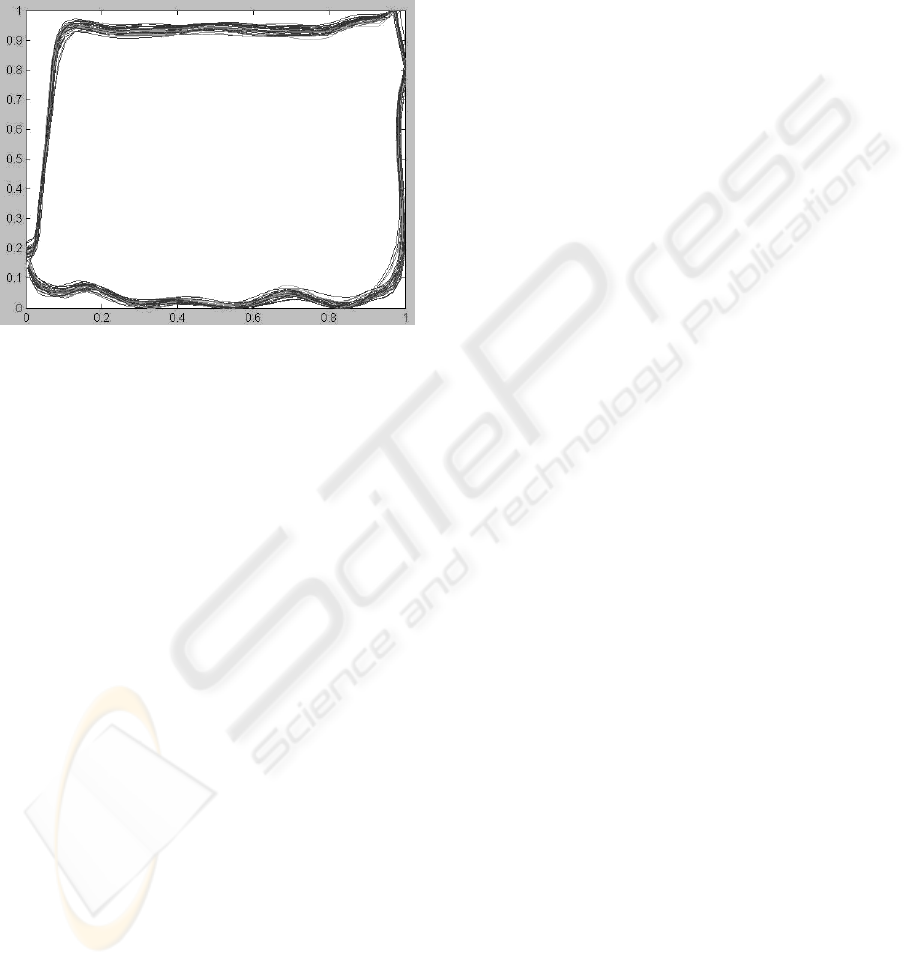
a) 11 card were not classified since the neurons
at the output layer held values below the limit
established by the criteria (0,1).
b) 69 cards were wrongly classified. However,
from these 69 cards, 30 of them were cards pre-
diagnosed as “plunger hitting top” when in fact they
were “normal”
Figure 8: Error analysis in the classification.
When the above results were shown to a human
expert, he observed that he would have considered
as an acceptable diagnosis produced by the artificial
neural network. That represents a considerable
improvement in the results.
Summing it up, from the 6,101 cards which were
tested, the total classification error was found to be
1,31%.
5 CONCLUSIONS
The present work shows that the generation of
artificial standards for training neural networks in
order to analyze the pump mechanic system in oil
elevation is feasible. The results shown here leave
open the possibility of creating a scheme that not
only incorporate the complete set of classes of
anomalies, but which is also able to integrate the
data base of real automation systems.
ACKNOWLEDGEMENTS
To PETROBRAS for letting available information
on real data for realizing the tests here. Also to the
Graduation Program in Mechatronics of UFBA
(Universidade Federal da Bahia) for the support
given.
REFERENCES
Abello, J., A. Houang and J. Russell. (1993). A Hierarchy
of Pattern Recognition Algorithms for Diagnosis of
Sucker Rod Pump Wells, IEEE 08186-4212-2/93, pp
359-364.
Derek, H. J., J. W. Jennings and S. M. Morgan. (1988).
EXPROD: Expert Advisor Program for Rod Pumping,
SPE 17318.
Dickinson, R. R. and J. W. Jennings. (1988). The Use of
Pattern Recognition Techniques in Analyzing
Downhole Dynamometer Cards, SPE 17313.
Eickmeier, J. R. (1966). Applications of the Delta II
Dynamometer Technique, 17th Annual Technical
Meeting, The Petroleum Society of C.I.M., Edmonton,
maio/1966.
Haykin, Simon (1998). "Neural Networks: A
Comprehensive Foundation", Second Edition. Prentice
Hall.
Foley, W. L. and J. G. Svinos. (1987). EXPROD: Expert
Advisor Program for Rod Pumping, SPE 16920.
Gibbs, S. G. and A. B. Neely. (1966). Computer Diagnosis
of Down-Hole Conditions in Sucker Rod Pumping
Wells, Journal of Petroleum Technology, jan/1966, pp
91-97.
Gilbert, W. E. (1936). An Oil-Well Pump Dynagraph.
Production Practice, Shell oil Co., pp 94-115.
Schinitman, L., G. S. Albuquerque, J. F. Corrêa, H.
Lepikson and Bitencourt, A. C. (2003). Modeling and
implementation a system for sucker rod downhole
dynamometer card pattern recognition, SPE 84140.
Xu, P., S. Xu and H. Yin. (2006). Application of self-
organizing competitive neural network in fault
diagnosis of suck rod pumping system, Journal of
Petroleum Science & Engineering, 58 (2007) pp 43-
48.
PATTERN RECOGNITION FOR DOWNHOLE DYNAMOMETER CARD IN OIL ROD PUMP SYSTEM USING
ARTIFICIAL NEURAL NETWORKS
355