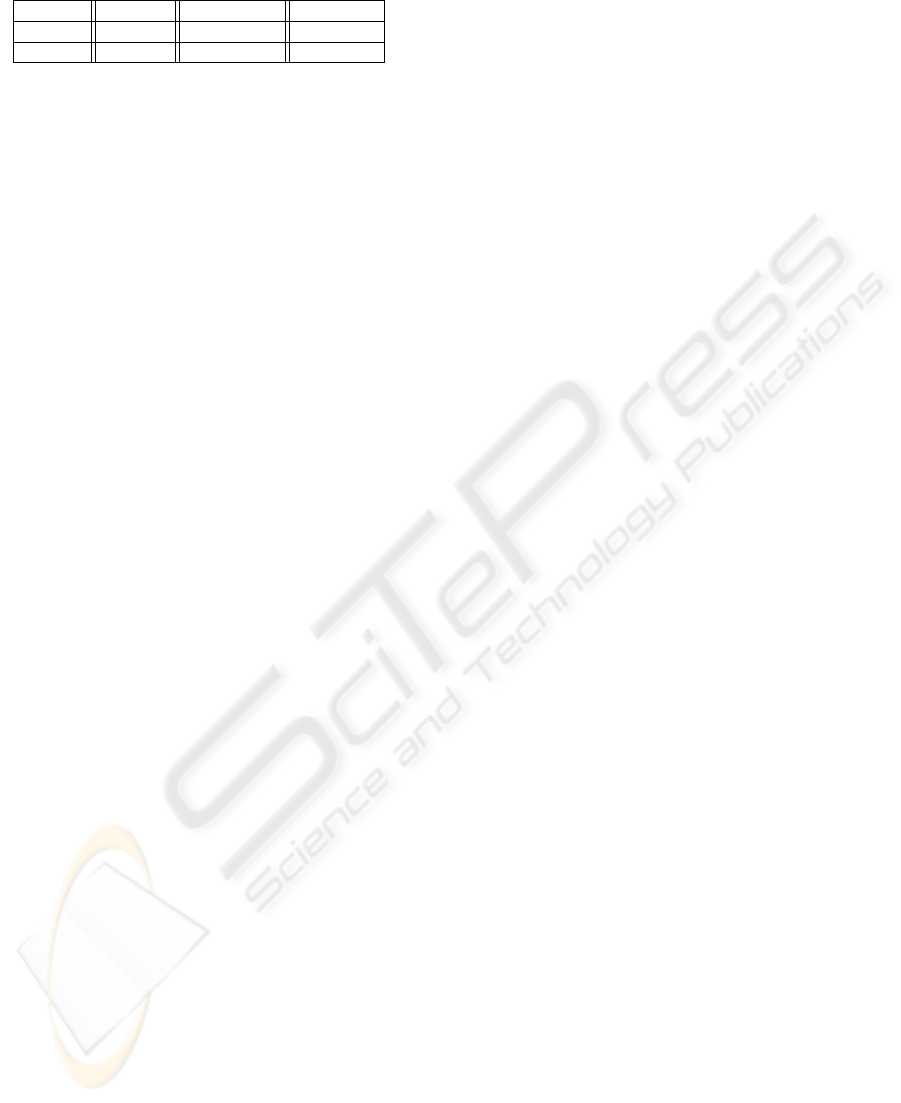
Table 3: Test of the Set of Rules.
Rule Support Confidence TP FP
R1 1.0% 23.5% 24 78
R1∪R2 2.1% 17.3% 38 181
support and confidence data.
The model evaluation is performed using ten-fold
cross validation (Witthen and Frank, 2000). This
kind of evaluation was selected to train the algorithms
using the entire data set and obtain a more precise
model. This will increase the computational effort but
improves the model’s capacity for generating differ-
ent data sets. The evaluation is performed by splitting
the initial sample in 10 sub-samples in order to fill
consumption range. The model is trained using 9/10
of the data set and tested with the 1/10 left. This is
performed 10 times on different training sets and fi-
nally the ten estimated errors are averaged to yield an
overall error estimate. The overall accuracy obtained
is around 80%.
