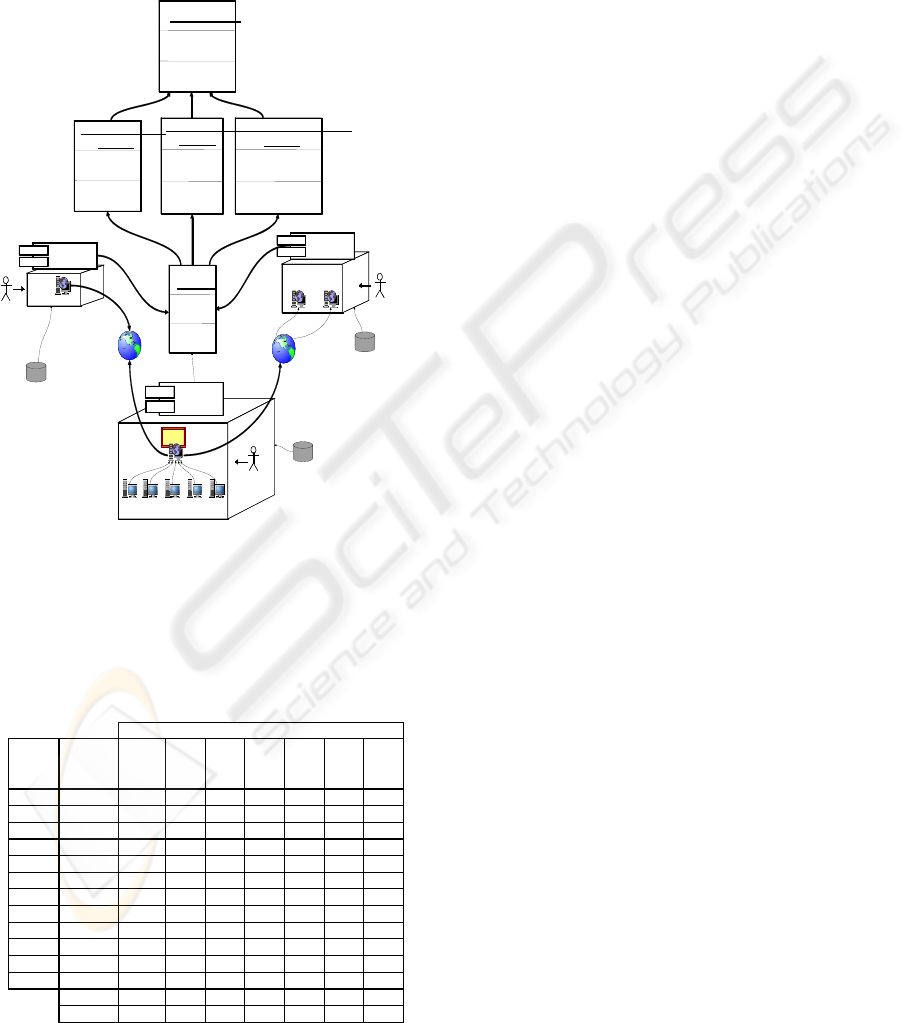
3.1 Main Results and Discussions
Therefore with the simulated agent-based model, the
results (Table 1) aim to highlight the forecasting
deviaton in terms of the collaborative configuration
which has been assigned to differents nodes. In
Figure 2, these are the instantiated classes that carry
out the ACLMESSAGES.
N1
N11
N17
N12
N14
N13
N15
N31N32
JADE
Container 1
JADE
Container 2
JADE
Container 3
1
1
1
N
N
N
Network1
1
1
1
N
N
N
ACLMESSAGE()
Forecasting
agen
Collaborative
agent
Non-Collaborative
agent
User 2
User 3
User 1
Supply chain
network 1
Supply chain
network 2
Supply chain
network 3
Information
repository
FIPA-PROTOCOLS
(AchieveRE)
Network2
Setup() Setup() Setup()
Behaviour
Main
Setup()
FIPA-PROTOCOLS
(AchieveRE)
FIPA-PROTOCOLS
(AchieveRE)
Information
repository
Information
repository
Demand plan
firm orders
Demand plan/
firm orders
Figure 2: Particular decentralized supply chain network
agent-based model (a UML-based model approach).
