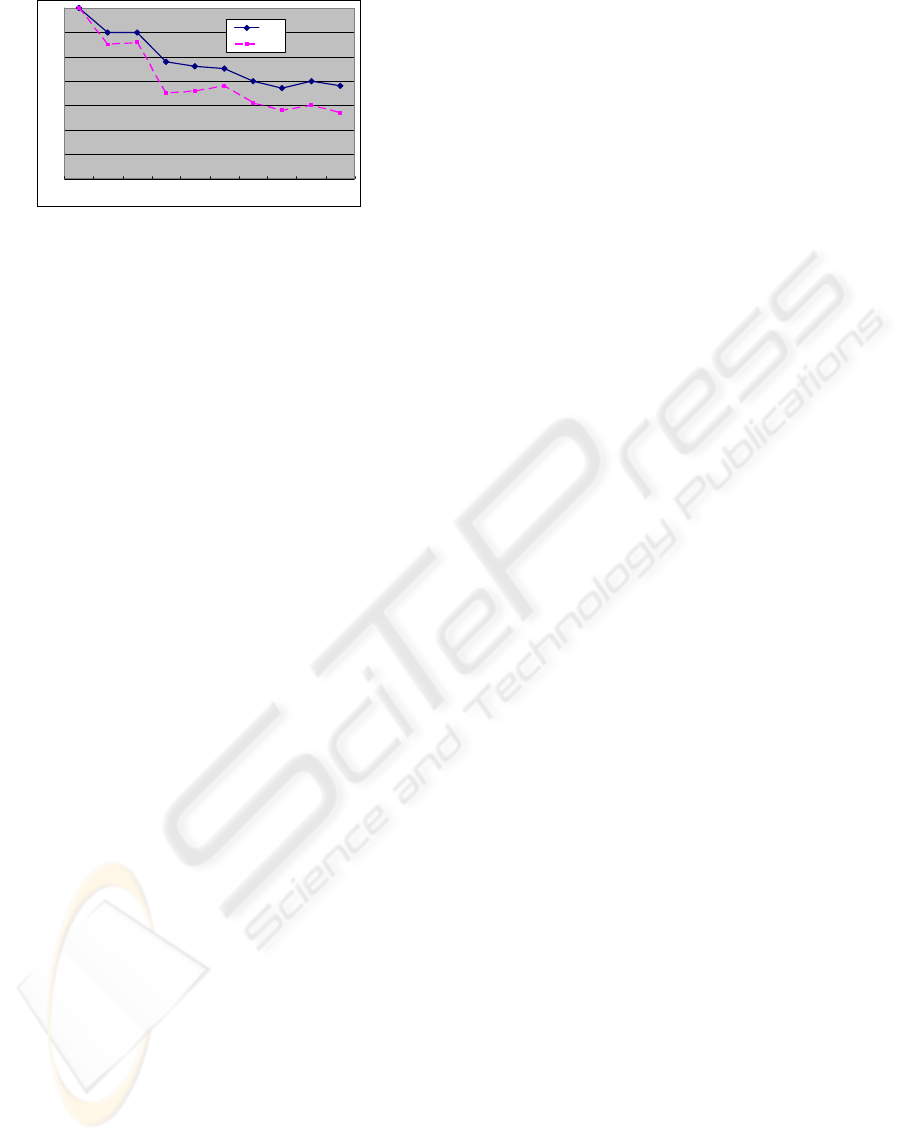
93
94
95
96
97
98
99
100
10 20 30 40 50 60 70 80 90 100
Number of Testing Images
Recognition Rat
GRA
MD
Figure 4: Recognition trends of different methods.
in C++ language under Borland C++ Builder 6.0
environment.
An experiment was conducted to demonstrate the
effect for the proposed two classifiers when the
number of the testing objects increased from 10 to
100 to reveal the effect of number of objects.
Combining synthetic and real object images, the
study had 1000 testing images for validation. The
experimental results is shown in Figure 4.
Accordingly, the GRA-based method outperformed
minimum distance method (MD), even though the
MD method still owned 95% of recognition rate
when the number of patterns reaches 100.
