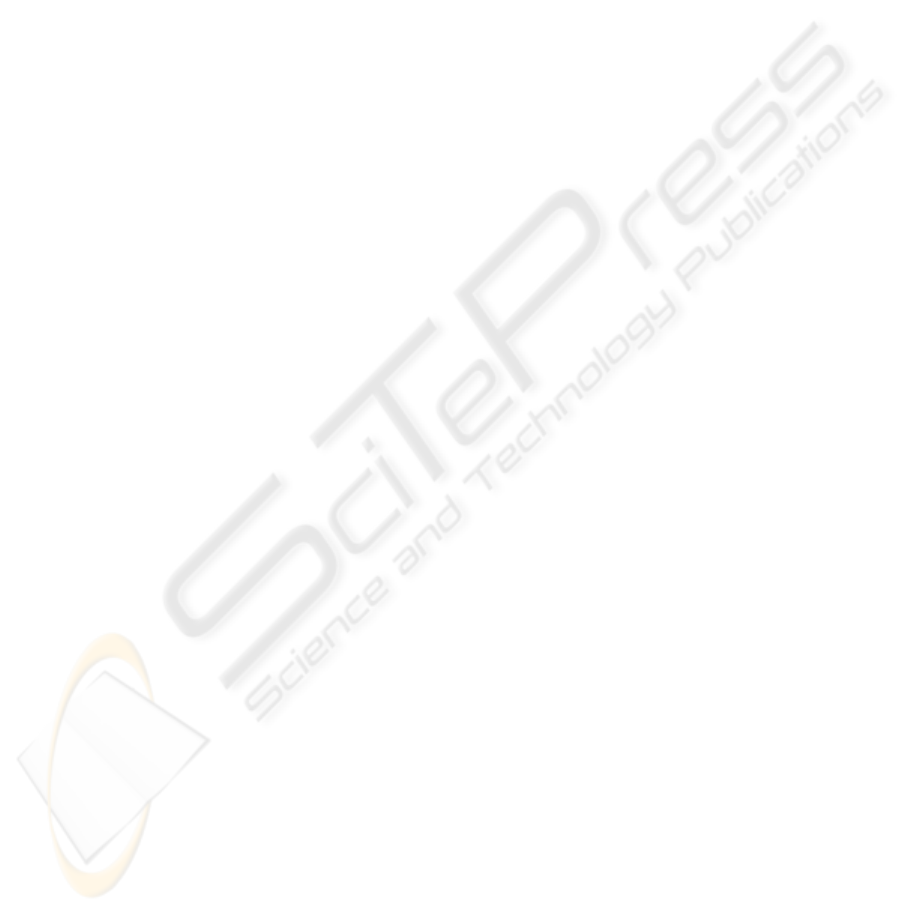
5 EXPECTED RESULTS
Our research attempts to shed light to the field of
negotiation optimization, by focusing on the
acquisition and application of negotiation routines.
It is commonly stated that the main problem of
routinization is the risk of applying ineffective
routines. As discussed earlier, among the causes that
produce inflexible routines lays a repetitive
mechanism that prohibits further questioning or
evaluation of the acts. We trust that our framework
makes contribution to theory of negotiation routines
by presenting two essential directions for breaking
routine rigidity. The first concerns the identification
of the need of external evaluation after each routine
has been applied and the second concerns the
enrichment of the system core with an evolving
structure that adjusts its functionality to
accommodate new knowledge. The first direction
actually indicates the necessity to keep an open
communication channel with the environment, and
allow for the notification and triggering of routine
change. The second direction concerns the
identification of an appropriate structure that
embraces evolving processes, in order to absorb new
knowledge and proceed to routine change.
To this extent we have outlined four desirable
characteristics in section 4 that are met in our
proposed framework. The first concerns a hypothesis
of the representation of negotiation context and
routines as vector pairs. The second identifies the
need of external routine evaluation and dynamic
system structure. Our framework suggests the
integration of the dynamic system structure (ECOS)
with a negotiation agent, in order to allow the
interaction of the model that captures knowledge and
extracts routines with the negotiators, and be
notified in terms of a utility measure (after routine
application). The mediator agent will be motivated,
if the utility decreases, to request further planning by
the negotiators. The results (new knowledge) will
then be inserted to the dynamic structure. The third
and fourth characteristics concern the system’s
ability to develop in open space and quickly adapt to
the environment. These characteristics are met by
the ECOS structure, since it learns and partitions the
problem space locally. Furthermore in section 3 we
have identified several limitations posed by the
application of AI techniques in evolving processes.
These are addressed by ECOS algorithms, since they
apply fast one-pass learning (adaptation) and are
resistant to catastrophic forgetting (Kasabov, 2007).
Their structure is simple and grows in terms of
adaptation to the environment (the eMLP grows by
the addition of new nodes to the evolving and output
layer). Finally ECOS systems evolve different types
of knowledge representation from data; therefore our
representational hypothesis is not limited.
This framework is completely new and will be
tested in several stages of negotiation to provide
support to negotiators by the extraction of routines.
We trust that since the system is evolving it will lead
to efficient results in prenegotiation processes
(strategy formulation, commitment to rules,
opponent observation, issues and problem
formulation, other prenegotiation convensions), as
well as in negotiation processes (decision of
proposal and negotiation locutions). We have made
the hypothesis that knowledge can be provided to
the system in pairs of x, y vectors therefore the
model is generic and can be used for the substitution
of parts or even whole negotiations. Our future
research concerns extensive tracing of negotiation
repetitive acts, and the development and application
of our model in those that can be modeled as vector
pairs. If in several cases uncertainty is introduced to
vector dimensions and we need to address the issue
of missing values, the framework can be extended to
contain evolving fuzzy neural network (EFuNN)
instead of simple eMPL in its dynamic structure.
EFuNNs, function as eMLPs but have two extra
connection layers that represent fuzzy input and
output spaces. For these cases we will investigate the
integration of EFuNNs to our mediator framework.
Model validation, in terms of the generalization
ability of the ECOS core to produce good results on
new, unseen data samples, can be implemented by
splitting the original dataset to train and test sets and
calculating the actual error of the system. The most
commonly stated validation methods are simple train
and test split of data, k-fold cross validation and
leave-one-out cross validation. Furthermore, the
proposed structure itself suggests external evaluation
of the applied routines by the negotiators, which
contributes in measuring the overall system
performance.
REFERENCES
Braun, P., Brzostowski, J., Kersten, G., Baek Kim, J.,
Kowalczyk, R., Strecker, R & Vahidov, R 2005, ‘E-
Negotiation Systems and Software Agents: Methods,
Models and Applications’, in J. Gupta, G. Forgionne
& M. Mora (eds), Intelligent Decision-Making
Support Systems: Foundation, Applications, and
Challenges, Springer, London.
INTEGRATING AGENTS WITH CONNECTIONIST SYSTEMS TO EXTRACT NEGOTIATION ROUTINES
255