technology. The principles and
techniques can be extended to different industries
with modifications to the fitness function and
structure of chromosome. By incorporating the error
measurement and complexity of process change into
the fitness evaluation, the generalized fuzzy rule sets
can be less complexity and higher accuracy. An
extension of different measures can also be
incorporated in order to improve the quality of
generalized rules. Future work will entail other
fuzzy learning methods to dynamically adjust the
membership functions of various process parameters
for enhancing the accuracy of the system.
ACKNOWLEDGEMENTS
The authors wish to thank the Research Committee
of The Hong Kong Polytechnic University for the
support of this research.
REFERENCES
Bayraktar, D. (1998), A knowledge-based expert system
approach for the auditing process of some elements in
the quality assurance system, Int. J. Production
Economics, vol. 5657, pp. 37-46.
Bernardy, G. and Scherff, B. (1998), SPOC – process
modelling provides on-line quality control and
predictive process control in particle and fibreboard
production, Proceedings of the 24th Annual
Conference of IEEE Industrial Electronics Society,
IECON’98, 31.08.-04.09., Aachen.
Heinloth, S. (2001), Measuring quality’s return on
investment, Quality Yearbook 2001, McGraw-Hill,
New York, NY.
Yang, J.B., Liu, J. , Xu, D.L., Wang, J. and Wang, H.W.
(2007), Optimization Models for Training Belief-
Rule-Based Systems, IEEE Trans. Syst., Man Cybern.
A, Syst., Humans, vol. 37, no. 4, pp. 569-585.
Kobbacy, K., Vadera, S. and Rasmy, M.H. (2007), AI and
OR in management of operations: history and trends,
Journal of the Operational Research Society, vol.58,
pp. 10-28.
Tana, K.H., Limb, C.P., Plattsc, K. and Koay, H.S. (2006),
An intelligent decision support system for
manufacturing technology investments, Int. J.
Production Economics, vol. 104, pp. 179-90.
Yu, F., Tu, F. and Pattipati, K.R. (2006), A novel
congruent organizational design methodology using
group technology and a nested genetic algorithm,
IEEE Trans. Syst., Man Cybern. A, Syst., Humans, vol.
36, no. 1, pp. 5-18.
Chiang, T.C., Huang, A.C. and Fu, L.C. (2007), Modeling,
scheduling, and performance evaluation for wafer
fabrication: a queueing colored Petri-net and GA-
based approach, IEEE Transactions on Automation
Science and Engineering, vol. 3, no. 3, pp. 912-918.
Wang, C.H., Hong, T.P. and Tseng, S.S. (1998),
Integrating fuzzy knowledge by genetic algorithms,
IEEE Transactions on Evolutionary Computation,
vol.2, no. 4, pp. 138-149.
Lau, H.C.W., Ho, G.T.S., Chu, K.F., Ho, W. and Lee,
C.K.M. (2009), Development of an intelligent quality
management system using fuzzy association rules,
Expert Systems with Application, vol.36, no. 2, pp.
1801-1815.
Hwang, S.F. and He, R.S. (2006), Improving real-
parameter genetic algorithm with simulated annealing
for engineering problems, Advances in Engineering
Software, vol. 37, pp. 406-18.
Ho, G.T.S., Lau, H.C.W., Chung S.H., Fung R.Y.K., Chan,
T.M. and Lee, C.K.M (2008), Development of an
intelligent quality management system using fuzzy
association rules, Industrial Management & Data
Systems, vol.108, no. 7, pp. 947-972.
Winnall, S., and Winderbaum, S, (2000), Lithium Niobate
Reactive Ion Etching Electronic Warfare Division,
DSTO Electronics and Surveillance Research
Laboratory, DSTO-TN-0291.
A FUZZY-GUIDED GENETIC ALGORITHM FOR QUALITY ENHANCEMENT IN THE SUPPLY CHAIN
89