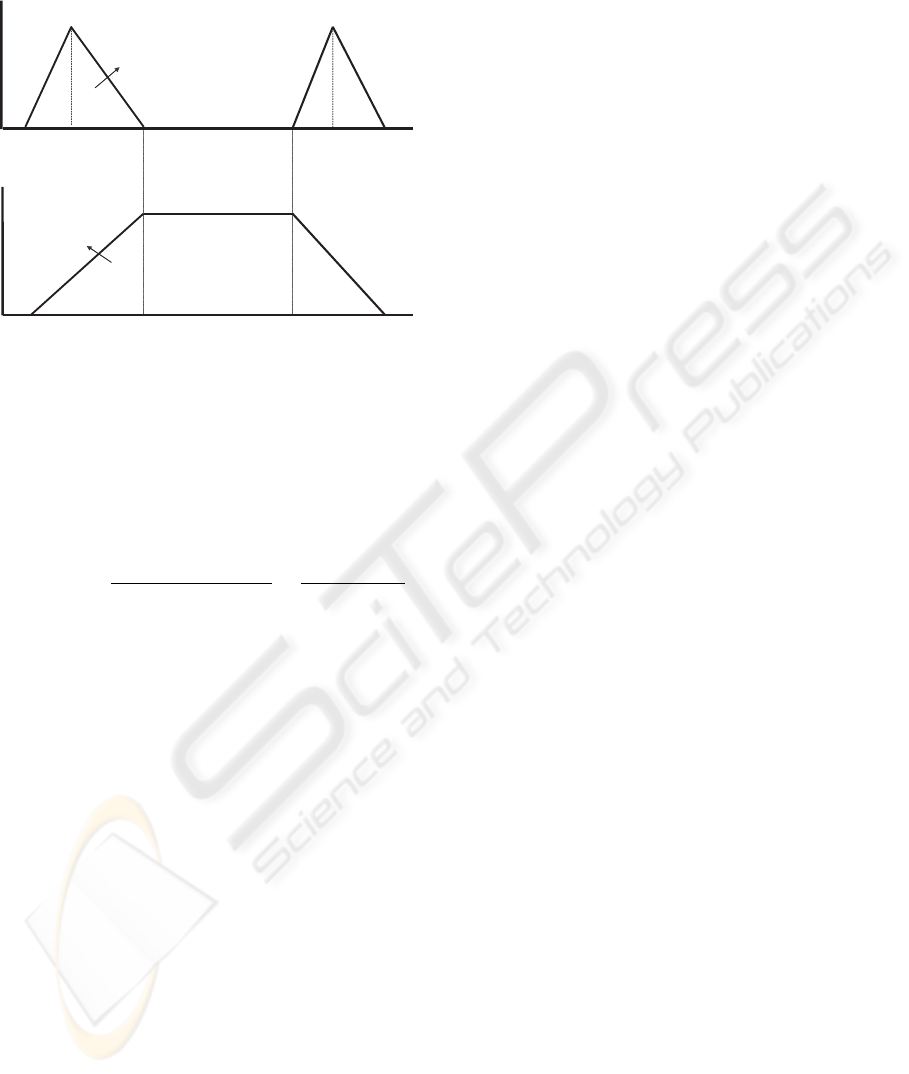
The posed problem is shown in a graphical way in
figure 6.
ds-as ds ds+bs de-ae de de+be
Starting Date Ending Date
Initial Data
d1-a d1 d2 d2+b
Transformed Data
S1
S2
Figure 6: Transformation of two fuzzy dates into a fuzzy
period preserving imprecision.
The resulting fuzzy interval is obtained by means
of the equality S
1
= S
2
that obliges to maintain the
same amount of imprecision after the transformation
is performed.
S
1
= S
2
=⇒
(d
s
+ b
s
) − (d
s
− a
s
)
2
=
d
1
− (d
1
− a)
2
If we assume that the data associated to this time
specification are precisely known from (d
s
+ b
s
) to
(d
e
− a
e
), then d
1
= d
s
+ b
s
and both terms become
equal and d
1
− a = d
s
− a
s
, as shown in figure 6. The
same substitution should be made to obtain the right
part of the interval.
As it was explained in section 1.2, it is quite easy
to represent a fuzzy interval with this characteristics
since only four parameters need to be stored in order
to specify it. In (Medina et al., 1994) (Medina et al.,
1995) is presented a generalized model of fuzzy DB
that supports this representation for fuzzy data and the
corresponding implementation in a classical relational
DB system (Oracle).
3 CONCLUSIONS AND FUTURE
WORK
In this paper we have shown how to represent differ-
ent time specifications in a unified way. The repre-
sentation considered is the fuzzy interval, which re-
sults very suitable for both precise and imprecise time
points and periods when the time is interpreted as
valid time. For the case that two fuzzy dates are pro-
vided by the user, it is necessary to perform a transfor-
mation to convert this original time information into a
fuzzy interval that preserves the imprecision involved.
ACKNOWLEDGEMENTS
This work has been partially supported by research
projects TIN2008-02066/TIC and P07-TIC-03175.
REFERENCES
Barro, S., Mar
´
ın, R., Mira, J., and Pat
´
on, A. R. (1994). A
model and a language for the fuzzy representation and
handling of time. Fuzzy Sets Syst., 61(2):153–175.
Bettini, C., Wang, X. S., and Jajodia, S. (1998). A general
framework for time granularity and its application to
temporal reasoning. Annals of Mathematics and Arti-
ficial Intelligence, 22(1-2):29–58.
Chountas, P. and Petrounias, I. (2000). Modelling and rep-
resentation of uncertain temporal information. Re-
quirements Eng., 5:144–156.
Clifford, J. and Rao, A. (1987). A simple, general structure
for temporal domains. In Temporal Aspects in Infor-
mation Systems, pages 17–28.
de Feri
´
et, J. K. (1973). La theorie generalise
´
ede
l’information et le mesure subjetive de l’information.
In de F
`
eriet, K. and Picard., C., editors, Lecture notes
in Math: Th
´
eories de l’information., volume 398,
pages 1–28. Springer-Verlag.
Dubois, D. and Prade, H. (1987). Fuzzy numbers: An
overview. In Bezdek, J., editor, Analysis of Fuzzy In-
formation, Vol.I: Mathematics and Logic, pages 3–39.
CRC Press, Boca Raton, FL.
Elmasri, R. and Wuu, G. T. J. (1990). A temporal model and
query language for er databases. In Proceedings of the
Sixth International Conference on Data Engineering,
pages 76–83, Washington, DC, USA. IEEE Computer
Society.
Goguen, J. (1967). L-fuzzy sets. Journ. of Math. Anal. and
Applications, 18:145–174.
Gonz
´
alez, A., Pons, O., and Vila, M. A. (1999). Dealing
with uncertainty and imprecision by means of fuzzy
numbers. Int. J. Approx. Reasoning, 21(3):233–256.
Medina, J. M., Pons, O., and Vila, M. A. (1994). Gefred: a
generalized model of fuzzy relational databases. Inf.
Sci. Inf. Comput. Sci., 76(1-2):87–109.
Medina, J. M., Vila, M. A., Cubero, J. C., and Pons, O.
(1995). Towards the implementation of a general-
ized fuzzy relational database model. Fuzzy Sets Syst.,
75(3):273–289.
Yager, R. (1981). Measurement of properties on fuzzy sets
and possibility distributions. In Klement, E., editor,
3rd Intern. Seminar on Fuzzy Set Theory, pages 211–
222. Johannes Keplas Univ. Liuz.
ICEIS 2009 - International Conference on Enterprise Information Systems
358