5 CONCLUSIONS
Deriving invariant features is a crucial task in the
area of pattern recognition. This study proposes a
new translation, rotation, and scaling-free 2D object
recognition method, which adopts K-curvature
boundary representation to derive position-invariant
property, re-sampling to achieve scaling-invariant
property, and autocorrelation transform to obtain
orientation-invariant property. In addition, the
proposed method incorporated with Grey Relational
Analysis method to recognize the 2D digital objects
with a high recognition rate. A set of fifty synthetic
images, each of them was acquiring with ten
different positions, orientations and scales, were
used for validation, and another fifty real objects
were obtained for validation. Experimental results
also reveal that the proposed method with either
GRA or MD methods is effective and reliable for
part recognition. Conclusively, the proposed KRA
feature extractor incorporated with GRA classifier,
not only successfully obtained position, orientation,
and scaling- invariant features, but also classified the
features in an excellent performance. It is also
expected that the proposed method may be applied
to various applications such as part sorting,
automated visual inspection, robot positioning,
control and monitoring system.
REFERENCES
Cao, W., Hao, F., & Wang, S. (2004). The application of
DBF neural networks for object recognition.
Information Sciences, 160, 153–160.
Chang, K.-C., Yeh, M.-F. (2005). Grey relational analysis
based approach for data clustering, IEE Proc.- Vis.
Image Signal Process 152 (2), April 2005, 165-172.
Chen, C.-M.; Chen, Y.-Y., Liu, C.-Y. (2007). Learning
Performance Assessment Approach Using Web-Based
Learning Portfolios for E-learning Systems. IEEE
Transactions on Systems, Man, and Cybernetics, Part
C: Applications and Reviews 37 (6), 1349 – 1359.
Deng, L.-L. (1982). Control problems of grey systems,
Systems and Control Letters 5(1), 288-294.
Deng, J.-L. (1989). Introduction to grey system theory,
Journal of Grey System 1, 1-24.
Huang, X., Wang, B., Zhang, L. (2005). A new scheme for
extraction of affine invariant descriptor and affine
motion estimation based on independent component
analysis. Pattern Recognition Letters, 26, 1244–1255. 499
Jiang, B.C., Tasi, S.L, Wang, C.C. (2002), Machine
vision-based grey relational theory applied to IC
marking inspection, IEEE Transaction on
Semiconductor Manufacturing 15 (4), 531-530.
Jones, G., III, & Bhanu, B. (2001). Recognizing
articulated objects in SAR images. Pattern
Recognition, 34, 469–485.
Khalil, M. I., & Bayoumi, M. M. (2002a). Affine
invariants for object recognition using the wavelet
transform. Pattern Recognition Letters 23, 57–72.
Khalil, M. I., & Bayoumi, M. M. (2002b). Invariant 2D
object recognition using the wavelet modulus maxima.
Pattern Recognition Letters, 21, 863–872.
Kung, C.-Y., Wen, K.-L. (2007). Applying Grey
Relational Analysis and Grey Decision-Making to
evaluate the relationship between company attributes
and its financial performance- A case study of venture
capital enterprises in Taiwan. Decision Support
Systems Volume: 43, Issue:3, April, 2007, pp.842-852
Kyrki, V., Kamarainen, J.-K., and Kavianen, H. (2004).
Simple Gabor feature space for invariant object
recognition. Pattern Recognition Letters, 25, 311–318.
Li, W., and Lee, T. (2004). Projective invariant object
recognition by a Hopfield network. Neurocomputing,
30, 1–18.
Popovici, V., & Thiran, J.-P. (2004). Pattern recognition
using higher-order local autocorrelation coefficients.
Pattern Recognition Letters, 25, 1107–1113.
Rosenfeld, A., Johnson, E. (1973), Angle detection on
digital curves, IEEE Transactions on Computers C-22,
875-878.
Sohn, K., Alexander, W.E., Kim, J.H., Snyder, W.E.
(1994) A constrained regularization approach to robust
corner detection. IEEE Transactions on Systems, Man,
and Cybernetics. 24 (5), 820-828.
Song Q.Y., Jamalipour, A. (2005). Network selection in an
integrated wireless LAN and UMTS environment
using mathematical modeling and computing
techniques. IEEE Wireless Communications 12(3), 42- 48
USING GRA FOR 2D INVARIANT OBJECT RECOGNITION
111