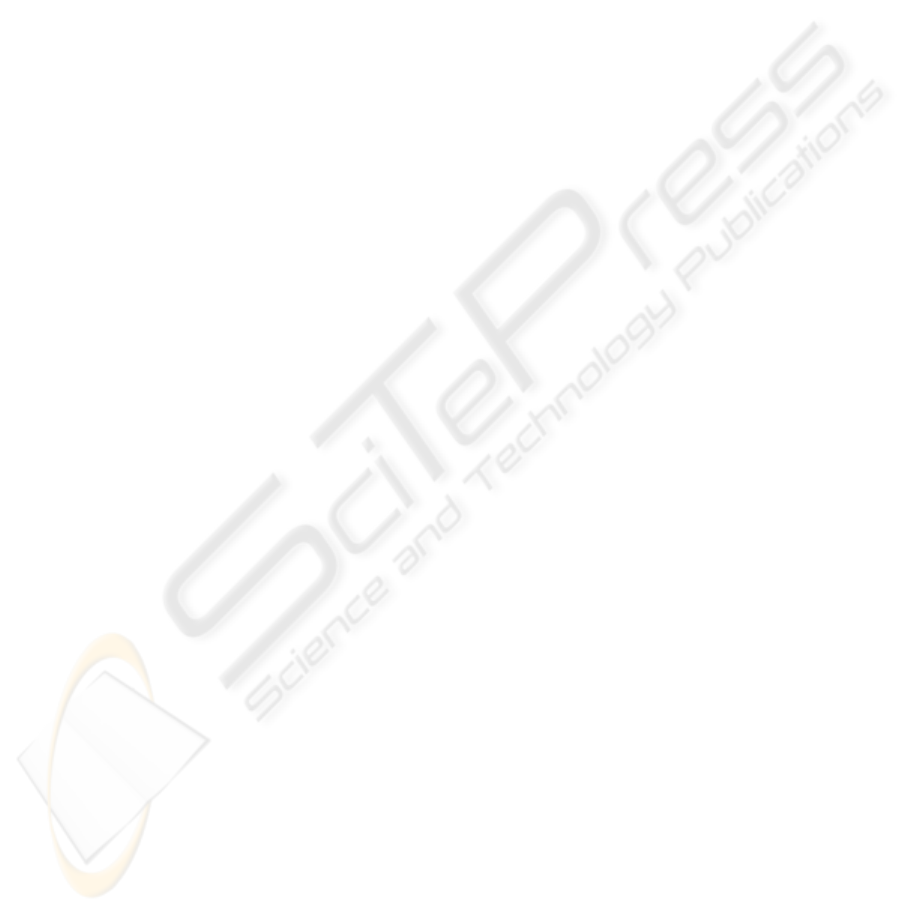
6 DISCUSSION
Granularity is a challenging topic in artificial intel-
ligence and education, both from a theoretical view-
point (e.g. (Hobbs, 1985; Keet, 2008)) but also in
several applications, for example in the computer-
assisted teaching of programming skills (Mccalla
et al., 1992), or in the modeling of biological infor-
mation systems (Keet, 2008).
In this paper, we have sketched a flexible, adap-
tive approach for modeling and assessing proof step
granularity. It is based on the collection of empiri-
cal data from the observed behavior of expert tutors,
which is then modeled via artificial intelligence and
data mining techniques. These models for granular-
ity can be generated independently of whether the ex-
perts are able to introspect or justify their judgments.
The learnt classifiers serve to imitate the mathemat-
ical practice of the experts (pertaining to granular-
ity) when used within an intelligent tutoring system.
An alternative approach would be to establish an ex-
plicit best practice of judging proof step granularity
by openly engaging tutoring experts in the discus-
sion of the involved cognitive dimensions. It remains
debatable which of the two approaches is more ade-
quate for building a granularity-informed proof tutor-
ing system, and we consider our work and our system
environment as a fruitful first step in both directions.
Future work will address the questions raised in
the introduction. Among other things this is depen-
dent on the successful completion of our ongoing ex-
periments.