According to Table 1, a clear impact caused by
increasing the collaborative level has been observed
in the forecasting deviation.
Table 1: Deviation of the forecasted demand.
Periods
TOTAL
real
orders
S9
(100%)
S8
(88%)
S7
(75%)
S6
(63%)
S5
(50%)
S2
(13%)
S1
(0%)
61 112 ‐1 ‐14,2 ‐28 ‐44,4 ‐44 ‐55,4 ‐41,8
62 85 ‐3 ‐12,2 ‐19 ‐12,4 ‐8 ‐22,4 ‐14,8
63 88 4 ‐11,2 ‐11 ‐19,4 ‐23 ‐22,4 ‐17,8
64 82 3 ‐2,2 ‐8 ‐14,4 ‐19 ‐20,4 ‐11,8
65 80 ‐2 ‐2,2 ‐6 ‐12,4 ‐22 ‐23,4 ‐9,8
66 102 2 ‐4,2
‐8 ‐16,4 ‐25 ‐44,4 ‐32,8
67 66 ‐1 4,8 7 17,6 11 4,6 4,2
68 74 12,8‐2 ‐14,4 ‐7 ‐7,4 ‐3,8
69 123 2 ‐2,2 ‐19 ‐30,4 ‐40 ‐50,4 ‐53,8
70 68 2 ‐11,2 ‐4 ‐12,4 ‐18 ‐8,4 2,2
71 113 ‐3 ‐16,2 ‐30 ‐31,4 ‐44 ‐56,4 ‐43,8
72 83 ‐2 ‐5,2 ‐30,6‐9 ‐13,4 ‐13,8
Average 0,2 ‐6,1 ‐10,9 ‐15,8 ‐20,7 ‐26,7 ‐19,8
Std.Dev. 2,4 6,8 11,0 15,7 16,5 20,3 18,9
Collaborativelevel
4 CONCLUSIONS
A collaborative forecasting agent-based model to
support the decentralized supply chain has been
proposed. Thus, under the supply chain network
supported by multiagent systems, the deviation was
lower compared with the real demand than the
traditional forecasting process when considering a
collaborative forecasting demand. In addition, full
collaboration among the nodes was not seen to be
necessary, but at list of more than a half of them to
collaborate is needed to generate real contribution
from this collaborative forecasting process. In future
research, the proposed model will be applied to a
real supply chain network in the automobile supply
chain sector, and will consider real demand data.
ACKNOWLEDGEMENTS
This research has been carried out in the framework
of a project funded by the Spanish Ministry of
Science and Education, entitled ‘Simulation and
evolutionary computation and fuzzy optimization
models of transportation and production planning
processes in a supply chain. Proposal of
collaborative planning supported by multi-agent
systems. Integration in a decision system.
Applications” (EVOLUTION project, DPI2007-
65501, http://www.cigip.upv.es/evolution).
REFERENCES
Aviv, Y. (2001). The Effect of Collaborative Forecasting on
Supply Chain Performance. Management Science,
47(10): 1326–1343.
Filipe, J. (2003). Information Fields in Organization Modeling
Using an EDA Multi-agent Architecture. Agent-Mediated
Knowledge Management, 2926: 130-142.
Hernández, J.E, Mula, J., and Ferriols, F.J. (2008). A
reference model for conceptual modelling of production
planning processes. Production Planning & Control,
19(8): 725–734.
Liang, W. Y., and Huang, C. C. (2006). Agent-based demand
forecast in multi-echelon supply chain. Decision Support
Systems, 42: 390–407.
Poler, R, Hernandez, J. E, Mula, J., and Lario, F.C. (2008).
Collaborative forecasting in networked manufacturing
enterprises. Journal of Manufacturing Technology
Management, 19(4): 514-528.
Rodriguez, R., Poler, R., Mula, J., and Ortiz, A. (2008).
Collaborative forecasting management: fostering
creativity within the meta value chain context. Supply
Chain Management: An International Journal, 13(5):
366–374.
MODELLING COLLABORATIVE FORECASTING IN DECENTRALIZED SUPPLY CHAIN NETWORKS WITH A
MULTIAGENT SYSTEM
375