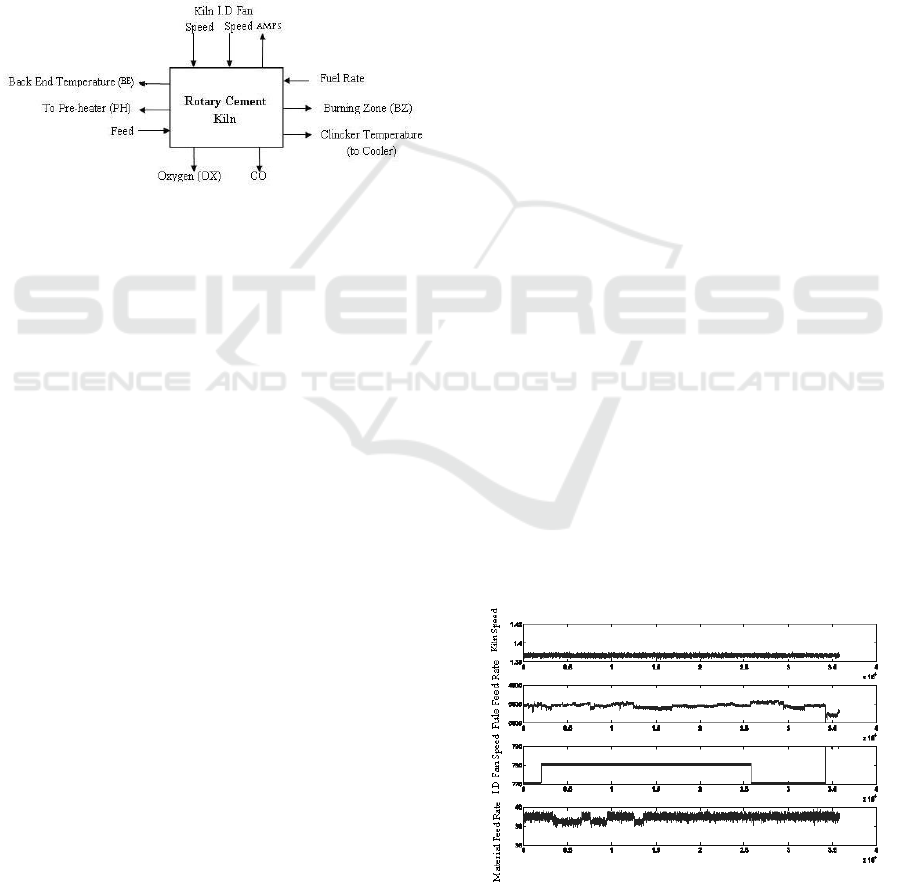
kiln speed
Fuel Flow Rate
ID. Fan speed
Raw Material Flow Rate
Also the output variables according to the
operator’s experiences are as following:
back end temperature
Remained (unused) oxygen, O
2
CO content of outlet gases from the kiln
Kiln DC motor current
Preheater temperature
Cooler temperature
Based on these variables the rotary kiln is a 4-
inputs 6-outputs plant as shown in figure 2.
Figure 2: Block diagram of the kiln based on input-output.
These variables are so important and selected
with fundament of expert operators, such as with
these input, the kiln can be controlled. The burning
zone temperature is not only one of the most
important kiln control variables but also the most
difficult one to monitor (Peray, 1986). Despite the
fact that burning zone condition in modern kilns are
shown as temperature profile that used for manual
controllers.
2 CONDITION OF DATA
GATHERING
There are three important factors in modelling based
on the identification techniques:
Useful and valid data
A perfect and useful model
Strong method to adjust the model
Input and outputs must be selected such that
input change affects output variables. Also the
recognition of process behavior will be much
simpler if input-output data is reach, i.e. it consists
different operating points and frequency contents.
However, system identification based on input
output data does not introduce a physical model with
exact structure but it does a model that fits the data.
Therefore, selection of a proper model is important.
Also, the obtained data should have the process
information to be used for identification.
An important point concerning data gathering is
that to be careful that the disturbances and
unexpected events such as creation of coating and
coating fall in the kiln and do not change the system
behaviour. The white cement rotary kiln
identification is passive process, meaning that we
can only observe the plant variables under a given
circumstance and it is technically impossible to
introduce extra excitation on these systems. The data
from these systems may not be informative enough.
This can make the identification of the system
difficult (Zhu, 2001). Therefore it is not possible to
expect from the presented model to have the same
behaviour with the real system in an abnormal
condition unless these conditions are occurred a few
times during data gathering.
For this reason, data gathered during a period of
18 hours for several times. Finally, the best
conditioned data were obtained for the rotary kiln in
2008-05-07. Figure 3 shows the input variables. The
output variables are shown in figure 4.
3 DATA PRETREATMENT
After collecting perfect data from rotary kiln, the
data will not be used directly for identification
process. One of its reasons is high frequency noises
and spikes on the main signals. Sometimes
immeasurable disturbances occur and take the
system out of its linear range. Changing Operation
point causes entering nonlinear effects in output
data. To solve the problem of high frequency noises
and some of these problems, it is tried to use some
pre-processing methods mentioned in identification
references to reach a perfect model of process
(Ljung, 1999; Nelles, 2001) . For considering rest of
them, we tried to choose the model structure and
focus on its flexibilities.
Figure 3: Input data representation for white cement kiln
identification.
LINEAR IDENTIFICATION OF ROTARY WHITE CEMENT KILN
191