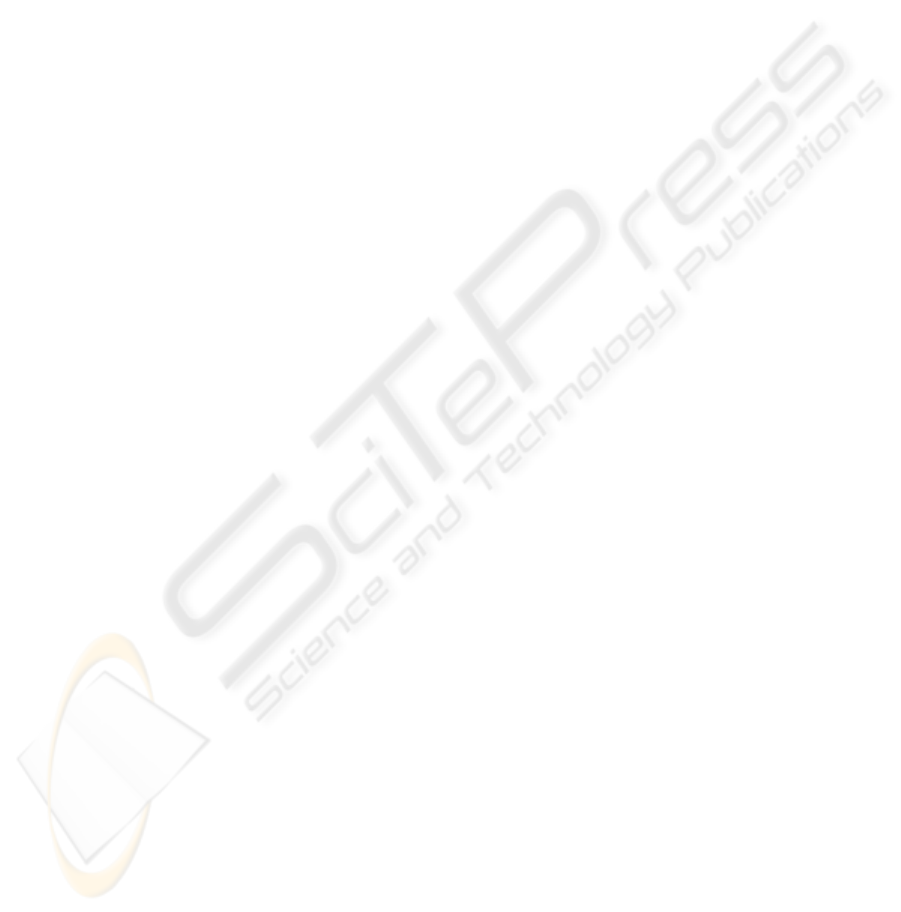
ON THE STATE–SPACE REALIZATION OF VECTOR
AUTOREGRESSIVE STRUCTURES
An Assessment
Vasilis K. Dertimanis, Dimitris V. Koulocheris
Vehicles Laboratory, National Technical University of Athens, Iroon Politechniou 9, 157 80, Athens, Greece
Keywords:
Vector autoregressive, Time–series, State–space, Green function, Covariance matrix, Dispersion analysis, Es-
timation.
Abstract:
This study explores the interconnection between vector autoregressive (VAR) structures and state–space mod-
els and results in a compact framework for the representation of multivariate time–series, as well as the esti-
mation of structural information. The corresponding methodology that is developed, applies the fact that every
VAR process of order n may be described by an equivalent (non–unique) VAR model of first order, which is
identical to a state–space realization. The latter uncovers many ”hidden” information of the initial model, it
is more easy to manipulate and maintains significant second moments’ information that can be reflected back
to the original structure with no effort. The performance of the proposed framework is validated using vector
time–series signatures from a structural system with two degrees of freedom, which retains a pair of closely
spaced vibration modes and has been reported in the relevant literature.
1 INTRODUCTION
The analysis of vector time–series, generally referring
to the determination of the dynamics that govern the
performance of a system under unobservable excita-
tions, has been a subject of constant development for
more than two decades, as part of the broader system
identification framework. Relative applications are
extended from econometrics (Clements and Henry,
1998; L¨utkepohl, 2005), to dynamics (Ljung, 1999;
Koulocheris et al., 2008), vibration (Papakos and Fas-
sois, 2003), modal analysis (Huang, 2001) and fault
diagnosis (Dertimanis, 2006).
The study of vector time–series can be assessed
from a variety of viewpoints, with respect to the ap-
plication of interest. These include simulation, pre-
diction and extraction of structural information. Yet,
while in the first two areas the interrelation of the cor-
responding time–series structures, such as the VAR
one (or the VARX and the VARMAX, under the
availability of input information), to equivalent state–
space models has been studied extensively (Hannan,
1976; Brockwell and Davis, 2002; L¨utkepohl, 2005),
not much have been done in the third (Lardies, 2008),
from where it appears that state–space realizations
may provide significant advantages, regarding struc-
tural estimation, with respect to other approaches (He
and Fu, 2001).
This paper attempts to provide a unified frame-
work for the representation of vector time–series, by
means of VAR structures and their corresponding
state–space realizations. Based on the fact that ev-
ery VAR structure of order n (referred to from now on
as VAR(n) structure) can be expressed as an equiva-
lent (and non–unique) VAR(1) one, a corresponding
state–space model is developed. This specific model
qualifies, over other possible realizations, for having
a transition matrix that coincides with the VAR(n)
polynomial matrix. It turns out that the spectrum of
this transition matrix has all the structural information
about the system that generates the time–series ”hid-
den” in its spectrum. Consequently, by taking advan-
tage standard results of matrix algebra, closed form
expressions for the Green function and the covariance
matrix are derived. The latter, unlike other estimation
schemes, such as the Burg and the forward–backward
methods (Brockwell and Davis, 2002), is by definition
closely related to the energy distribution of the vec-
tor time–series. Thus, the corresponding expression
that is assessed, quantifies the impact of each specific
structural mode in the total energy of the system, a
technique that has been recorded in the literature as
20
K. Dertimanis V. and V. Koulocheris D. (2009).
ON THE STATE–SPACE REALIZATION OF VECTOR AUTOREGRESSIVE STRUCTURES: AN ASSESSMENT.
In Proceedings of the 6th International Conference on Informatics in Control, Automation and Robotics - Signal Processing, Systems Modeling and
Control, pages 20-27
DOI: 10.5220/0002188100200027
Copyright
c
SciTePress