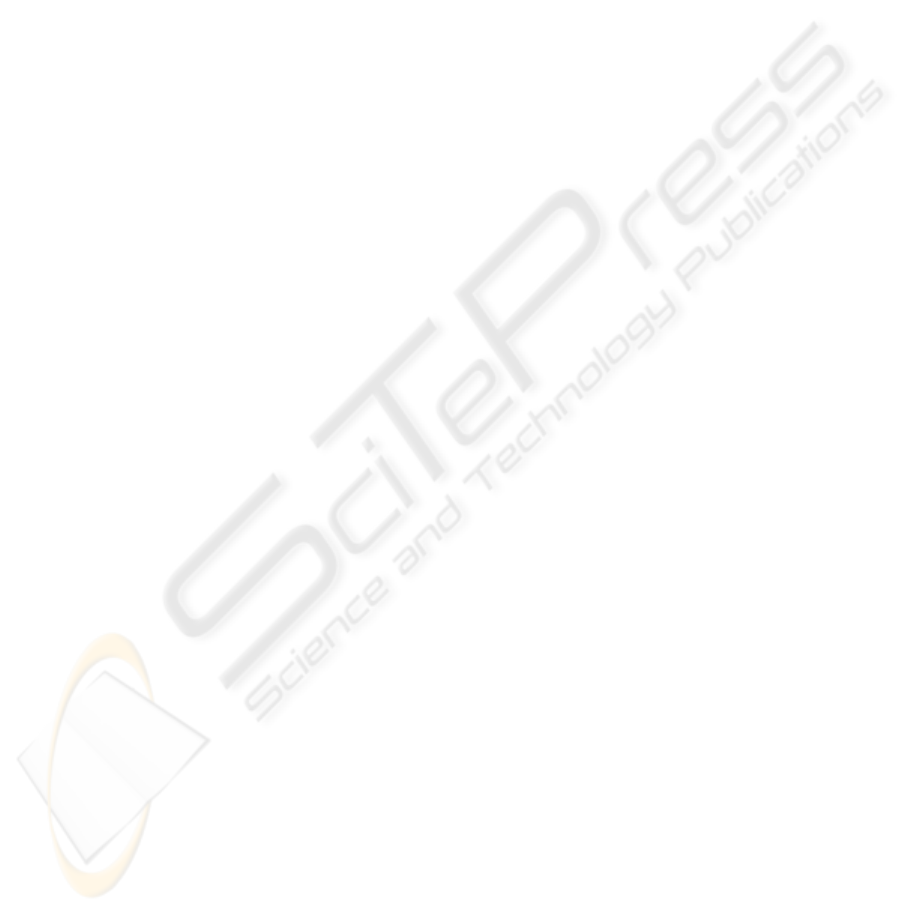
employing one of the six basic emotions can describe the emotion information to mass
of sentences precisely because human is more sensitive. Therefore previous models
mentioned above usually result in an emotion distortion problem, which means that the
results of the classification can not denote emotion states correctly. On the other hand,
Mishne experiment with emotion classification using cross-training and an ontology of
over 100 moods shows rather low accuracy even when the training corpus is very large
[7]. So we proposed that maybe we should consider this problem as similar with RGB
model. Thus at first we could complete the task of automatically classifying texts into
categories according to descriptions of their emotions, and then find the co-occurrence
patterns and their valence between labels, and finally synthesize those labels assigned to
one text to a more detailed and precise one described in Chinese, depending on the rules
learned from the previous step. In this paper, we utilized multi-label classification that
has been applied on topic detection and some other domains, to perform experiments as
the first single step of the work we focus on.
The remaining parts of this paper are organized as follows. Section 2 provides a
brief introduction of the blog emotion corpus we used. Section 3 describes the model
of our method, including the representation of the training samples, and the approach
to multi-label classification we employed. In Section 4 we present the results of our ex-
periments, compared using different evaluation measures. Finally, Section 5 describes
with a discussion and Section 6 concludes on this investigation and presents the possible
directions for the future work.
2 A Blog Emotion Corpus
With the increase in the web’s accessibility in the last years, the amount of weblogs has
risen dramatically and the so-called blogsphere attracts new research interests.
In this paper, our study is based on a collection of blog posts from various Chinese
blog communities, because these sources for modeling are recognized as more private,
honest, and polemic than opinions voiced in other style [4]. Emotion recognition models
proposedbefore were usually implemented on a roughly annotated corpus, so ambiguity
would be caused by the emotion corpus itself. Some corpora with which the previousre-
lated work experimented are actually emotionless. Lee et al. reported a high accuracy of
emotion recognition, but 73.17% of their corpus are neutral [8], and thus they obtained
a baseline system with the accuracy of 73.17%, which can be achieved easy by simply
marking the neutral texts. Our current corpus Ren-CECps (Chinese Emotion Corpus of
RenLab) 1.0 is manually annotated as a sentence level one consists of 198 documents,
5608 sentences, and 135,606 Chinese characters. Annotators worked in triples on the
same texts. They have been trained and work independently in order to avoid any anno-
tation bias and get a true understanding of the task difficulty. The goal of our annotation
project is to annotate a corpus of approximately 1000 blog posts, and the details of the
corpus would be published after completing it. Comparing the six basic categories of
emotion, each annotator marks the sentences with one or more of eight emotions listed
as follow: Anger, Anxiety, Expect, Hate, Joy, Love, Sorrow and Surprise. In order to
find the rules howeach emotion keyword or phrase correspondto the labels in our future
work, the image value of every emotion term attributed to every emotion label is also
104