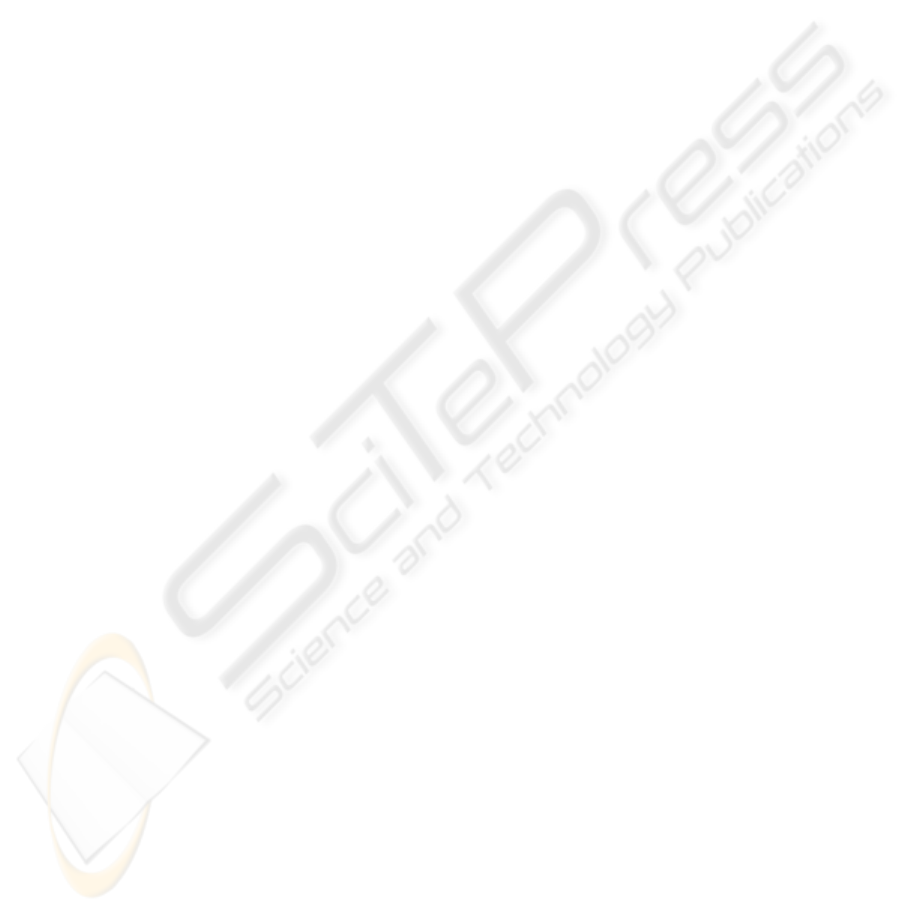
6 CONCLUSIONS
The CRAF system introduced in this paper is aimed
at addressing the problem of fast shifting customer
needs. In general, the system studies the dynamics of
pattern evolution to further perform data forecast.
The algorithm is specified to operate continuously so
as to track and to analyse the customer needs data
dynamically. Such characteristic is in contrast with
traditional methods that treat singular temporal
customer data in discrete approaches. It is
envisioned that the intelligence that could be derived
from the proposed system may serve to reduce the
uncertainties inherently found in product
development projects. In view of the increasingly
fast changing market, dynamic customer
requirement analysis and forecast (as well as the
applications of the generated intelligence on
downstream activities) are vital areas for future
research.
REFERENCES
Chen, C.-H., Yan, W., 2008. An in-process customer
utility prediction system for product conceptualisation.
Expert Systems with Applications. 34(4): 2555-2567.
Chong, Y.T., Chen, C.-H., Leong, K.F., 2009. Human-
centric product conceptualization using a design space
framework. Advanced Engineering Informatics,
Special Issue on Human Centered Product Design and
Development. 23(2), in press.
Dasgupta, D., 2006. Advances in artificial immune
systems. IEEE Computational Intelligence Magazine.
Nov 2006:40-48.
de Castro, L.N., Timmis, J., 2002. Artificial immune
systems: a new computational intelligence approach.
Heidelberg Germany : Springer-Verlag.
Herstatt, C., Stockstrom, C., Verworn, B., et al., 2006.
“Fuzzy front end” practices in innovating Japanese
companies in new product development. Journal of
Product Innovation Management. 3(1):43-60.
Kärkkäinen, H., Piippo, P., Puumalainen, K., Tuominen,
M., 2001. Assessment of hidden and future customer
needs in Finnish business-to-business companies.
R&D Management. 31(4): 391-407
Kurawarwala, A.A., Matsuo, H., 1998. Product growth
models for medium-term forecasting of short life cycle
products. Technological Forecasting and Social
Change. 57: 169-196.
Mittal, V., Kumar, P., Tsiros, M., 1999. Attribute-level
performance, satisfaction, and behavioral intentions
over time: A consumption-system approach. Journal
of Marketing. 63: 88-101.
Raharjo, H., Xie, M., Brombacher, A.C., 2006. Prioritizing
quality characteristics in dynamic quality function
deployment. International Journal of Production
Research. 44 (23): 5005-5018.
Reichwald, R., Stotko, C.M., Pillar, F.T., 2005.
Distributed mini-factory networks as a form of real-
time enterprise: concept, flexibility potential and case
studies. In: Kuhlin, B., Thielmann, H., (editors), The
practical real-time enterprise – facts and perspectives.
Springer Berlin Heidelberg. 403-434.
Timmis, J., Neal, M., 2001. A resource limited artificial
immune system for data analysis. Knowledge-based
Systems. 14: 121-130.
Xie, M., Tan, K.C., Goh, T.N., 2003. Advanced QFD
applications. ASQ Quality Press. Wisconsin.
AN INVESTIGATION INTO DYNAMIC CUSTOMER REQUIREMENT USING COMPUTATIONAL INTELLIGENCE
117