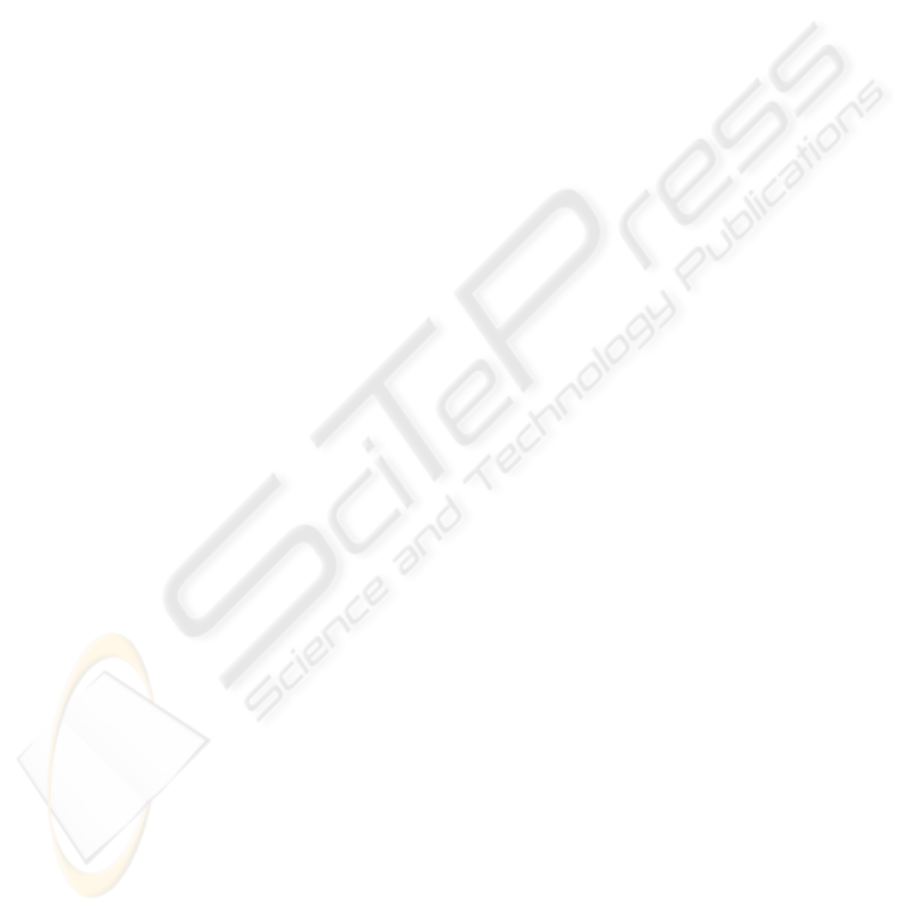
certain information. Since decision-making is usually
carried out in a distributed environment to achieve a
satisfactory solution, organizational learning and col-
laborative learning is another direction of need in fu-
ture study. Additionally, special attentions should be
given to the implementation of emergency manage-
ment system. As pointed out by several authors, an
interactive system is more realistic than a completely
automated system, in which human is responsible of
personnel knowledge specification, knowledge inter-
pretation and decision selection. The selection can
be performed through a multi-criteria evaluation from
several perspectives such as cost, effort, feasibility,
public acceptance, psychological and political impli-
cation, preference of decision makers (W. Raskob,
2005). Since EM managers are not experts on DM,
the comprehension is quite important for easy access,
e.g, derived rules are easily understandable and appli-
cable in decision reasoning. The scalability of DM
is worth noting for decision-making due to the infor-
mation flood occurring at the inception of emergency,
when real-time response becomes difficult.
ACKNOWLEDGEMENTS
The authors would like to acknowledge the financial
grant of GECAD/ISEP-Knowledge Based, Cognitive
and Learning Systems (C2007-FCT/442/2006).
REFERENCES
A. Aamodt, E. P. (1994). Case-based reasoning: Founda-
tional issues, methodological variations and system
approaches. Artificial Intelligence Communications,
1:39–52.