ACKNOWLEDGEMENTS
We thank the members of the ΩMEGA and DIALOG
research teams at Saarland University for their in-
put and their feedback on this work. Furthermore,
we thank Erica Melis and her ActiveMath group for
valuable institutional and intellectual support. We are
thankful to three anonymous reviewers for their help-
ful comments, to Marc Wagner for internal review and
to Mark Buckley for proof-reading of the paper.
REFERENCES
Autexier, S. (2005). The core calculus. In Nieuwen-
huis, R., editor, Automated Deduction - CADE-20,
20th International Conference on Automated Deduc-
tion, Tallinn, Estonia, July 22-27, 2005, Proceedings,
volume 3632 of LNCS, pages 84–98. Springer.
Benzm¨uller, C., Horacek, H., Kruijff-Korbayov´a, I., Pinkal,
M., Siekmann, J. H., and Wolska, M. (2007). Natural
language dialog with a tutor system for mathemati-
cal proofs. In Lu, R., Siekmann, J. H., and Ullrich,
C., editors, Cognitive Systems, Joint Chinese-German
Workshop, Shanghai, China, March 7-11, 2005, Re-
vised Selected Papers, volume 4429 of LNCS, pages
1–14. Springer.
Benzm¨uller, C., Horacek, H., Lesourd, H., Kruijff-
Korbajov´a, I., Schiller, M., and Wolska, M. (2006).
A corpus of tutorial dialogs on theorem proving; the
influence of the presentation of the study-material.
In Proceedings of International Conference on Lan-
guage Resources and Evaluation (LREC), Genoa,
Italy. ELDA.
Bundy, A., van Harmelen, F., Hesketh, J., and Smaill, A.
(1991). Experiments with proof plans for induction.
J. Autom. Reasoning, 7(3):303–324.
Gordon, M. J. C., Milner, R., and Wadsworth, C. P. (1979).
Edinburgh LCF, volume 78 of LNCS. Springer.
Hobbs, J. R. (1985). Granularity. In Proc. of the 9th Int.
Joint Conf. on Artificial Intelligence (IJCAI), pages
432–435.
Huang, X. (1994). Reconstruction proofs at the assertion
level. In Bundy, A., editor, Automated Deduction
- CADE-12, 12th International Conference on Auto-
mated Deduction, Nancy, France, June 26 - July 1,
1994, Proceedings, volume 814 of LNCS, pages 738–
752. Springer.
Keet, M. C. (2008). A Formal Theory of Granularity. PhD
thesis, Free University of Bozen-Bolzano, Italy.
Mccalla, G., Greer, J., Barrie, B., and Pospisil, P. (1992).
Granularity hierarchies. In Computers and Mathemat-
ics with Applications: Special Issue on Semantic Net-
works, pages 363–375.
McCune, W. (2003). Otter 3.3 reference manual.
www.mcs.anl.gov/AR/otter/otter33.pdf.
McMath, D., Rozenfeld, M., and Sommer, R. (2001). A
computer environment for writing ordinary mathemat-
ical proofs. In Proc. of LPAR 2001, volume 2250 of
LNCS, pages 507–516. Springer.
Melis, E. (1999). Knowledge-based proof planning. Artifi-
cial Intelligence, 115:494–498.
Schiller, M. and Benzm¨uller, C. (2009). Granularity-
adaptive proof presentation. In 14th Int. Conference
on Artificial Intelligence in Education (AIED). Sub-
mitted to The 14th International Conference on Artifi-
cial Intelligence in Education (AIED 2009).
Schiller, M., Dietrich, D., and Benzm¨uller, C. (2008). Proof
step analysis for proof tutoring – a learning approach
to granularity. Teaching Mathematics and Computer
Science. In print.
Sieg, W. (2007). The AProS project: Strategic thinking
& computational logic. Logic Journal of the IGPL,
15(4):359–368.
Siekmann, J., Benzm¨uller, C., and Autexier, S. (2006).
Computer supported mathematics with Omega. Jour-
nal of Applied Logic, 4(4):533–559.
Wack, B. (2005). Typage et deduction dans le calcul de
reecriture. PhD thesis, Univ. Henri Poincare Nancy 1.
CSEDU 2009 - International Conference on Computer Supported Education
354