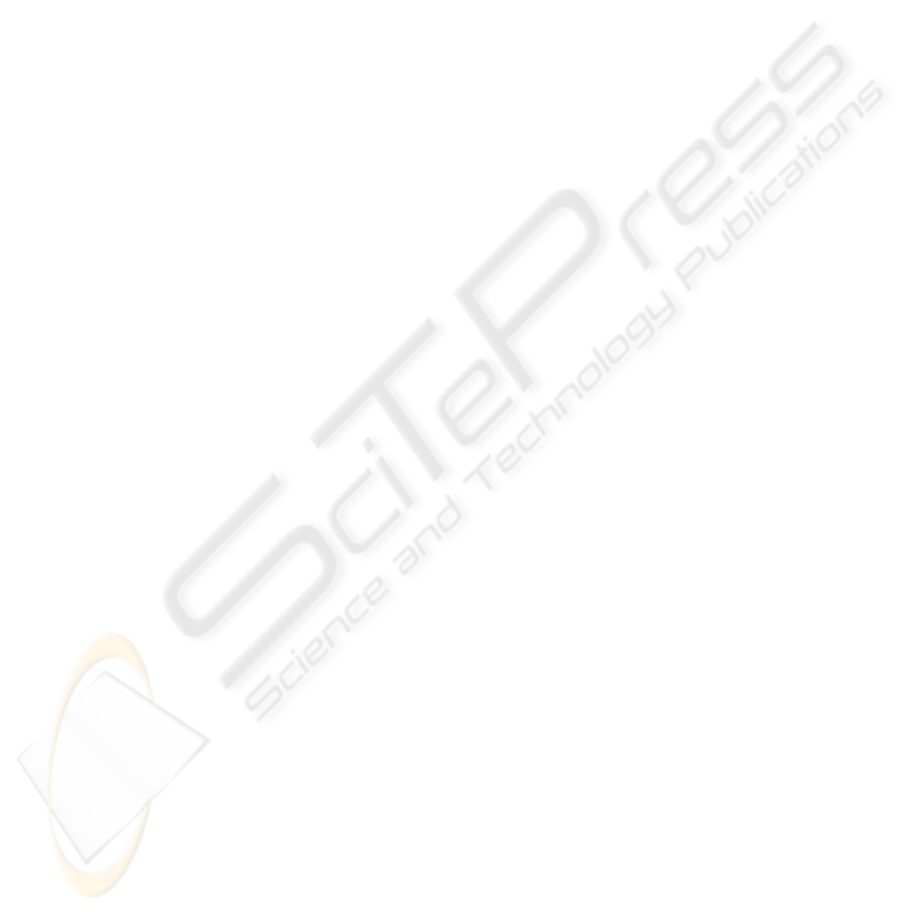
3 Steps of the Applied Method in the Task of Cytological
Specimen Analysis
The section shows how the steps of the method for image transformation selection in
recognition tasks [4] should be adapted for the task at hand. The corresponding
parameters are adjusted for each class, including image equivalence classes,
algorithms for image reduction to recognizable form, image model classes, and
recognition algorithms.
Step 1. Image Characterisation. Image equivalence classes are defined by the
recognition task at hand. Factor analysis shows [2] that feature descriptions of images
for different diagnosis have certain set of correlations, thus certain regularity or
mixture of regularities of different types characterise each class. Consequently, three
equivalence classes {I
1
}, {I
2
}, {I
3
} correspond to the diagnoses, CL, TCLL, and CLL.
Step 2. Image Model Construction. Initial images are described by feature sets.
Therefore variety {T
1
} of algorithms for image reduction to the recognizable form
consists of algorithms for feature calculation (similarly for {T
2
}, and {T
3
}). Note that
feature vectors for image description differ for images from different classes:
{I
1
}: { F2, F23, F25, F29 } ,
{I
2
}: { F22 – F29, F42, F45 } ,
{I
3
}: { F22 – F26, F29, F42, F45 } .
Step 3. Definition of Equivalence Class for Image Model. Since equivalence classes
for image models differ in natural way – feature sets vary for different classes,
equivalence class of image model is determined by the construction. So, by the
construction image model has the same class as the image selected on the first step.
Step 4. Image Model Classification. Notion of equivalence that was used on steps 1
and 2 was originally formulated for algorithms based on estimated calculations
(ACE), so we naturally use ACE for recognition. For experimental study we use
software system «Recognition 1.0» [1], it includes effective implementation of ACE
methods and supports its application for practical task solution. Experiments
demonstrate that the best results are achieved voting by all possible support sets. The
results of recognition are discussed in section 4.
Step 5. Verification of Image Characterisation. At the training stage we naturally
verify the correctness of image characterisation; since we know the correct class when
training, we just compare it with the class obtained. Verification for the recognition
stage is considered in detail at the next section.
It should be emphasized, that since recognition rates vary for the diagnoses, the
sequence of proposing hypothesis becomes essential. The general rule applied here is
as follows: we firstly assume that image belongs to the class with maximum number
of elements, then the second biggest class regarding number of its elements, and so
103