3 CONCLUSIONS
This classification results can be interpreted in a prac-
tical way. This classification can be used to assign
new customers to existing classes and/or to inspect
customers that had not been previously inspected but
that belong to a class with a high rate of historical
NTL. In this last sense, Endesa staff action is required.
The Endesa staff, due to the extremely high cost
of the in-situ inspection for this class of customers,
usually only revises and inspects small samples (a
hundred or so medium-high consumption customers).
The quality of this framework is illustrated by a case
study that uses a real database. Only 188 of 10279
customers (less than 2%) of the selected registers
for mining present results of NTLs inspect. Regar-
less of the difficulty to study real data instead of
simulated data, rate of correct fraud identification
(about 20%) significatively improved previous com-
pany detection campaigns, refering to medium-high
consumption customers.
ACKNOWLEDGEMENTS
The authors would like to thank the Endesa Company
for providing the funds for this project (since 2005).
The authors are also indebted to the following col-
leagues for their valuable assistance in the project:
Gema Tejedor, Miguel Angel L
´
opez and Francisco
Godoy. Special thanks to Juan Ignacio Cuesta, Tom
´
as
Blazquez and Jes
´
us Ochoa for their help and cooper-
ation to extract the data from Endesa.
REFERENCES
Biscarri, F., Monedero, I., Le
´
on, C., Guerrero, J., Bis-
carri, J., and Mill
´
an, R. (June 12-16, Barcelona, Spain,
2008). A data mining method based on the variabil-
ity of the customers consumption. In 10th Interna-
tional conference on Enterprise Information Systems
ICEIS2008.
Cabral, J., Pinto, J., Gontijo, E. M., and Reis, J. (2004).
Fraud detection in electrical energy consumers using
rough sets. In 2004 IEEE International Conference on
Systems, Man and Cybernetics. IEEE press.
Cabral, J., Pinto, J., Linares, K., and Pinto, A. (2006).
Methodology for fraud detection using rough sets.
In 2006 IEEE International Conference on Granular
Computing. IEEE press.
Cabral, J., Pinto, J., Martins, E., and Pinto, A. (April 21-
24, 2008). Fraud detection in high voltage electric-
ity consumers using data mining. In IEEE Trans-
mision and Distribution Conference and Exposition
T&D. IEEE/PES.
Filho, J. and als (The Hague, The Netherlands, 2004.).
Fraud identification in electricity company costumers
using decision tree. In IEEE International Conference
on Systems, Man and Cibernetics. IEEE/PES.
Galv
´
an, J., Elices, E., noz, A. M., Czernichow, T., and Sanz-
Bobi, M. (Nov. 2-6, 1998). System for detection of
abnormalities and fraud in customer consumption. In
12th Conference on Electric Power Supply Industry.
IEEE/PES.
Jiang, R., Tagiris, H., Lachsz, A., and Jeffrey, M. (Oct. 6-10,
2002). Wavelet based features extraction and multi-
ple classifiers for electricity fraud detection. In Trans-
mission and Distribution Conference and Exhibition
2002: Asia pacific. IEEE/PES.
K.S.Yap, Hussien, Z., and Mohamad, A. (April 2-4, Phuket,
Thailand, 2007). Abnormalities and fraud electric me-
ter detection using hybrid support vector machine and
genetic algorithm. In Proceeding of the Third IASTED
International Conference Advances in Computer Sci-
ence and Technology. IASTED PRESS.
Sforna, M. (England, 2000). Data mining in power com-
pany customer database. In Electric Power Systems
Reseach, 55, 201-209. Elsevier Press.
Witthen, I. and Frank, E. (2000). Data Mining–Practical
Machine Learning Tools and Techniques with Java
Implementations. Morgan Kaufmann, Academic
Press, New York and San Mateo, CA.
A MINING FRAMEWORK TO DETECT NON-TECHNICAL LOSSES IN POWER UTILITIES
101