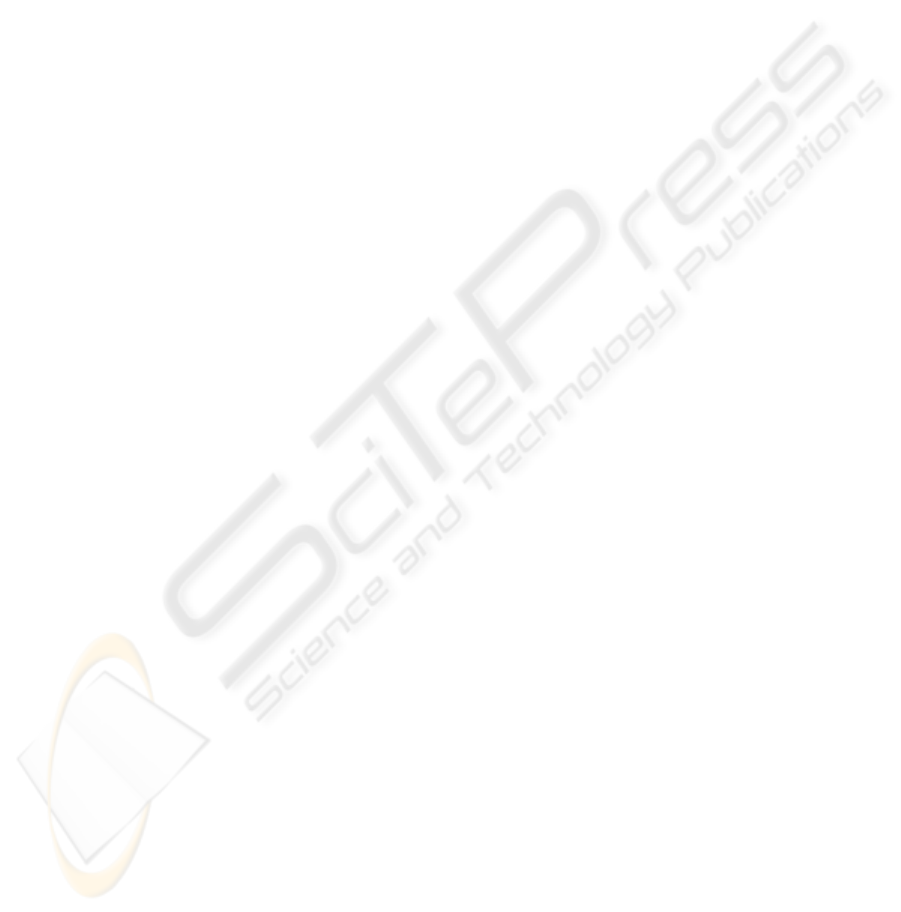
tended this dataset by adding several adjective scales
from examples in the literature, such as 4 adjective
scales from a teaching resource (Cadman, 2008). In
the exercises in the suggested resource, several verb
and adjective scales are provided, where the students
are requested to order them by strength. The dataset
of this kind is less confusing than adjective-sets in
WordNet that are not organized as scalable-sets to be-
gin with.
We relaxed each of the scales in our dataset manu-
ally into 2 antonymous subsets, where there were two
antonymous components and performed scaling, re-
sulting in 21 subsets. We compared the scaling results
by AdjScales with the same parameters as in section
3.1 to the expected scales as shown in Table 2.
4 RELATED WORK
A major work in differentiation between near-
synonyms in computational linguistics by (Inkpen
and Hirst, 2006) provides a list of types of nuances of
meaning that need to be differentiated, such as stylis-
tic, attitudinal etc. Using automatic methods to dif-
ferentiate between near-synonyms is the objective of
our research, and in this sense this work is relevant
to ours. Language learners have difficulty in perceiv-
ing the differences among near-synonyms and adding
distinctions on these subtleties to existing language
resources is needed. Currently, we focus only on dif-
ferentiation of similar adjectives by strength. Adding
further types of differentiation is a much needed ex-
tension of our work in the future. Differently from
Inkpen and Hirst that use a collection of machine
readable dictionaries, we use the Web as a corpus.
(Hatzivassiloglou and McKeown, 1993) estab-
lished the first step towards automatic identification
of adjective scales. They provide an excellent back-
ground on adjectives and a general plan to identify
adjective scales, though, they focus only on cluster-
ing of similar adjectives.
Using patterns extracted from large corpora in or-
der to learn semantic relations between words is a
common approach in computational linguistics. The
pioneering work (Hearst, 1992) extracted hyponym
(IS-A) and meronym (PART-OF) relations. Further
studies (Chklovski and Pantel, 2004; Davidov and
Rappoport, 2008; Turney, 2008) intensively extend
this methodology, further relations are explored, su-
pervised and unsupervised methods are introduced.
Our work belongs to this school of relation extraction.
VerbOcean (Chklovski and Pantel, 2004),
explores fine-grained relations between verbs,
STRONGER-THAN being one of them. Their work is
very similar to ours in using lexico-syntactic patterns
extracted from the Web. Their selection of patterns is
manual, and it is based on training on 50 verb pairs,
with a total of 8 patterns selected for the STRONGER-
THAN relation. We utilize the asymmetry of the
STRONGER-THAN relation in a similar manner to
VerbOcean. We differ in our focus on adjectives and
in our evaluation procedure. VerbOcean, providing
differentiation between similar verbs, should be
considered in the context of language learners.
A large body of research (Turney and Littman,
2003; Popescu and Etzioni, 2005) has been conducted
in the field of opinion mining. An important dis-
tinction for opinion mining is semantic orientation
(positive, negative, or neutral) of words and utter-
ances. In this work we do not distinguish between
the positive or the negative senses of adjectives, but
rather make a more general distinction of the extent
of adjectival descriptive strength. We also have a dif-
ferent objective to provide linguistic distinction be-
tween synonymous adjectives for learners, while the
research in opinion mining concentrates on strength
of subjectivity and sentiment of words, and texts.
One of the main approaches in opinion mining is
extraction of semantic information from the Web, and
typically adjectives play a central role in understand-
ing opinion from texts. In these aspects, this field
is related to our work. According to (Turney and
Littman, 2003) semantic orientation of a word, in ad-
dition to its direction also comprises intensity, mild or
strong. They compute intensity in a combined com-
putation of the direction, using statistical association
with a set of positive and negative paradigm words.
OPINE (Popescu and Etzioni, 2005), a system for
product reviews mining ranks opinion words by their
strength as one of its subtasks. Both of these works
focus on detection of semantic orientation of words
and report on a very limited evaluation of ranking by
strength.
No previous work that we are aware of proposes
an automatic method to identify adjective-scales for
language learners.
5 DISCUSSION
We have presented AdjScales, a method to build ad-
jective scales from several examples of similar ad-
jectives using a state-of-the-art methodology of ex-
tracting relations using patterns over the Web. It is
simple, and the only required resource (for Scaling
step, which is the main focus of this work) is access
to a search engine. Overall, as can be seen from the
evaluation, AdjScales scales similar adjectives only
CSEDU 2009 - International Conference on Computer Supported Education
234