A. Gerbesioti, V. Delis, Y. T. S. A. (2001). Developing de-
cision support tools for confronting seismic hazards.
In Proc. of the 8th Panhellenic Conference on Infor-
matics, pages 247–253.
B. Yang, L.X. Lib, H. J. J. X. (2001). An early warning
system for loan risk assessment using artificial neural
networks. Knowledge-Based Systems, 14(5-6):303–
306.
Bienkowski, M. (1995). Demonstrating the operational fea-
sibility of new technologies: the arpi ifds. IEEE Ex-
pert, 10(1):27–33.
C. T. Lu, L.N. Sripada, S. S. R. L. (2005). Transportation
data visualization and mining for emergency manage-
ment. International Journal of Critical Infrastruc-
tures, 1(2/3):170–194.
E. Iakovou, C. D. (2001). An information management sys-
tem for the emergency management of hurricane dis-
asters. International Journal of Risk Assessment and
Management, 2(3-4):243–262.
G. Capuzzi, E. Cardinale, I. P. L. S. (2006). An incident re-
sponse support system. International Journal of Com-
puter Science and Network Security, 6(10):72–78.
J. L. Hellerstein, S. Ma, C.-S. P. (2002). Discovering ac-
tionable patterns in event data. IBM Systems Journal,
41(3):475–493.
J. Luo, L. Xu, J.-P. J. L. Z. Z. S. (2007). Flood decision
support system on agent grid: Method and implemen-
tation. Enterprise Information Systems, 1(1):49–68.
J. Z. Hernandez, J. S. (2001). Knowledge-based models for
emergency management systems. Expert Systems with
Applications, 20:173–186.
Kohonen, T. (1997). Self-organizing Maps. Springer Ver-
lag, Berlin.
K. S. Swarup, P. C. (2006). Power system static security
assessment using self-organizing neural network. J.
Indian Inst. Sci., 86:327–342.
L. Ji, H. Chi, e. (2006). Emergency Management. High
Education Press.
Lee, T.-L. (2008). Prediction of storm surge and surge de-
viation using a neural network. Journal of Coastal
Research, 24(sp3):76–82.
M. de la Asuncion, L. Castillo, F. J. G. A. P. F. (2005).
Siadex: an interactive knowledge-based planner for
decision support in forest fire fighting. AI Commu-
nications, 18(4):257–268.
P. Avesani, A. P. (1999). Cases on fire: Applying cbr to
emergency management. New Review of Applied Ex-
pert Systems, 6:175–190.
P. R. Cohen, M.L. Greenberg, D. H. A. H. (1989). Trial
by fire: Understanding the design requirements for
agents in complex environments. AI Magazine,
10(3):32–48.
Quinlan, J. (1993). C4.5: Programs for Machine Learning.
Morgan Kauffman, Los Altos, CA.
R. Agrawal, T. Imielinski, A. S. (1993). Mining association
rules between sets of items in large databases. In Proc.
of ACM SIGMOD, pages 207–216.
S. K. Harms, S. Goddard, S. R. W. W. T. T. (2001). Data
mining in a geospatial decision support system for
drought risk management. In Proc. of the National
Conference on Digital Government Research, pages
9–16.
U. Cortes, M.S. Marre, L. C. I. R. (2000). Artificial intel-
ligence and environmental decision support systems.
Applied Intelligence, 13:77–91.
Vandana, P. (2005). Dm-ams: Employing data mining tech-
niques for alert management. In Proceedings of Na-
tional Conference on Digital Government Research,
volume 89 of ACM International Conference Pro-
ceeding Series, pages 103–111.
W. Raskob, V. Bertsch, J. G. S. B. F. G. (2005). Demands
to and experience with the decision support system
rodos for off-site emergency management in the de-
cision making process in germany. In Proc. of the 2nd
International ISCRAM Conference.
Y. Atoji, T. Koiso, S. N. (2000). An information filter-
ing method for emergency management based on self-
organizing map. Human Interface, 2(3):1–6.
THE ROLE OF DATA MINING TECHNIQUES IN EMERGENCY MANAGEMENT
123