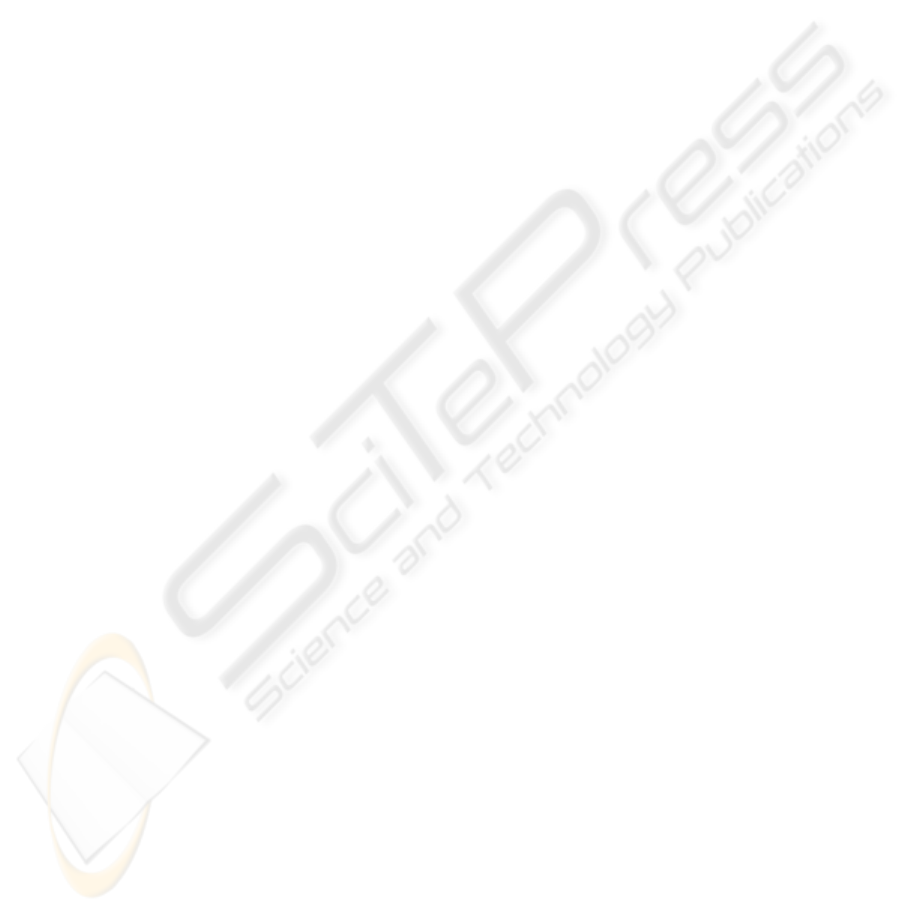
4 ENTERPRISE MANAGEMENT
The model supplies the user (and the agents) with a
set of generated reports, typical of Management and
enterprise analysis. The users, by reading and
analyzing them, can track down the influence of the
single decision – or even better the aggregate effects
coming from two or more decisions – on the
synthetic results, representing the monthly
performance of the whole enterprise.
According to the traditional use of the above
quoted modelling, the design of a whatever
economic system being simulated provides a
discussion of its results “at the end” of simulation,
and the traditional transfer of knowledge suffer from
the paradigm of “coeteris paribus” i.e. teaching is
concerned with the behaviour of just given variables
at a time, while keeping the remaining ones “still”.
On the contrary, in the presented approach, the
learner (or better, the team of learners) “drives” the
whole system by his own decisions during the
decision making process, learns through the
interaction and the process of “role playing” within
the group, and at the end of each month gets a set of
reports, that will constitute the basis for the
decisions to be taken during the following one.
The users, by reading and analyzing them, have
to track down the influence of the single decision –
or even better the aggregate effects coming from two
or more decisions – on the synthetic results,
representing the monthly performance of the whole
enterprise.
While the model has been originally conceived
as a teaching platform, in Universities and schools
for transmitting such concepts as “double-entry
accounting”, and the way in which the decisions
taken in a real enterprise affect the synthetic results,
at the end of each period (month), it can be
employed also as a simulator for managing
purposes. After tuning it basing on a certain
enterprise, it can be used as a what-if analysis tool,
i.e.: a simulator in which certain changes can be
done, in order to see how the system reacts to them,
before doing them in the real world.
The cognitive agents can help both when the
system is used as a teaching platform in schools, and
when it’s used as a real simulator. In fact, in the first
case, the agents can correct the common mistakes of
the students, by learning a correct policy (or, better,
a correct set of action for a given managing strategy)
and aid the students when they need to keep their
decision. For an example, it’s possible to think about
a simulated enterprise, in which the R&D
department is particularly weak, while the income is
high, but the products are getting old and – possibly,
will turn obsolete in few months. Unskilled students,
that of course are not used to manage real enterprise,
will probably get excited by the good and steady
incomes, and will likely not invest in the R&D
department. In few months, the competitors will
have better products on the market and suddenly the
enterprise will drastically diminish the sold
quantities. Unfortunately, it will be too late to start
an R&D campaign, since that’ll likely require
several months (or even year) to develop a new
competitive product from scratch.
A cognitive agent, when turned on, by means of
a dynamic hint could inform the students that their
product is in the maturity phase of its life cycle, and
that soon it’ll face a probable decline. If, that
notwithstanding, the students still do not invest in
R&D, then the agent will suggest this as a possible
strategy to prevent a forthcoming decline for the
product, that will lead to a likely drastic reduction in
the sales and will directly point to R&D as a way to
overcome this in advance, by developing new
products or improving the old ones.
When used a dynamic real-time simulator for
what-if analysis, the agent has a different task; since
we can aspect that users are, in this case,
experienced managers, the agent could help them in
finding the cause-effect relations basing on historical
data. For example, when the system is tuned on a
real enterprise, and empirically validated on its raw
data, it could be used to conduct a scenario analysis.
If we consider, for instance, the introduction of a
new machinery in a manufacturing enterprise, then
the system will keep track of its costs (variable and
fixed) and all the interactions it could have with the
rest of the environment (e.g.: required labour force,
energy, training and so on).
Though, without an intelligent system acting as a
supervisor, many of these relations will result in
black boxes, exactly as it happens in the real world.
An intelligent agent could supply step-by-step
explanations for that, by monitoring all the data
flow, through statistical and data-mining techniques.
Besides acting as a decision support system for
human learners experiencing with the model,
artificial agents can be used to supervise the decision
taken by learners, in order to interpret them in a
cognitive way.
For example, in the previously mentioned
enterprise accounting model, some users could
immediately pursue an high profit, while others
could be concerned first with the expansion of their
enterprise on new markets. Others could choose to
improve industrial plants, while others could want to
CSEDU 2009 - International Conference on Computer Supported Education
404